Empowering Investigative Journalism with Graph-based Heterogeneous Data Management.
IEEE Data Eng. Bull.(2021)
摘要
Investigative Journalism (IJ, in short) is staple of modern, democratic societies. IJ often necessitates working with large, dynamic sets of heterogeneous, schema-less data sources, which can be structured, semi-structured, or textual, limiting the applicability of classical data integration approaches. In prior work, we have developed ConnectionLens, a system capable of integrating such sources into a single heterogeneous graph, leveraging Information Extraction (IE) techniques; users can then query the graph by means of keywords, and explore query results and their neighborhood using an interactive GUI. Our keyword search problem is complicated by the graph heterogeneity, and by the lack of a result score function that would allow to prune some of the search space. In this work, we describe an actual IJ application studying conflicts of interest in the biomedical domain, and we show how ConnectionLens supports it. Then, we present novel techniques addressing the scalability challenges raised by this application: one allows to reduce the significant IE costs while building the graph, while the other is a novel, parallel, in-memory keyword search engine, which achieves orders of magnitude speed-up over our previous engine. Our experimental study on the real-world IJ application data confirms the benefits of our contributions.
更多查看译文
关键词
investigative journalism,heterogeneous data
AI 理解论文
溯源树
样例
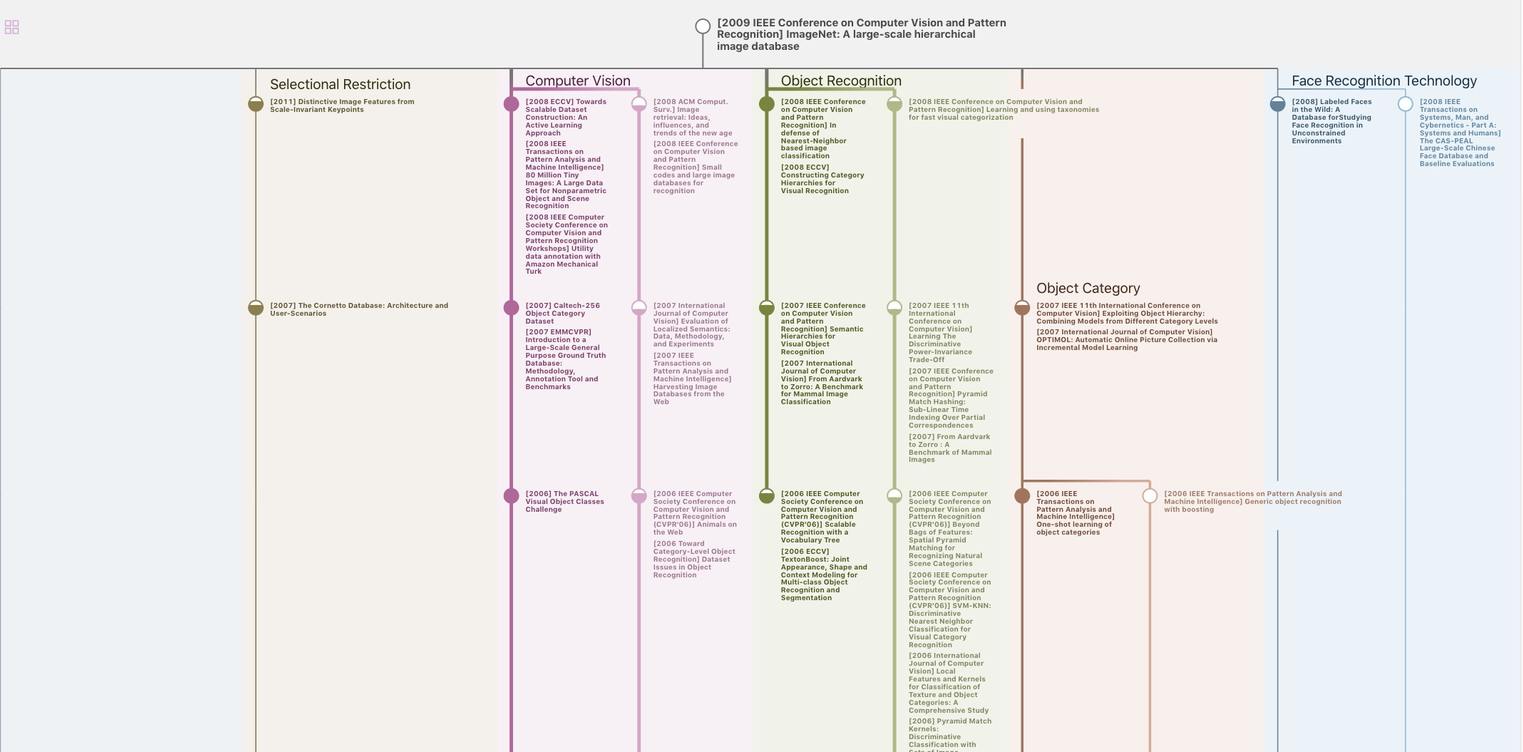
生成溯源树,研究论文发展脉络
Chat Paper
正在生成论文摘要