Functional Optimal Transport: Mapping Estimation and Domain Adaptation for Functional data
arxiv(2021)
摘要
Optimal transport (OT) has generated much recent interest by its capability of finding mappings that transport mass from one distribution to another, and found useful roles in machine learning tasks such as unsupervised learning, domain adaptation and transfer learning. On the other hand, in many applications data are generated by complex mechanisms involving convoluted spaces of functions, curves and surfaces in high dimensions. Functional data analysis provides a useful framework of treatment for such domains. In this paper we introduce a novel formulation of optimal transport problem in functional spaces and develop an efficient learning algorithm for finding the stochastic map between functional domains. We apply our method to synthetic datasets and study the geometric properties of the transport map. Experiments on real-world datasets of robot arm trajectories and digit numbers further demonstrate the effectiveness of our method on applications of domain adaptation and generative modeling.
更多查看译文
关键词
functional data,transport,domain adaptation,mapping estimation
AI 理解论文
溯源树
样例
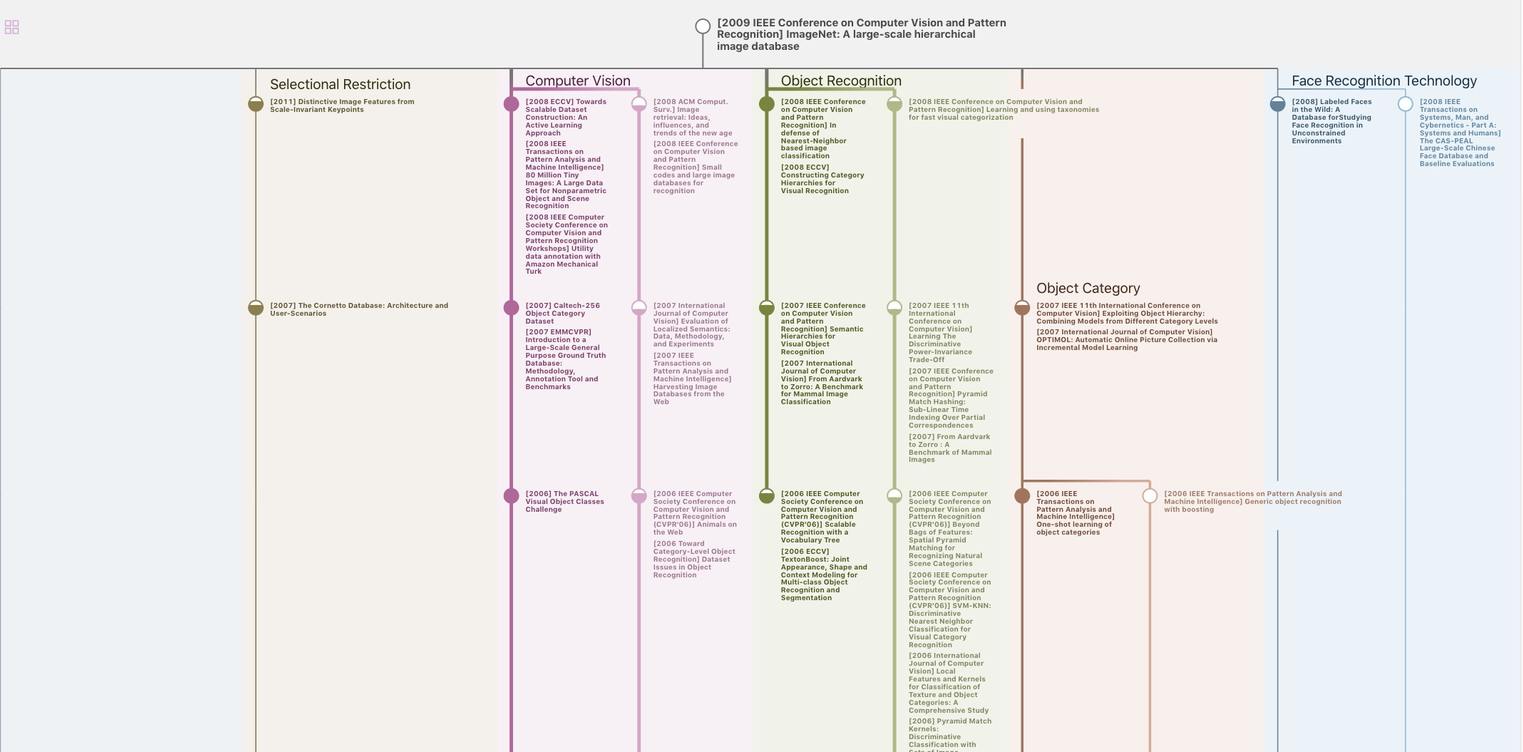
生成溯源树,研究论文发展脉络
Chat Paper
正在生成论文摘要