CMS-LSTM: Context-Embedding and Multi-Scale Spatiotemporal-Expression LSTM for Video Prediction
arxiv(2021)
摘要
Extracting variation and spatiotemporal features via limited frames remains as an unsolved and challenging problem in video prediction. Inherent uncertainty among consecutive frames exacerbates the difficulty in long-term prediction. To tackle the problem, we focus on capturing context correlations and multi-scale spatiotemporal flows, then propose CMS-LSTM by integrating two effective and lightweight blocks, namely Context-Embedding (CE) and Spatiotemporal-Expression (SE) block, into ConvLSTM backbone. CE block is designed for abundant context interactions, while SE block focuses on multi-scale spatiotemporal expression in hidden states. The newly introduced blocks also facilitate other spatiotemporal models (e.g., PredRNN, SA-ConvLSTM) to produce representative implicit features for video prediction. Qualitative and quantitative experiments demonstrate the effectiveness and flexibility of our proposed method. We use fewer parameters to reach markedly state-of-the-art results on Moving MNIST and TaxiBJ datasets in numbers of metrics. All source code is available at https://github.com/czh-98/CMS-LSTM.
更多查看译文
关键词
learning,context,cms-lstm,multi-scale
AI 理解论文
溯源树
样例
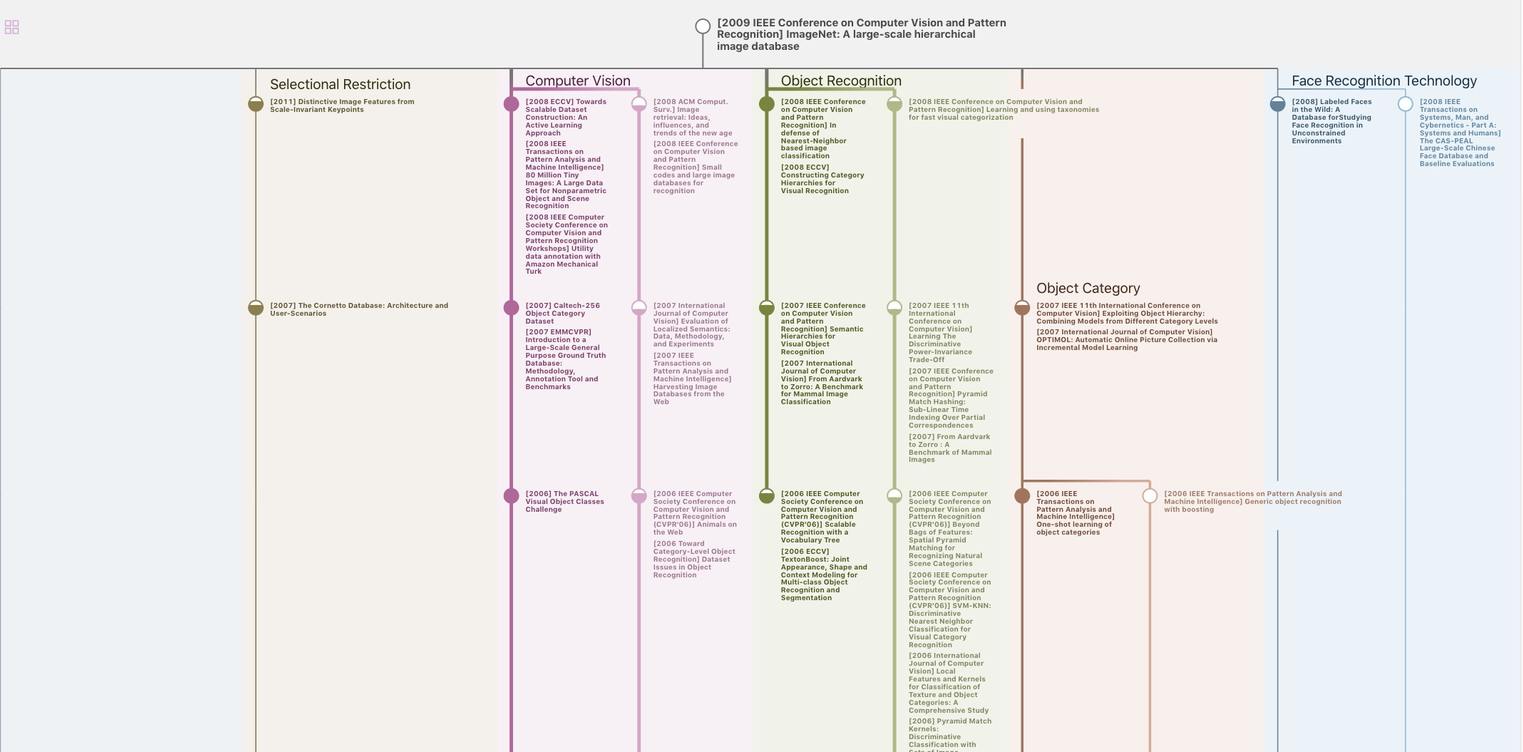
生成溯源树,研究论文发展脉络
Chat Paper
正在生成论文摘要