Sill-Net: Feature Augmentation with Separated Illumination Representation
arxiv(2022)
摘要
For visual object recognition tasks, the illumination variations can cause distinct changes in object appearance and thus confuse the deep neural network based recognition models. Especially for some rare illumination conditions, collecting sufficient training samples could be time-consuming and expensive. To solve this problem, in this paper we propose a novel neural network architecture called Separating-Illumination Network (Sill-Net). Sill-Net learns to separate illumination features from images, and then during training we augment training samples with these separated illumination features in the feature space. Experimental results demonstrate that our approach outperforms current state-of-the-art methods in several object classification benchmarks.
更多查看译文
关键词
feature augmentation,separated illumination
AI 理解论文
溯源树
样例
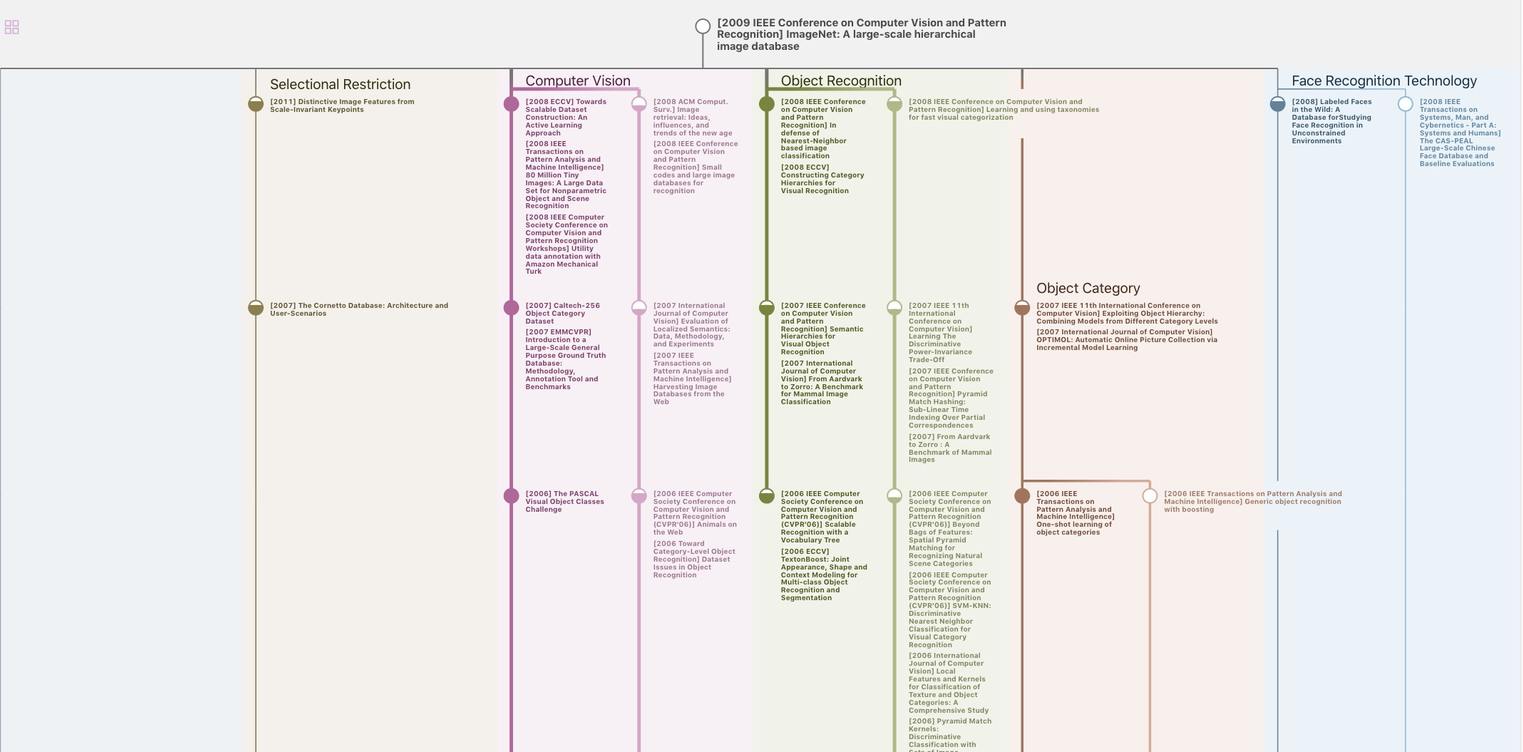
生成溯源树,研究论文发展脉络
Chat Paper
正在生成论文摘要