ChainCQG: Flow-Aware Conversational Question Generation
EACL(2021)
摘要
Conversational systems enable numerous valuable applications, and question-answering is an important component underlying many of these. However, conversational question-answering remains challenging due to the lack of realistic, domain-specific training data. Inspired by this bottleneck, we focus on conversational question generation as a means to generate synthetic conversations for training and evaluation purposes. We present a number of novel strategies to improve conversational flow and accommodate varying question types and overall fluidity. Specifically, we design ChainCQG as a two-stage architecture that learns question-answer representations across multiple dialogue turns using a flow propagation training strategy.ChainCQG significantly outperforms both answer-aware and answer-unaware SOTA baselines (e.g., up to 48% BLEU-1 improvement). Additionally, our model is able to generate different types of questions, with improved fluidity and coreference alignment.
更多查看译文
关键词
chaincqg,generation,flow-aware
AI 理解论文
溯源树
样例
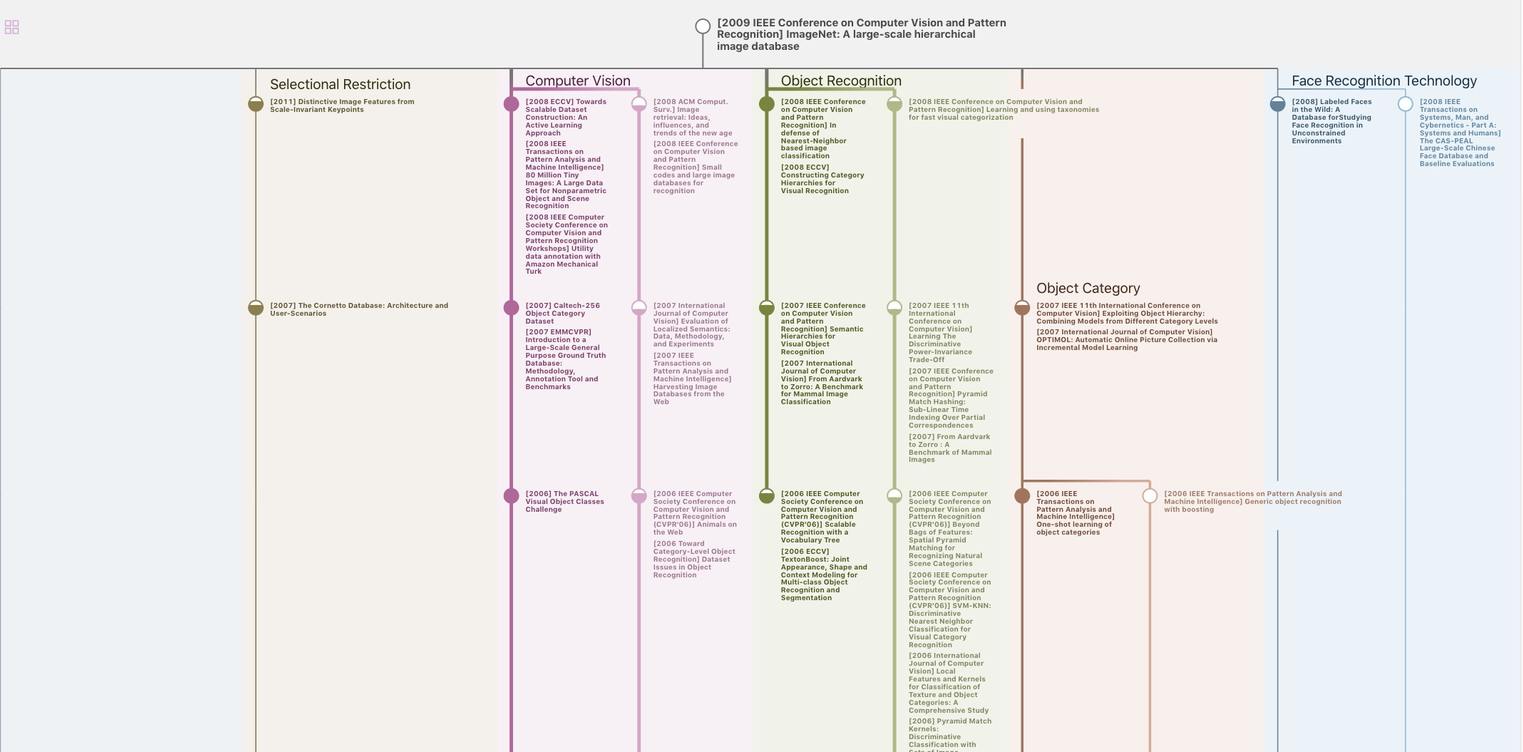
生成溯源树,研究论文发展脉络
Chat Paper
正在生成论文摘要