Content Learning With Structure-Aware Writing: A Graph-Infused Dual Conditional Variational Autoencoder For Automatic Storytelling
AAAI 2021(2021)
摘要
Recent automatic storytelling methods mainly rely on keyword planning or plot skeleton generation to model long-range dependencies and create consistent narrative texts. However, these approaches generate story plans or plots sequentially, leaving the non-sequential conception and structural design processes of human writers unexplored. To mimic human writers and exploit the fine-grained, intrinsic structural information of each story, we decompose automatic story generation into sub-problems of graph construction, graph generation, and graph-infused sequence generation. Specifically, we propose a graph-infused dual conditional variational autoencoder model to capture multi-level intra-story structures (i.e., graph) by continuous variational latent variables and generate consistent stories through dual-infusion of story structure planning and content learning. Experimental results on the ROCStories dataset and the CMU Movie Summary corpus confirm that our proposed model outperforms strong baselines in both human judges and widely-used automatic metrics.
更多查看译文
AI 理解论文
溯源树
样例
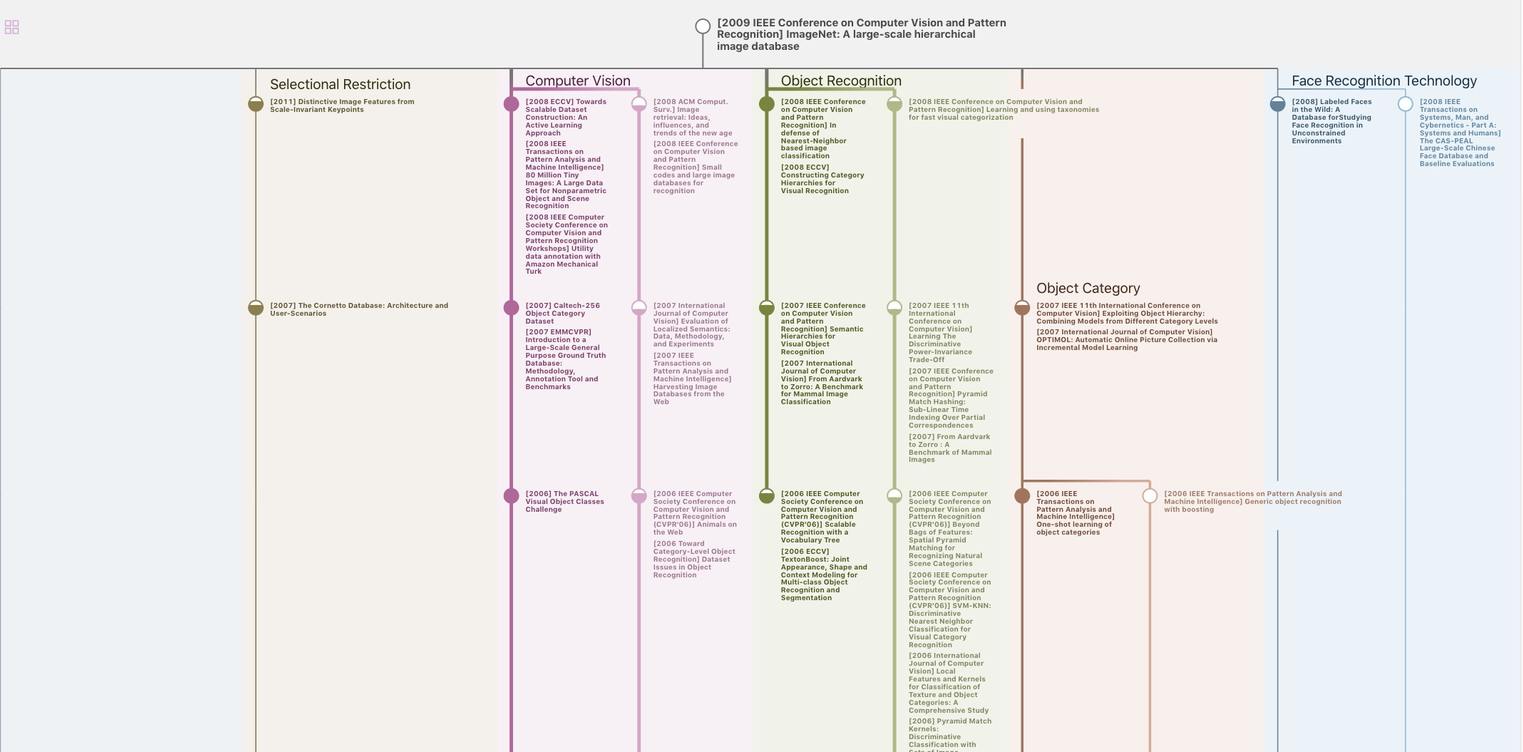
生成溯源树,研究论文发展脉络
Chat Paper
正在生成论文摘要