Frugal Optimization For Cost-Related Hyperparameters
THIRTY-FIFTH AAAI CONFERENCE ON ARTIFICIAL INTELLIGENCE, THIRTY-THIRD CONFERENCE ON INNOVATIVE APPLICATIONS OF ARTIFICIAL INTELLIGENCE AND THE ELEVENTH SYMPOSIUM ON EDUCATIONAL ADVANCES IN ARTIFICIAL INTELLIGENCE(2021)
摘要
The increasing demand for democratizing machine learning algorithms calls for hyperparameter optimization (HPO) solutions at low cost. Many machine learning algorithms have hyperparameters which can cause a large variation in the training cost. But this effect is largely ignored in existing HPO methods, which are incapable to properly control cost during the optimization process. To address this problem, we develop a new cost-frugal HPO solution. The core of our solution is a simple but new randomized direct-search method, for which we provide theoretical guarantees on the convergence rate and the total cost incurred to achieve convergence. We provide strong empirical results in comparison with state-of-the-art HPO methods on large AutoML benchmarks.
更多查看译文
关键词
frugal optimization,cost-related
AI 理解论文
溯源树
样例
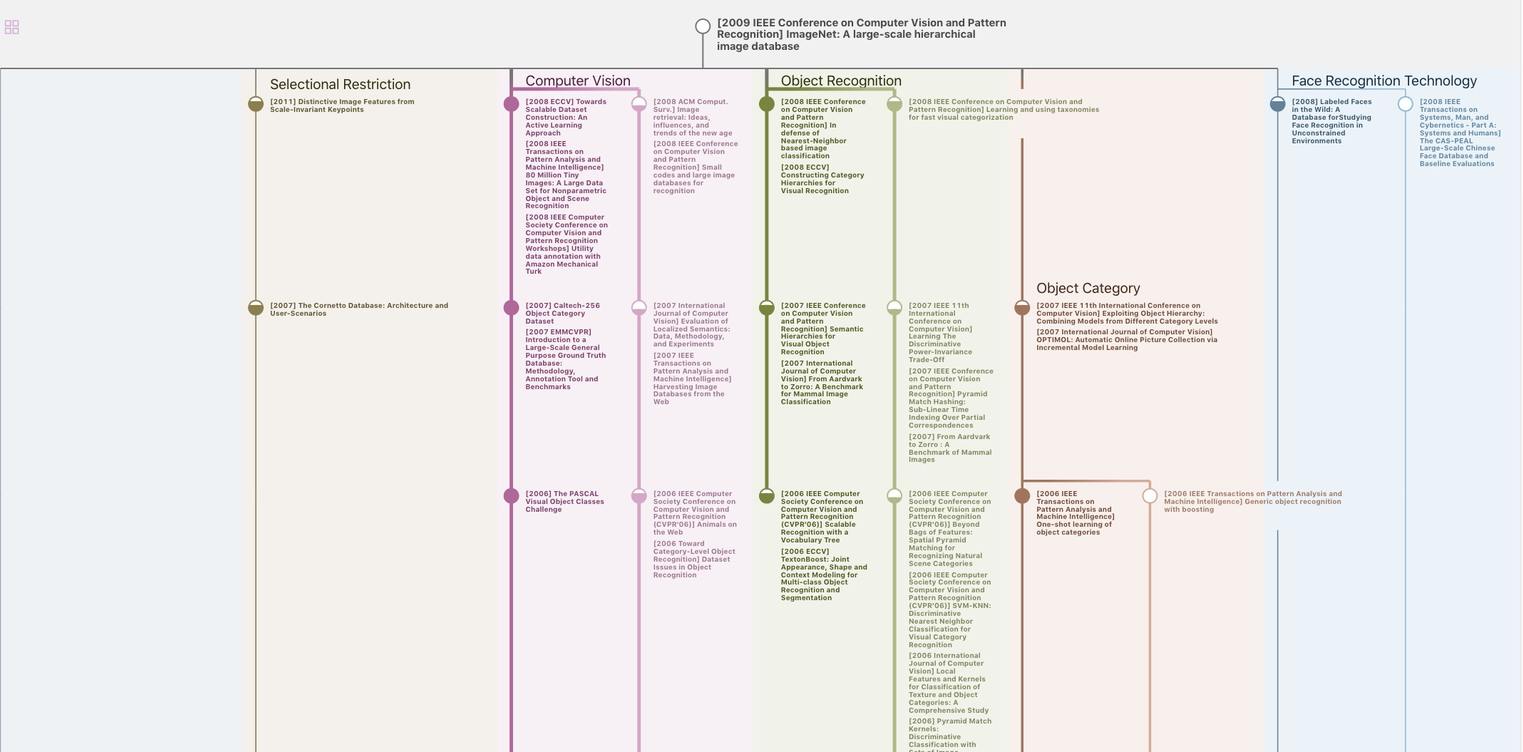
生成溯源树,研究论文发展脉络
Chat Paper
正在生成论文摘要