Denoising Distantly Supervised Named Entity Recognition via a Hypergeometric Probabilistic Model
AAAI 2021(2021)
摘要
Denoising is the essential step for distant supervision based named entity recognition. Previous denoising methods are mostly based on instance-level confidence statistics, which ignore the variety of the underlying noise distribution on different datasets and entity types. This makes them difficult to be adapted to high noise rate settings. In this paper, we propose Hypergeometric Learning (HGL), a denoising algorithm for distantly supervised NER that takes both noise distribution and instance-level confidence into consideration. Specifically, during neural network training, we naturally model the noise samples in each batch following a hypergeometric distribution parameterized by the noise-rate. Then each instance in the batch is regarded as either correct or noisy one according to its label confidence derived from previous training step, as well as the noise distribution in this sampled batch. Experiments show that HGL can effectively denoise the weakly-labeled data retrieved from distant supervision, and therefore results in significant improvements on the trained models.
更多查看译文
关键词
supervised named entity recognition,hypergeometric probabilistic model
AI 理解论文
溯源树
样例
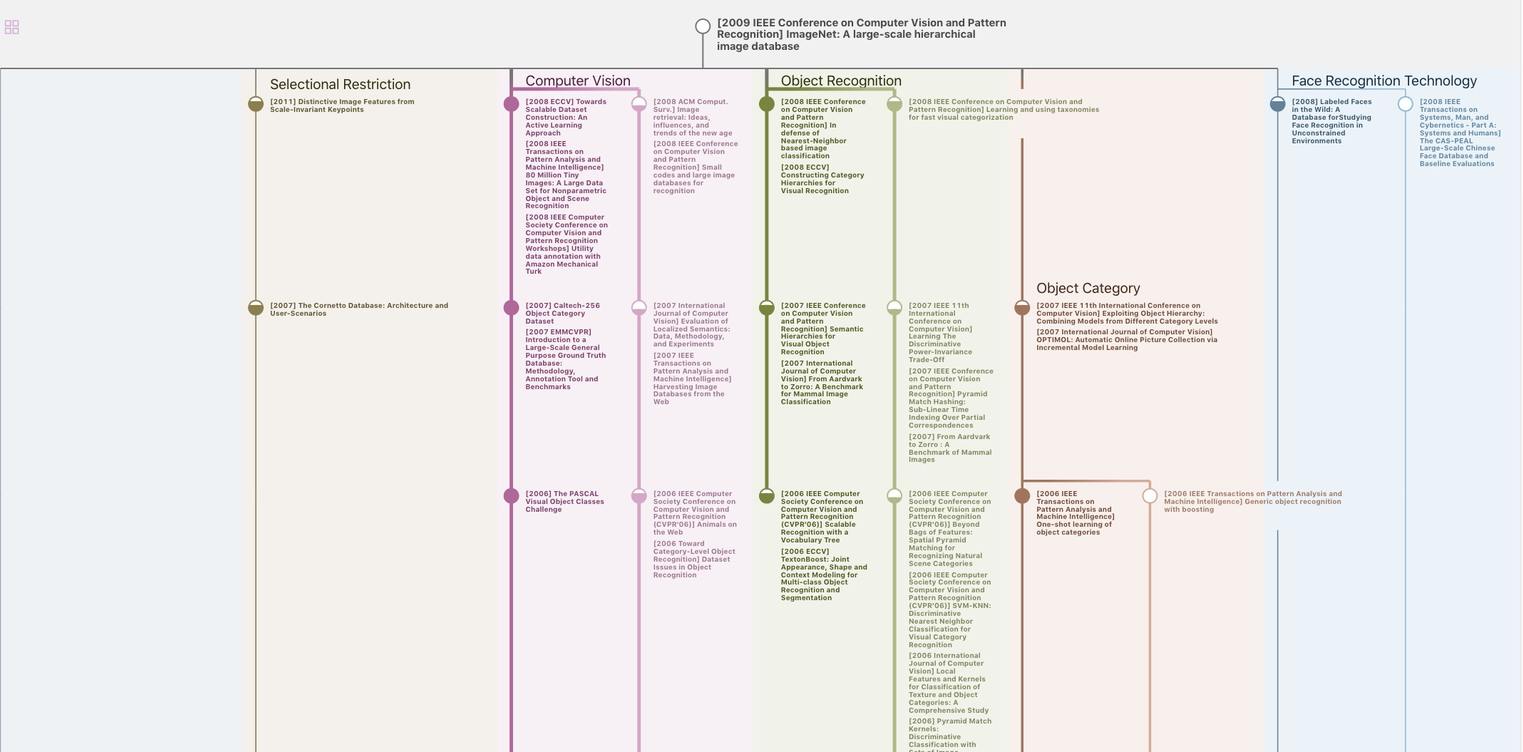
生成溯源树,研究论文发展脉络
Chat Paper
正在生成论文摘要