Stochastic Bandits With Graph Feedback In Non-Stationary Environments
THIRTY-FIFTH AAAI CONFERENCE ON ARTIFICIAL INTELLIGENCE, THIRTY-THIRD CONFERENCE ON INNOVATIVE APPLICATIONS OF ARTIFICIAL INTELLIGENCE AND THE ELEVENTH SYMPOSIUM ON EDUCATIONAL ADVANCES IN ARTIFICIAL INTELLIGENCE(2021)
摘要
We study a variant of stochastic bandits where the feedback model is specified by a graph. In this setting, after playing an arm, one can observe rewards of not only the played arm but also other arms that are adjacent to the played arm in the graph. Most of the existing work assumes the reward distributions are stationary over time, which, however, is often violated in common scenarios such as recommendation systems and online advertising. To address this limitation, we study stochastic bandits with graph feedback in non-stationary environments and propose algorithms with graph-dependent dynamic regret bounds. When the number of reward distribution changes L is known in advance, one of our algorithms achieves an (O) over tilde(root alpha LT) dynamic regret bound. We also develop an adaptive algorithm that can adapt to unknown L and attain an (O) over tilde(root theta LT) dynamic regret. Here, alpha and theta are some graph-dependent quantities and T is the time horizon.
更多查看译文
AI 理解论文
溯源树
样例
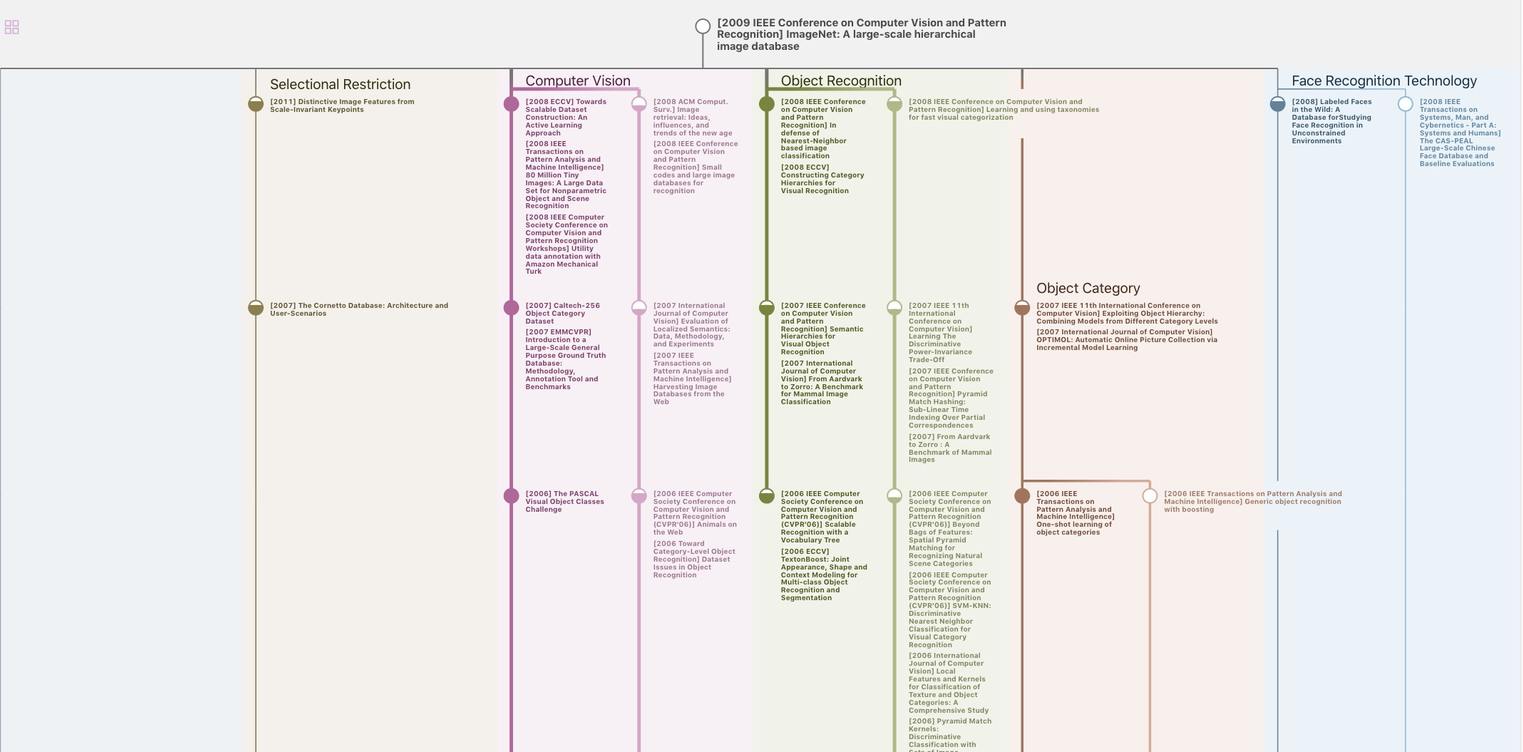
生成溯源树,研究论文发展脉络
Chat Paper
正在生成论文摘要