Deep Event Stereo Leveraged By Event-To-Image Translation
THIRTY-FIFTH AAAI CONFERENCE ON ARTIFICIAL INTELLIGENCE, THIRTY-THIRD CONFERENCE ON INNOVATIVE APPLICATIONS OF ARTIFICIAL INTELLIGENCE AND THE ELEVENTH SYMPOSIUM ON EDUCATIONAL ADVANCES IN ARTIFICIAL INTELLIGENCE(2021)
摘要
Depth estimation in real-world applications requires precise responses to fast motion and challenging lighting conditions. Event cameras use bio-inspired event-driven sensors that provide instantaneous and asynchronous information of pixel-level log intensity changes, which makes them suitable for depth estimation in such challenging conditions. However, as the event cameras primarily provide asynchronous and spatially sparse event data, it is hard to provide accurate dense disparity maps in stereo event camera setups - especially in estimating disparities on local structures or edges. In this study, we develop a deep event stereo network that reconstructs spatial image features from embedded event data and leverages the event features using the reconstructed image features to compute dense disparity maps. To this end, we propose a novel image reconstruction sub-network with a cross-semantic attention mechanism. A feature aggregation sub-network is also developed for accurate disparity estimation, which modulates the event features with the reconstructed image features by a stacked dilated spatially-adaptive denormalization mechanism. Experimental results reveal that our method outperforms the state-of-the-art methods by significant margins both in quantitative and qualitative measures.
更多查看译文
AI 理解论文
溯源树
样例
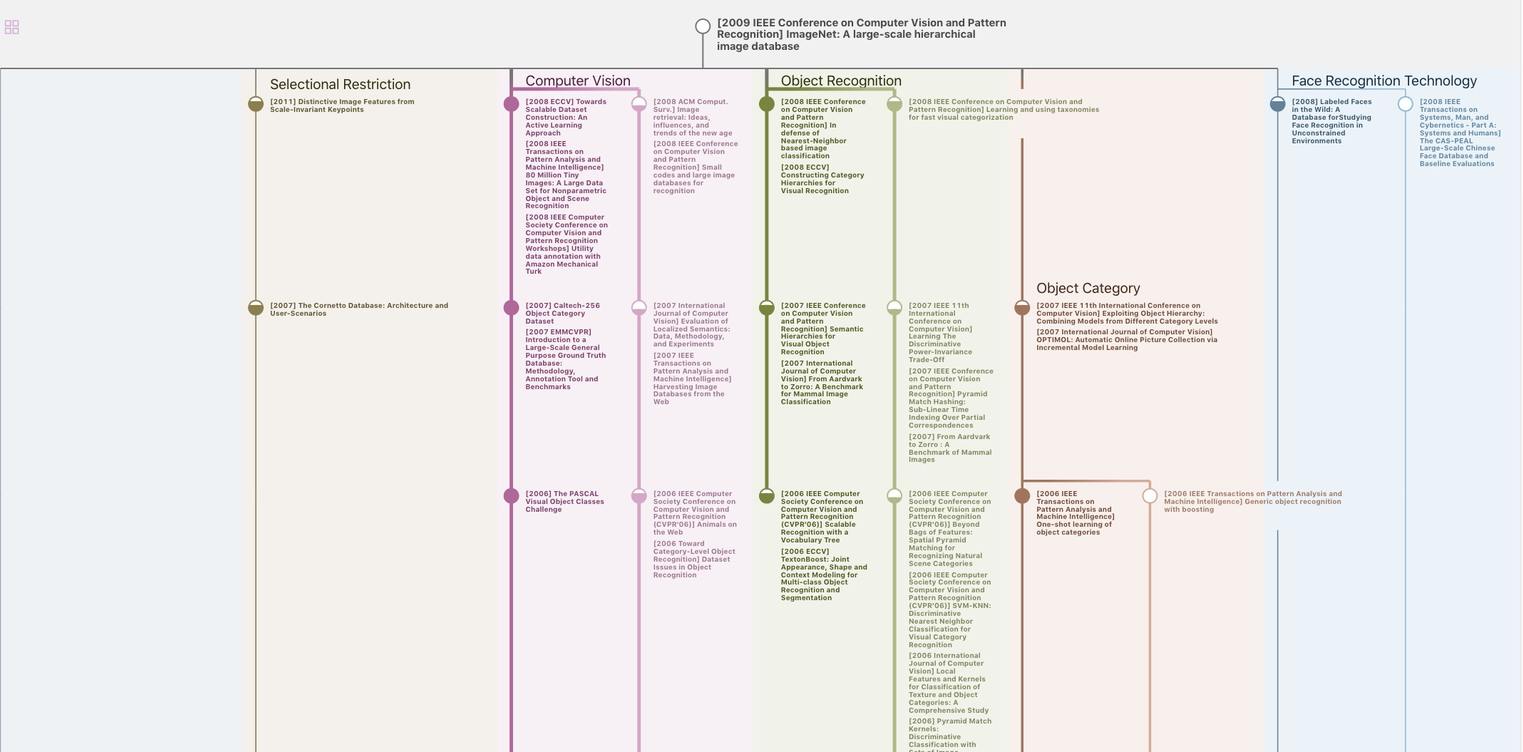
生成溯源树,研究论文发展脉络
Chat Paper
正在生成论文摘要