Time Series Anomaly Detection With Multiresolution Ensemble Decoding
THIRTY-FIFTH AAAI CONFERENCE ON ARTIFICIAL INTELLIGENCE, THIRTY-THIRD CONFERENCE ON INNOVATIVE APPLICATIONS OF ARTIFICIAL INTELLIGENCE AND THE ELEVENTH SYMPOSIUM ON EDUCATIONAL ADVANCES IN ARTIFICIAL INTELLIGENCE(2021)
摘要
Recurrent autoencoder is a popular model for time series anomaly detection, in which outliers or abnormal segments are identified by their high reconstruction errors. However, existing recurrent autoencoders can easily suffer from over-fitting and error accumulation due to sequential decoding. In this paper, we propose a simple yet efficient recurrent network ensemble called Recurrent Autoencoder with Multiresolution Ensemble Decoding (RAMED). By using decoders with different decoding lengths and a new coarse-to-fine fusion mechanism, lower-resolution information can help long-range decoding for decoders with higher-resolution outputs. A multiresolution shape-forcing loss is further introduced to encourage decoders' outputs at multiple resolutions to match the input's global temporal shape. Finally, the output from the decoder with the highest resolution is used to obtain an anomaly score at each time step. Extensive empirical studies on real-world benchmark data sets demonstrate that the proposed RAMED model outperforms recent strong baselines on time series anomaly detection.
更多查看译文
AI 理解论文
溯源树
样例
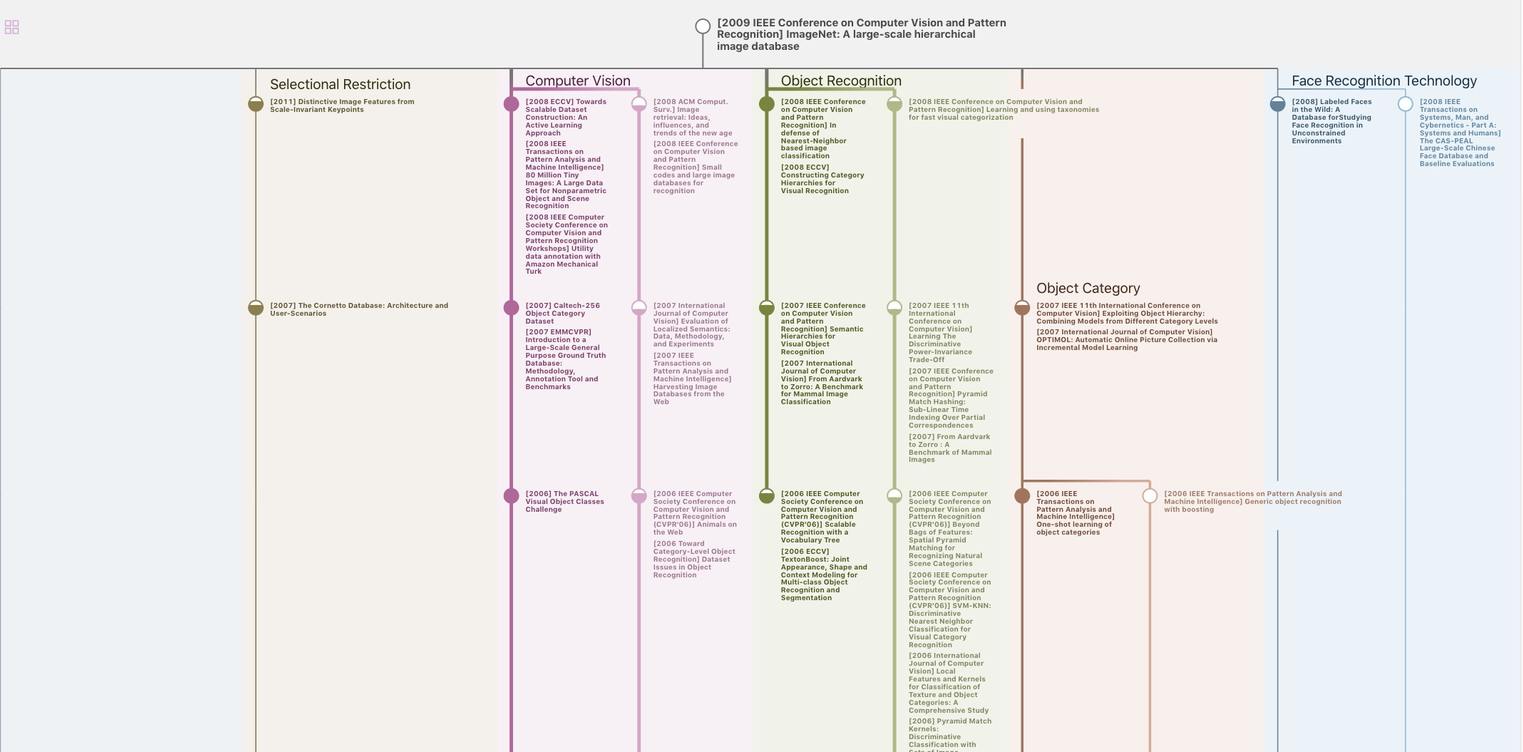
生成溯源树,研究论文发展脉络
Chat Paper
正在生成论文摘要