Towards Enabling Learnware To Handle Unseen Jobs
THIRTY-FIFTH AAAI CONFERENCE ON ARTIFICIAL INTELLIGENCE, THIRTY-THIRD CONFERENCE ON INNOVATIVE APPLICATIONS OF ARTIFICIAL INTELLIGENCE AND THE ELEVENTH SYMPOSIUM ON EDUCATIONAL ADVANCES IN ARTIFICIAL INTELLIGENCE(2021)
摘要
The learnware paradigm attempts to change the current style of machine learning deployment, i.e., user builds her own machine learning application almost from scratch, to a style where the previous efforts of other users can be reused, given a publicly available pool of machine learning models constructed by previous users for various tasks. Each learnware is a high-quality pre-trained model associated with its specification. Although there are many models in the learnware market, only a few, even none, may be potentially helpful for the current job. Therefore, how to identify and deploy useful models becomes one of the main concerns, which particularly matters when the user's job involves certain unseen parts not covered by the current learnware market. It becomes more challenging because, due to the privacy consideration, the raw data used for training models in the learnware market are inaccessible. In this paper, we develop a novel scheme that works can effectively reuse the learnwares even when the user's job involves unseen parts. Despite the raw training data are inaccessible, our approach can provably identify samples from the unseen parts while assigning the rest to proper models in the market for predicting under a certain condition. Empirical studies also validate the efficacy of our approach.
更多查看译文
AI 理解论文
溯源树
样例
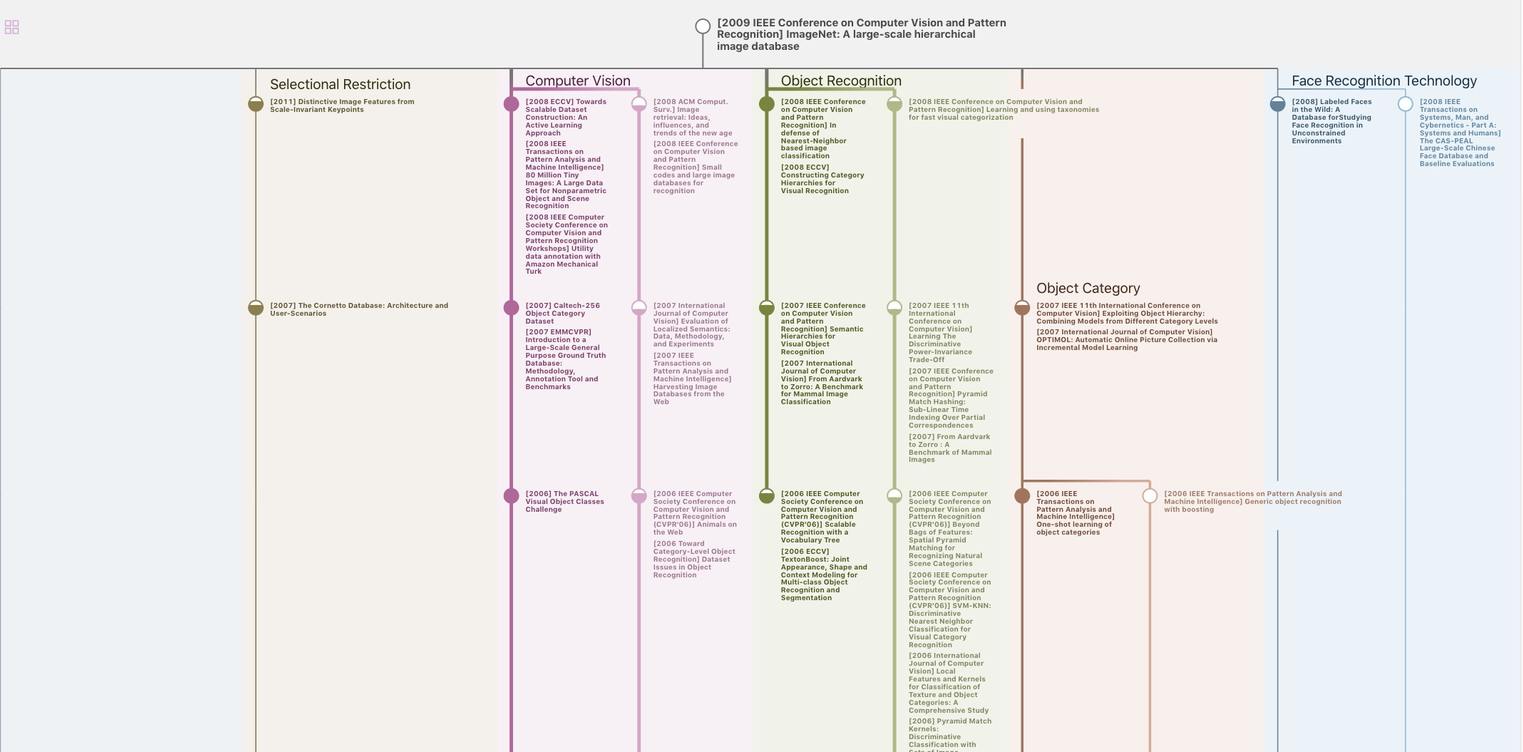
生成溯源树,研究论文发展脉络
Chat Paper
正在生成论文摘要