Empirical Regularization For Synthetic Sentence Pairs In Unsupervised Neural Machine Translation
THIRTY-FIFTH AAAI CONFERENCE ON ARTIFICIAL INTELLIGENCE, THIRTY-THIRD CONFERENCE ON INNOVATIVE APPLICATIONS OF ARTIFICIAL INTELLIGENCE AND THE ELEVENTH SYMPOSIUM ON EDUCATIONAL ADVANCES IN ARTIFICIAL INTELLIGENCE(2021)
摘要
UNMT tackles translation on monolingual corpora in two required languages. Since there is no explicitly cross-lingual signal, pre-training and synthetic sentence pairs are significant to the success of UNMT. In this work, we empirically study the core training procedure of UNMT to analyze the synthetic sentence pairs obtained from back-translation. We introduce new losses to UNMT to regularize the synthetic sentence pairs by training the UNMT objective and the regularization objective jointly. Our comprehensive experiments support that our method can generally improve the performance of currently successful models on three similar pairs {French, German, Romanian} <-> English and one dissimilar pair Russian <-> English with acceptably additional cost.
更多查看译文
AI 理解论文
溯源树
样例
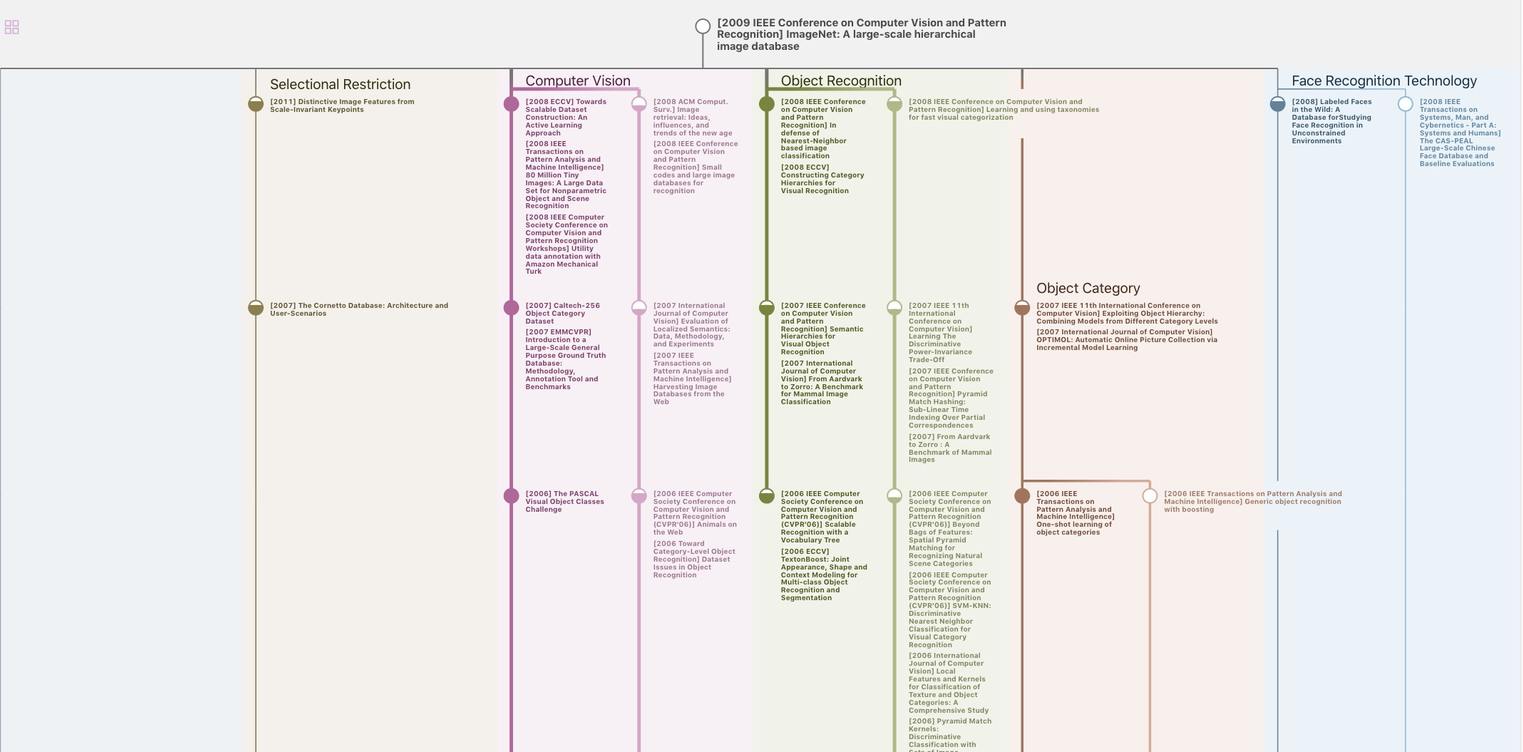
生成溯源树,研究论文发展脉络
Chat Paper
正在生成论文摘要