SelfNorm and CrossNorm for Out-of-Distribution Robustness
arxiv(2021)
摘要
Normalization techniques are crucial in stabilizing and accelerating the training of deep neural networks. However, they are mainly designed for the independent and identically distributed (IID) data, not satisfying many real-world out-of-distribution (OOD) situations. Unlike most previous works, this paper presents two normalization methods, SelfNorm and CrossNorm, to promote OOD generalization. SelfNorm uses attention to recalibrate statistics (channel-wise mean and variance), while CrossNorm exchanges the statistics between feature maps. SelfNorm and CrossNorm can complement each other in OOD generalization, though exploring different directions in statistics usage. Extensive experiments on different domains (vision and language), tasks (classification and segmentation), and settings (supervised and semi-supervised) show their effectiveness.
更多查看译文
关键词
Training,Bridges,Computer vision,Codes,Robustness,Task analysis
AI 理解论文
溯源树
样例
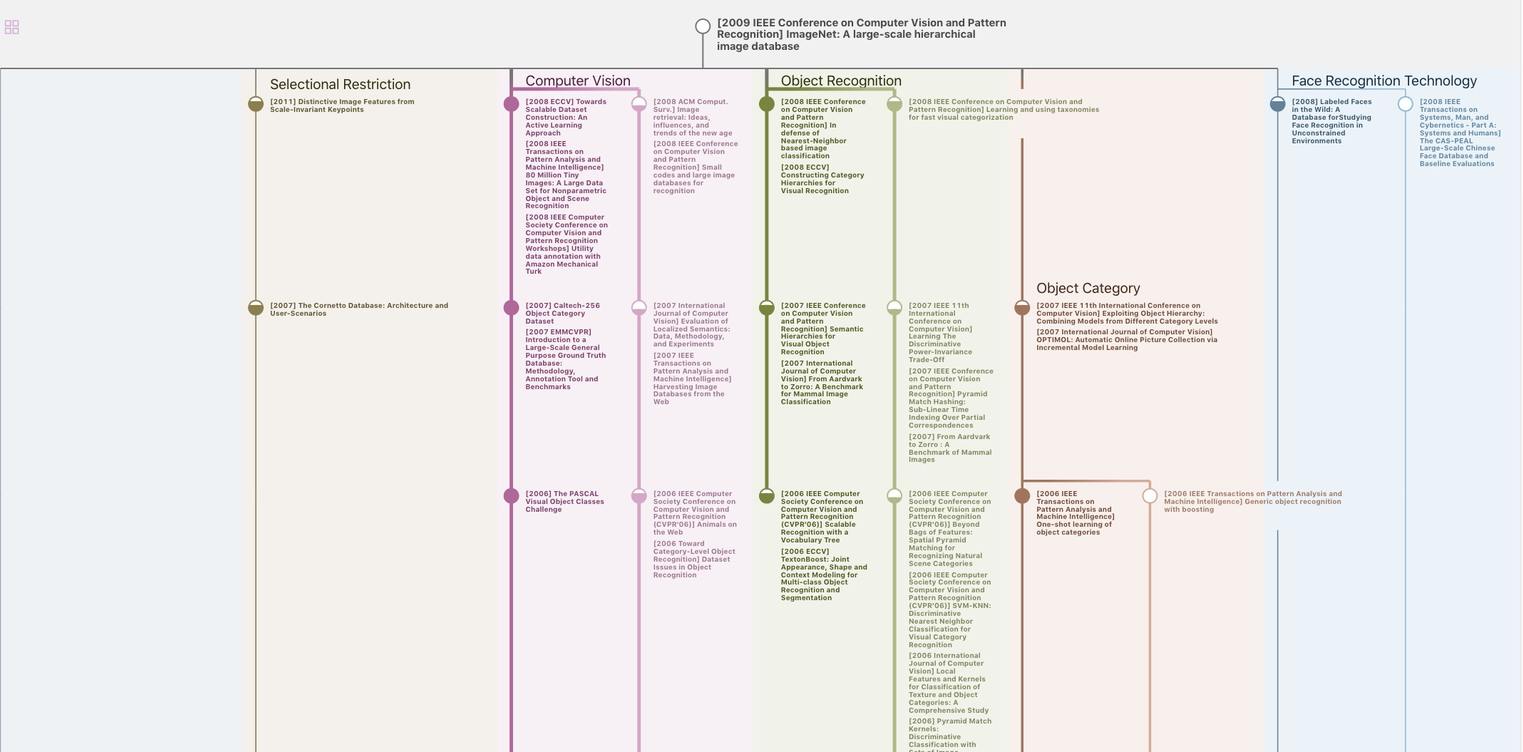
生成溯源树,研究论文发展脉络
Chat Paper
正在生成论文摘要