TricycleGAN: Unsupervised Image Synthesis and Segmentation Based on Shape Priors
arxiv(2021)
摘要
Medical image segmentation is routinely performed to isolate regions of interest, such as organs and lesions. Currently, deep learning is the state of the art for automatic segmentation, but is usually limited by the need for supervised training with large datasets that have been manually segmented by trained clinicians. The goal of semi-superised and unsupervised image segmentation is to greatly reduce, or even eliminate, the need for training data and therefore to minimze the burden on clinicians when training segmentation models. To this end we introduce a novel network architecture for capable of unsupervised and semi-supervised image segmentation called TricycleGAN. This approach uses three generative models to learn translations between medical images and segmentation maps using edge maps as an intermediate step. Distinct from other approaches based on generative networks, TricycleGAN relies on shape priors rather than colour and texture priors. As such, it is particularly well-suited for several domains of medical imaging, such as ultrasound imaging, where commonly used visual cues may be absent. We present experiments with TricycleGAN on a clinical dataset of kidney ultrasound images and the benchmark ISIC 2018 skin lesion dataset.
更多查看译文
关键词
unsupervised image synthesis,shape priors,tricyclegan,segmentation
AI 理解论文
溯源树
样例
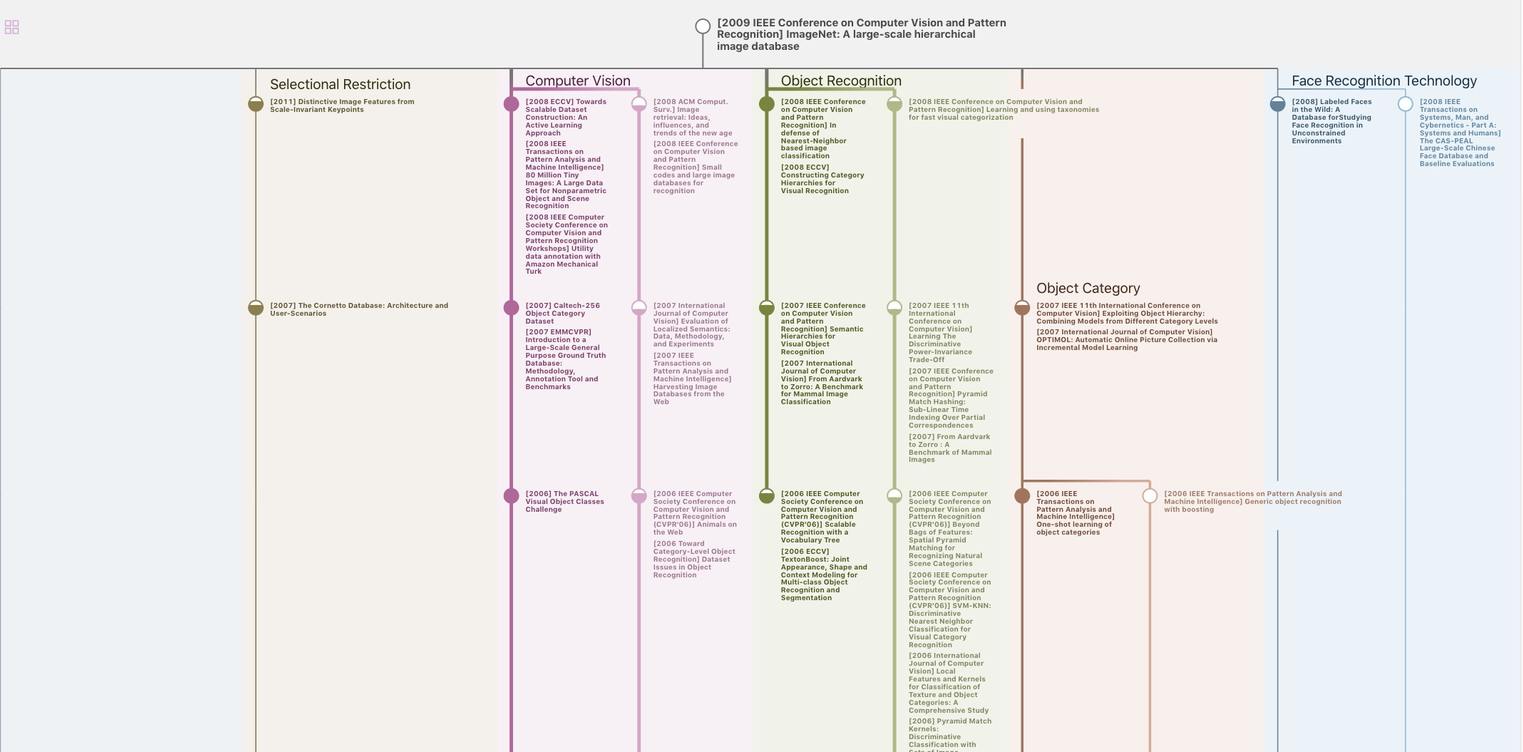
生成溯源树,研究论文发展脉络
Chat Paper
正在生成论文摘要