MaxiMin Active Learning in Overparameterized Model Classes
IEEE Journal on Selected Areas in Information Theory(2020)
摘要
Generating labeled training datasets has become a major bottleneck in Machine Learning (ML) pipelines. Active ML aims to address this issue by designing learning algorithms that automatically and adaptively select the most informative examples for labeling so that human time is not wasted labeling irrelevant, redundant, or trivial examples. This paper proposes a new approach to active ML with nonparametric or overparameterized models such as kernel methods and neural networks. In the context of binary classification, the new approach is shown to possess a variety of desirable properties that allow active learning algorithms to automatically and efficiently identify decision boundaries and data clusters.
更多查看译文
关键词
Active learning,overparameterized learning,neural networks,reproducing Kernel Hilbert spaces,pool-based learning
AI 理解论文
溯源树
样例
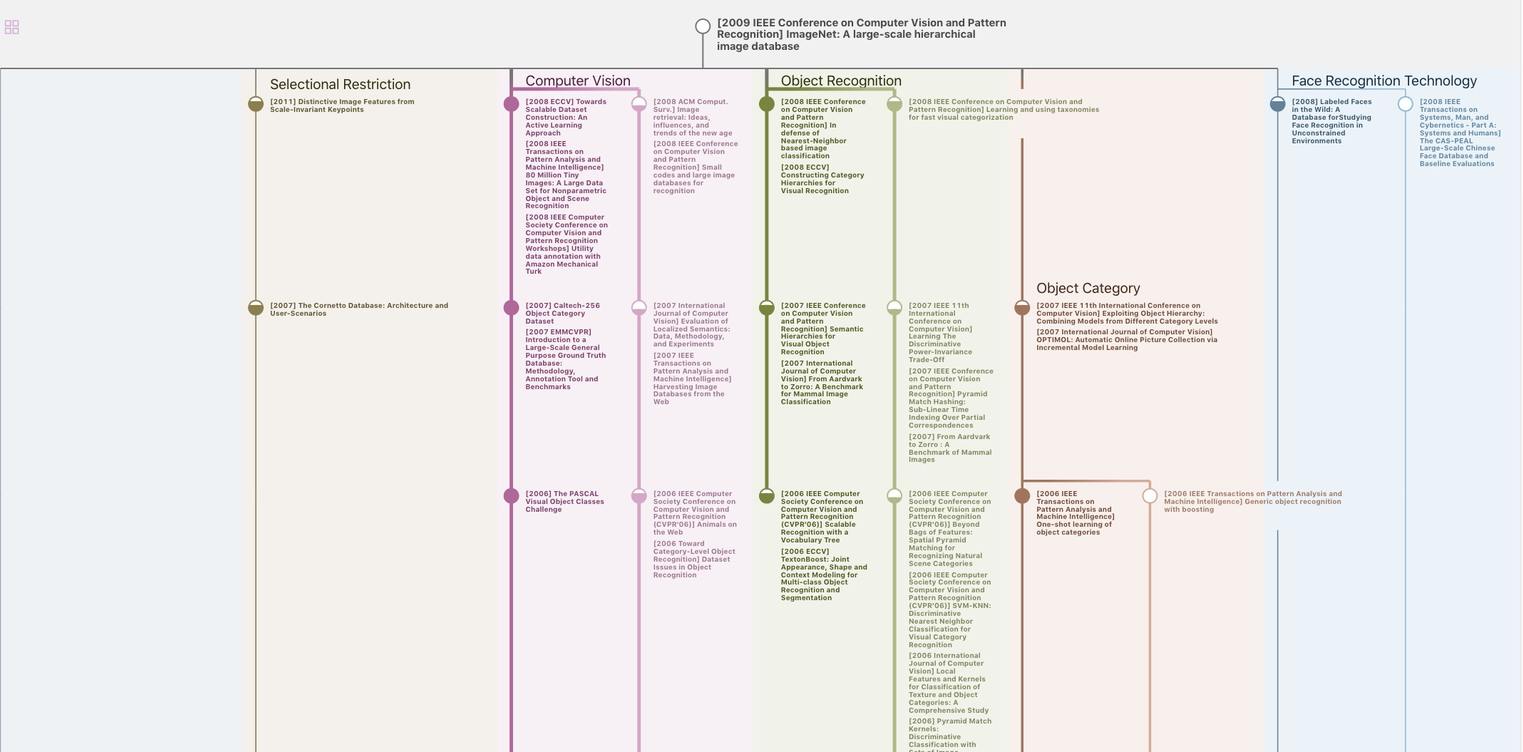
生成溯源树,研究论文发展脉络
Chat Paper
正在生成论文摘要