UPHDR-GAN: Generative Adversarial Network for High Dynamic Range Imaging With Unpaired Data
IEEE Transactions on Circuits and Systems for Video Technology(2022)
摘要
The paper proposes a method to effectively fuse multi-exposure inputs and generate high-quality high dynamic range (HDR) images with unpaired datasets. Deep learning-based HDR image generation methods rely heavily on paired datasets. The ground truth images play a leading role in generating reasonable HDR images. Datasets without ground truth are hard to be applied to train deep neural networks. Recently, Generative Adversarial Networks (GAN) have demonstrated their potentials of translating images from source domain
$X$
to target domain
$Y$
in the absence of paired examples. In this paper, we propose a GAN-based network for solving such problems while generating enjoyable HDR results, named UPHDR-GAN. The proposed method relaxes the constraint of the paired dataset and learns the mapping from the LDR domain to the HDR domain. Although the pair data are missing, UPHDR-GAN can properly handle the ghosting artifacts caused by moving objects or misalignments with the help of the modified GAN loss, the improved discriminator network and the useful initialization phase. The proposed method preserves the details of important regions and improves the total image perceptual quality. Qualitative and quantitative comparisons against the representative methods demonstrate the superiority of the proposed UPHDR-GAN.
更多查看译文
关键词
Multi-exposure HDR imaging,generative adversarial network,unpaired data
AI 理解论文
溯源树
样例
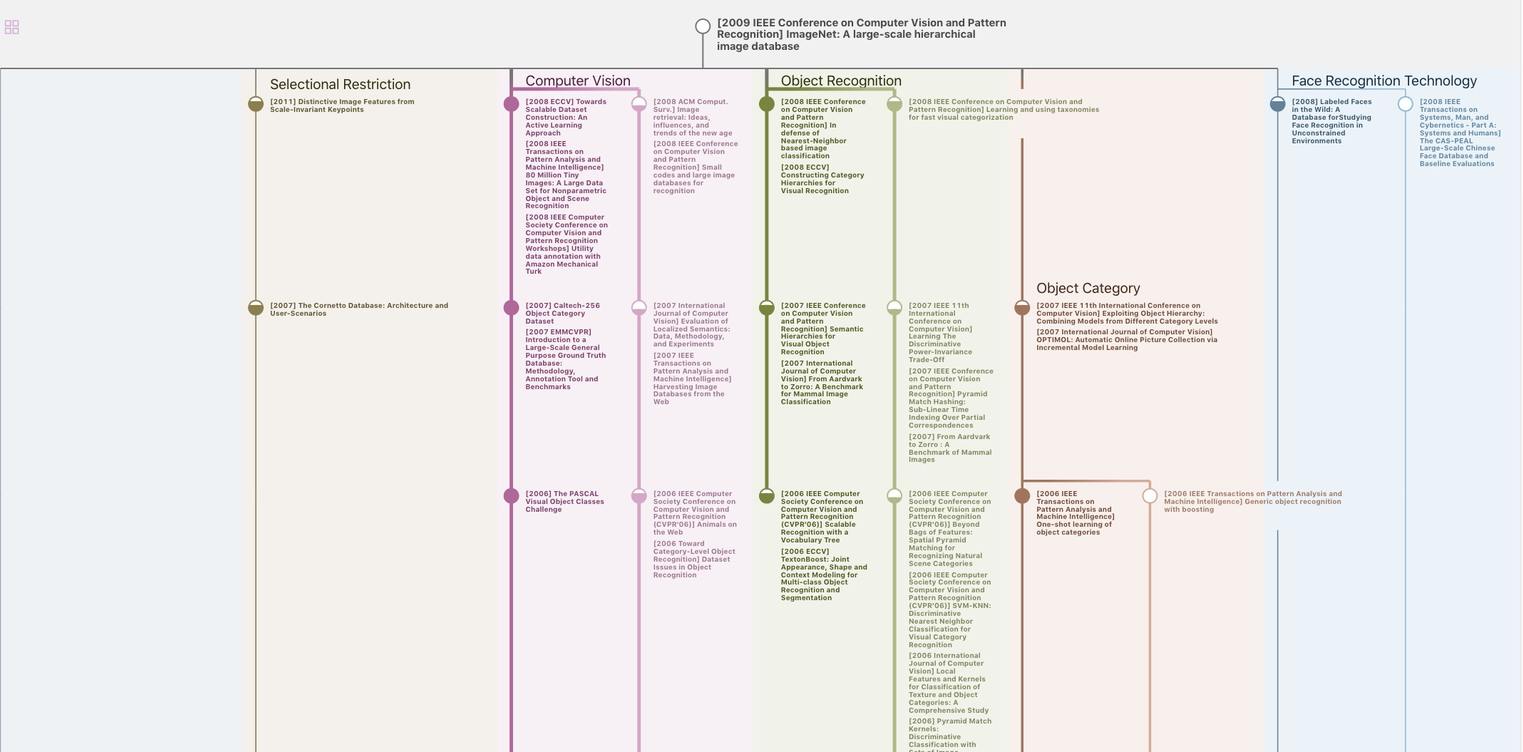
生成溯源树,研究论文发展脉络
Chat Paper
正在生成论文摘要