Advanced Learning Architectures and Spatial Statistics for Beam Selection with Multi-Path
GLOBECOM 2020 - 2020 IEEE Global Communications Conference(2020)
摘要
In this paper, we investigate the applicability of machine and deep learning (ML/DL) techniques to uplink beam selection problems with hybrid beamforming architectures. The goal is to select the element in the codebook of analog beamformers (ABF) yielding the highest sum-rate. The multi-antenna system operates in 5GNR's Frequency Range 2 (mmWave band). In this context, the presence of multi-path propagation along with the use of multi-carrier signals precludes the use of (single) angle-of-arrival information as an input to the learning system. Therefore, we investigate alternative statistics such as the singular vector/values of the (multi-carrier) channel matrix, the average covariance matrix, or the covariance matrix at a given subcarrier. Besides, we propose a novel ML/DL architecture enabling a continuous operation of the system which avoids the spectral efficiency losses associated to periodically switching to a dedicated ABF for the estimation of such statistics.
更多查看译文
关键词
angle-of-arrival information,learning system,average covariance matrix,dedicated ABF,advanced learning architectures,spatial statistics,deep learning techniques,beam selection problems,hybrid beamforming architectures,codebook,analog beamformers,multiantenna system,multipath propagation,multicarrier signals,5GNR frequency range,ML-DL techniques,machine learning,multicarrier channel matrix
AI 理解论文
溯源树
样例
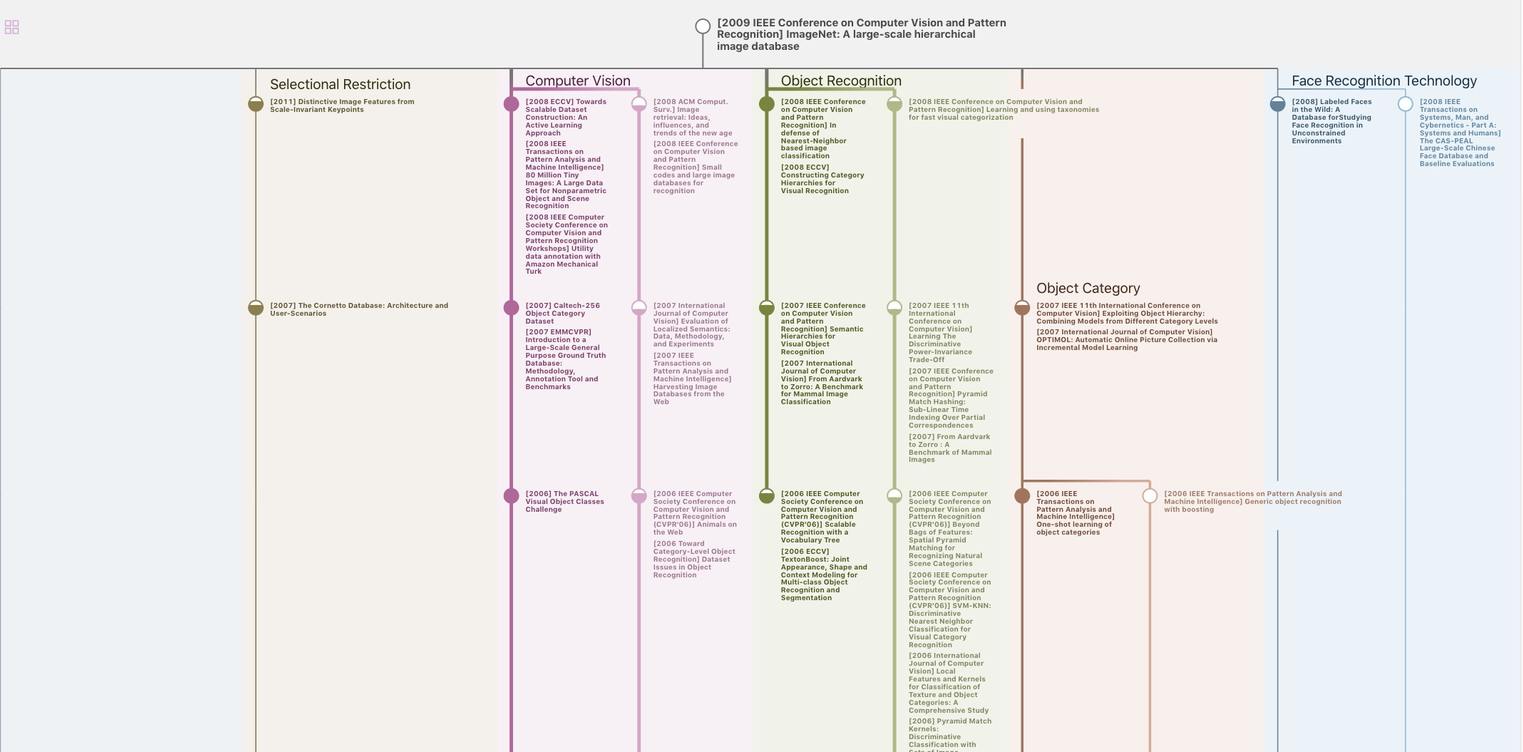
生成溯源树,研究论文发展脉络
Chat Paper
正在生成论文摘要