Explainable Early Stopping For Action Unit Recognition
2020 15TH IEEE INTERNATIONAL CONFERENCE ON AUTOMATIC FACE AND GESTURE RECOGNITION (FG 2020)(2020)
摘要
A common technique to avoid overfitting when training deep neural networks (DNN) is to monitor the performance in a dedicated validation data partition and to stop training as soon as it saturates. This only focuses on what the model does, while completely ignoring what happens inside it. In this work, we open the "black-box" of DNN in order to perform early stopping. We propose to use a novel theoretical framework that analyses meso-scale patterns in the topology of the functional graph of a network while it trains. Based on it, we decide when it transitions from learning towards overfitting in a more explainable way. We exemplify the benefits of this approach on a state-of-the art custom DNN that jointly learns local representations and label structure employing an ensemble of dedicated subnetworks. We show that it is practically equivalent in performance to early stopping with patience, the standard early stopping algorithm in the literature. This proves beneficial for AU recognition performance and provides new insights into how learning of AUs occurs in DNNs.
更多查看译文
关键词
deep learning,facial AU recognition,explainable deep learning
AI 理解论文
溯源树
样例
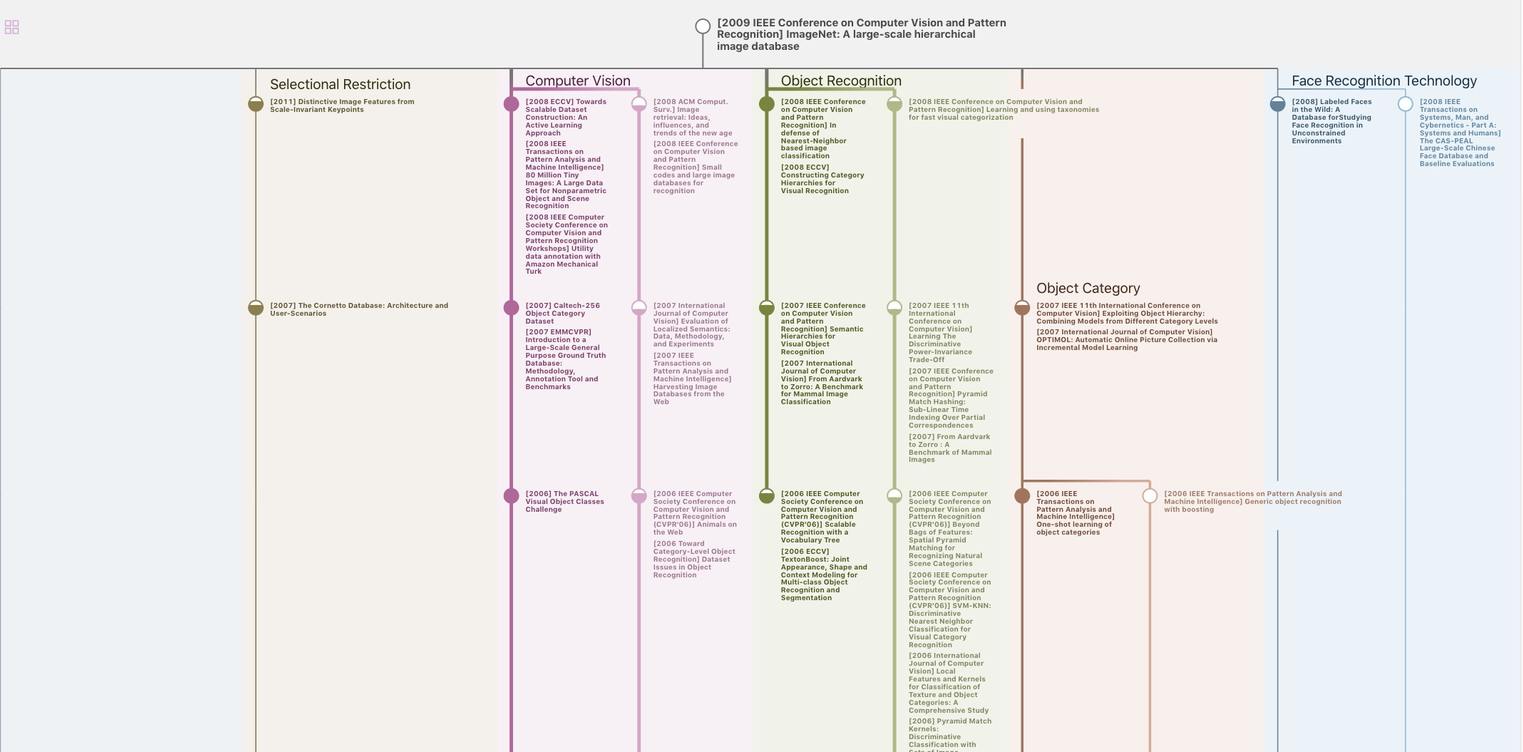
生成溯源树,研究论文发展脉络
Chat Paper
正在生成论文摘要