Closer than they appear: A Bayesian perspective on individual-level heterogeneity in risk assessment
JOURNAL OF THE ROYAL STATISTICAL SOCIETY SERIES A-STATISTICS IN SOCIETY(2022)
摘要
Risk assessment instruments are used across the criminal justice system to estimate the probability of some future event, such as failure to appear for a court appointment or re-arrest. The estimated probabilities are then used in making decisions at the individual level. In the past, there has been controversy about whether the probabilities derived from group-level calculations can meaningfully be applied to individuals. Using Bayesian hierarchical models applied to a large longitudinal dataset from the court system in the state of Kentucky, we analyse variation in individual-level probabilities of failing to appear for court and the extent to which it is captured by covariates. We find that individuals within the same risk group vary widely in their probability of the outcome. In practice, this means that allocating individuals to risk groups based on standard approaches to risk assessment, in large part, results in creating distinctions among individuals who are not meaningfully different in terms of their likelihood of the outcome. This is because uncertainty about the probability that any particular individual will fail to appear is large relative to the difference in average probabilities among any reasonable set of risk groups.
更多查看译文
关键词
algorithmic fairness, automated decision making, Bayesian, multilevel model, risk assessment
AI 理解论文
溯源树
样例
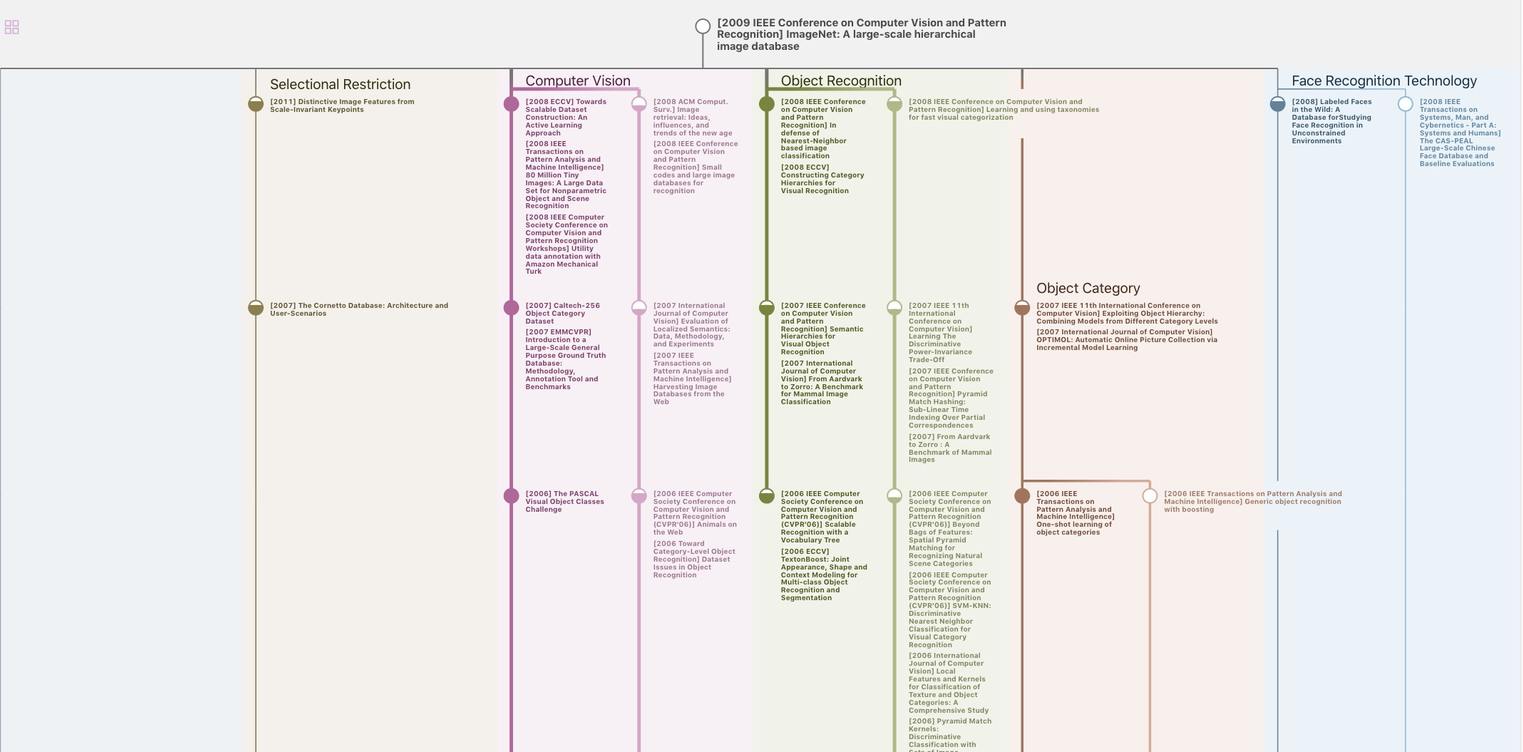
生成溯源树,研究论文发展脉络
Chat Paper
正在生成论文摘要