Information-Theoretic Generalization Bounds for Stochastic Gradient Descent.
COLT(2021)
摘要
We study the generalization properties of the popular stochastic gradient descent method for optimizing general non-convex loss functions. Our main contribution is providing upper bounds on the generalization error that depend on local statistics of the stochastic gradients evaluated along the path of iterates calculated by SGD. The key factors our bounds depend on are the variance of the gradients (with respect to the data distribution) and the local smoothness of the objective function along the SGD path, and the sensitivity of the loss function to perturbations to the final output. Our key technical tool is combining the information-theoretic generalization bounds previously used for analyzing randomized variants of SGD with a perturbation analysis of the iterates.
更多查看译文
关键词
stochastic gradient descent,generalization,information-theoretic
AI 理解论文
溯源树
样例
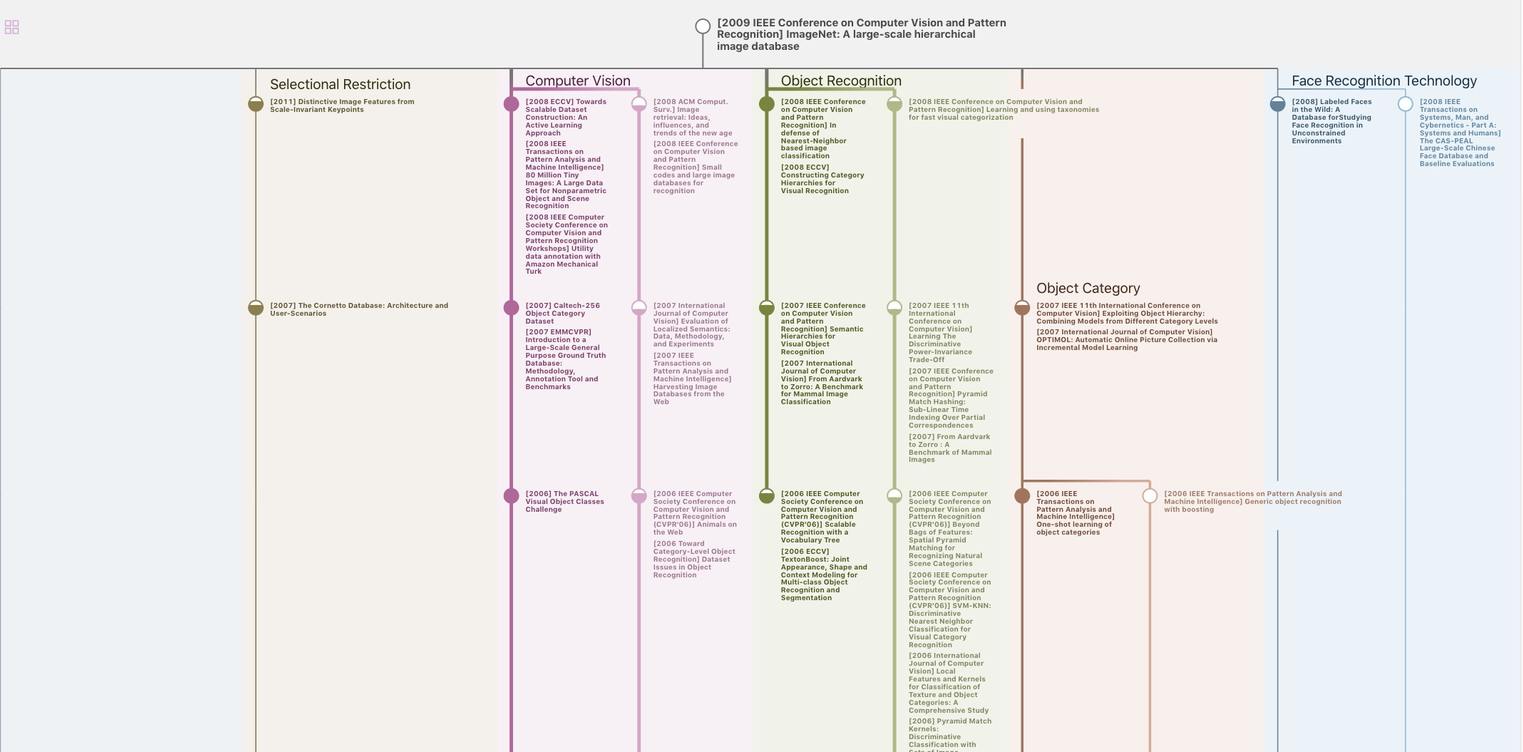
生成溯源树,研究论文发展脉络
Chat Paper
正在生成论文摘要