Fairness through Social Welfare Optimization
arxiv(2022)
摘要
We propose social welfare optimization as a general paradigm for formalizing fairness in AI systems. We argue that optimization models allow formulation of a wide range of fairness criteria as social welfare functions, while enabling AI to take advantage of highly advanced solution technology. Rather than attempting to reduce bias between selected groups, one can achieve equity across all groups by incorporating fairness into the social welfare function. This also allows a fuller accounting of the welfare of the individuals involved. We show how to integrate social welfare optimization with both rule-based AI and machine learning, using either an in-processing or a post-processing approach. We present empirical results from a case study as a preliminary examination of the validity and potential of these integration strategies.
更多查看译文
AI 理解论文
溯源树
样例
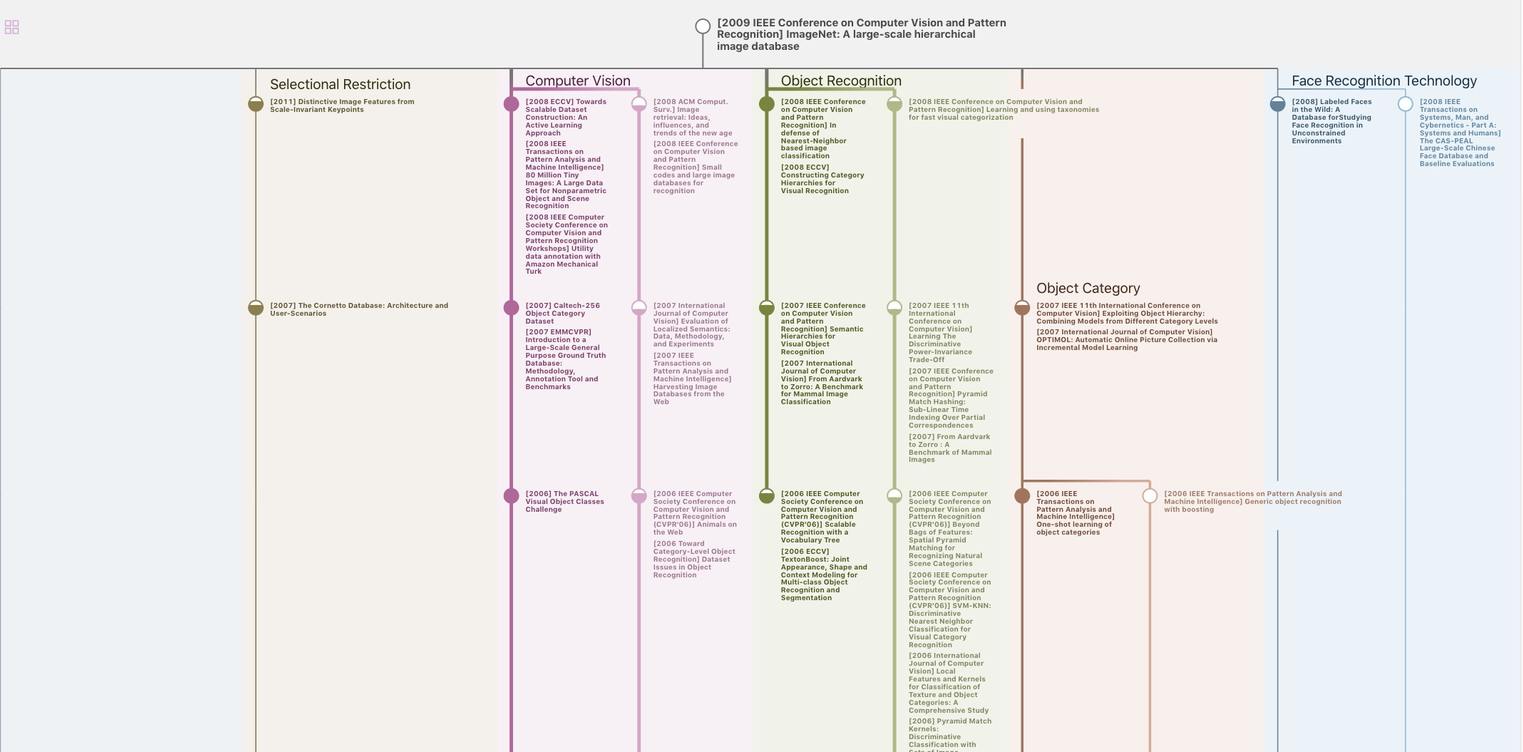
生成溯源树,研究论文发展脉络
Chat Paper
正在生成论文摘要