Gaussian process latent class choice models
TRANSPORTATION RESEARCH PART C-EMERGING TECHNOLOGIES(2022)
摘要
We present a Gaussian Process - Latent Class Choice Model (GP-LCCM) to integrate a non parametric class of probabilistic machine learning within discrete choice models (DCMs). Gaussian Processes (GPs) are kernel-based algorithms that incorporate expert knowledge by assuming priors over latent functions rather than priors over parameters, which makes them more flexible in addressing nonlinear problems. By integrating a Gaussian Process within a LCCM structure, we aim at improving discrete representations of unobserved heterogeneity. The proposed model would assign individuals probabilistically to behaviorally homogeneous clusters (latent classes) using GPs and simultaneously estimate class-specific choice models by relying on random utility models. Furthermore, we derive and implement an Expectation-Maximization (EM) algorithm to jointly estimate/infer the hyperparameters of the GP kernel function and the class-specific choice parameters by relying on a Laplace approximation and gradient-based numerical optimization methods, respectively. The model is tested on two different mode choice applications and compared against different LCCM benchmarks. Results show that GP-LCCM allows for a more complex and flexible representation of heterogeneity and improves both in sample fit and out-of-sample predictive power. Moreover, behavioral and economic interpretability is maintained at the class-specific choice model level while local interpretation of the latent classes can still be achieved, although the non-parametric characteristic of GPs lessens the transparency of the model.
更多查看译文
关键词
Discrete choice models, Latent class choice models, Machine learning, Gaussian process, EM algorithm
AI 理解论文
溯源树
样例
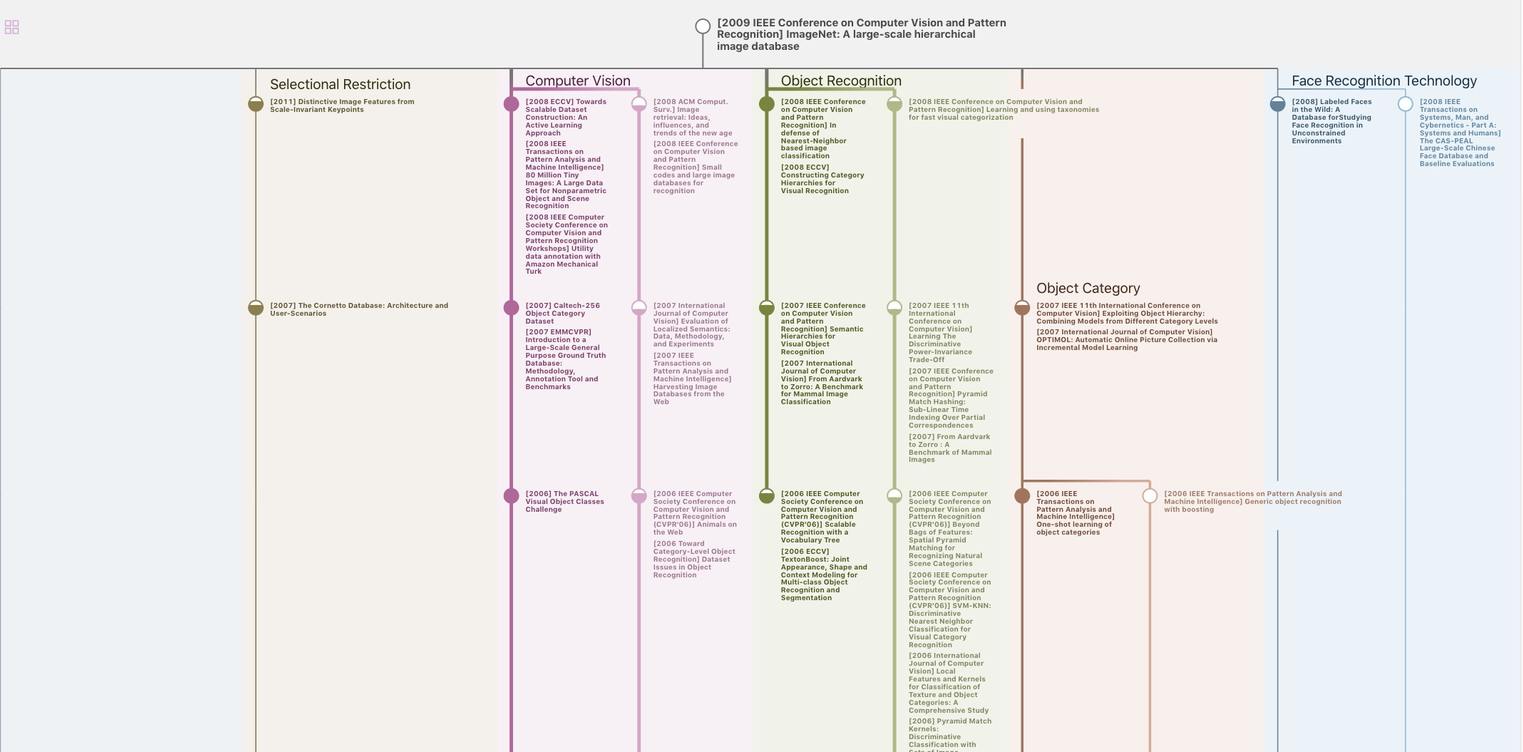
生成溯源树,研究论文发展脉络
Chat Paper
正在生成论文摘要