Pet/Ct For Brain Amyloid A Feasibility Study For Scan Time Reduction By Deep Learning
CLINICAL NUCLEAR MEDICINE(2021)
摘要
PurposeThis study was to develop a convolutional neural network (CNN) model with a residual learning framework to predict the full-time F-18-florbetaben (F-18-FBB) PET/CT images from corresponding short-time scans. MethodsIn this retrospective study, we enrolled 22 cognitively normal subjects, 20 patients with mild cognitive impairment, and 42 patients with Alzheimer disease. Twenty minutes of list-mode PET/CT data were acquired and reconstructed as the ground-truth images. The short-time scans were made in either 1, 2, 3, 4, or 5 minutes. The CNN with a residual learning framework was implemented to predict the ground-truth images of F-18-FBB PET/CT using short-time scans with either a single-slice or a 3-slice input layer. Model performance was evaluated by quantitative and qualitative analyses. Additionally, we quantified the amyloid load in the ground-truth and predicted images using the SUV ratio. ResultsOn quantitative analyses, with increasing scan time, the normalized root-mean-squared error and the SUV ratio differences between predicted and ground-truth images gradually decreased, and the peak signal-to-noise ratio increased. On qualitative analysis, the predicted images from the 3-slice CNN model showed better image quality than those from the single-slice model. The 3-slice CNN model with a short-time scan of at least 2 minutes achieved comparable, quantitative prediction of full-time F-18-FBB PET/CT images, with adequate to excellent image quality. ConclusionsThe 3-slice CNN model with a residual learning framework is promising for the prediction of full-time F-18-FBB PET/CT images from short-time scans.
更多查看译文
关键词
Alzheimer disease, amyloid imaging, deep learning, PET, CT
AI 理解论文
溯源树
样例
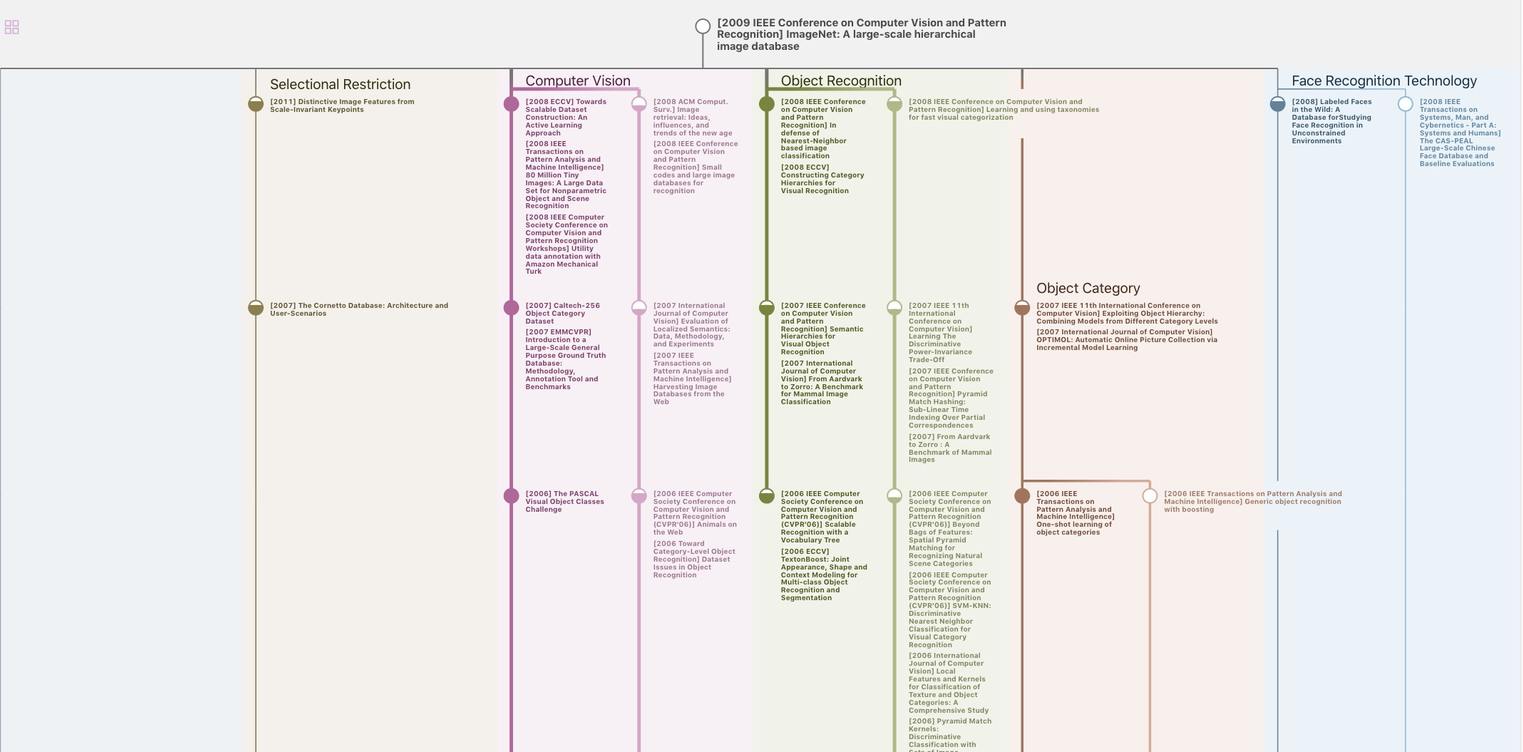
生成溯源树,研究论文发展脉络
Chat Paper
正在生成论文摘要