A Comparative Evaluation of Learned Feature Descriptors on Hybrid Monocular Visual SLAM Methods
2020 Latin American Robotics Symposium (LARS), 2020 Brazilian Symposium on Robotics (SBR) and 2020 Workshop on Robotics in Education (WRE)(2020)
摘要
Classical Visual Simultaneous Localization and Mapping (VSLAM) algorithms can be easily induced to fail when either the robot's motion or the environment is too challenging. The use of Deep Neural Networks to enhance VSLAM algorithms has recently achieved promising results, which we call hybrid methods. In this paper, we compare the performance of hybrid monocular VSLAM methods with different learned feature descriptors. To this end, we propose a set of experiments to evaluate the robustness of the algorithms under different environments, camera motion, and camera sensor noise. Experiments conducted on KITTI and Euroc MAV datasets confirm that learned feature descriptors can create more robust VSLAM systems.
更多查看译文
关键词
Classical Visual Simultaneous Localization,Mapping algorithms,robot,Deep Neural Networks,VSLAM algorithms,hybrid methods,hybrid monocular VSLAM methods,different learned feature descriptors,camera motion,robust VSLAM systems,comparative evaluation,hybrid monocular Visual SLAM methods
AI 理解论文
溯源树
样例
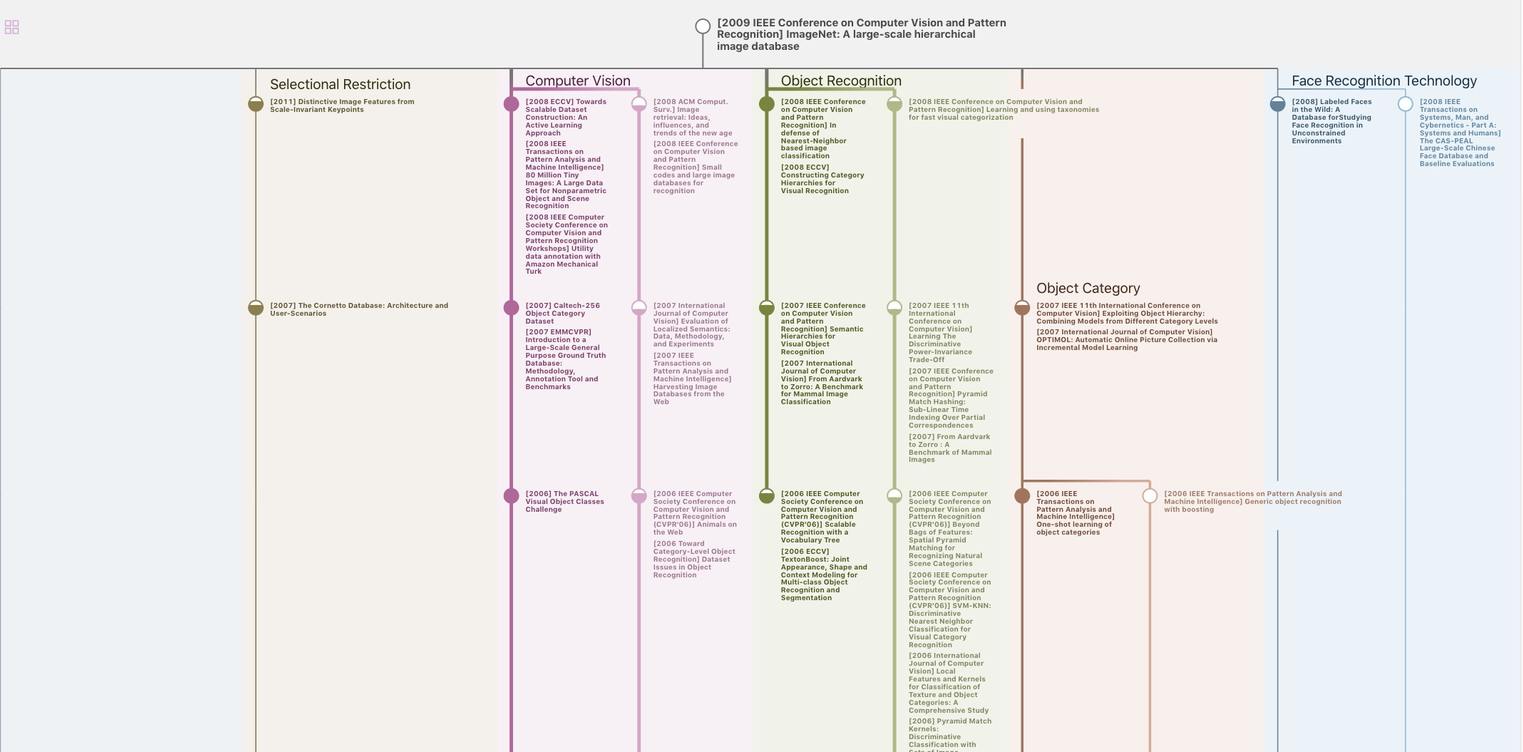
生成溯源树,研究论文发展脉络
Chat Paper
正在生成论文摘要