Poster Abstract: Examining Cross-Validation Strategies for Predictive Modeling of Anterior Cruciate Ligament Reinjury
2019 IEEE/ACM International Conference on Connected Health: Applications, Systems and Engineering Technologies (CHASE)(2019)
摘要
The ability to detect subtle changes in movement patterns is the most important step towards early and accurate detection of aberrant movement patterns that lead to Anterior Cruciate Ligament (ACL) reinjury which is a common and costly disease resulting in surgical reconstruction and rehabilitation costs well over $3 billion annually. Various types of commercially available wearable motion sensors have been used to assess gait and mobility characteristics in patients performing activities that resemble the demand for sports and in native sport environments. However, the question of to what extent the sensor data could be used to develop predictive models and aid in treatment decision making that may improve care in patients with an ACL injury. Therefore, this paper explores the influences of cross-validation strategies (e.g., record-wise, subject-wise) in developing machine learning models to predict the attributes of an ACL injury that play a significant role in clinical decision-making process including gender, left and right involved limbs, and comparison between patients and healthy controls. Six machine learning models were developed to examine the influences and experimental results demonstrated the performance of the models varied depending on the cross-validation methods and revealed practical implications for clinical decision-making process regarding ACL injury.
更多查看译文
关键词
ACL-Injury,Gait-Analysis,Predictive-Modeling,Leave-one-subject-out-cross-validation
AI 理解论文
溯源树
样例
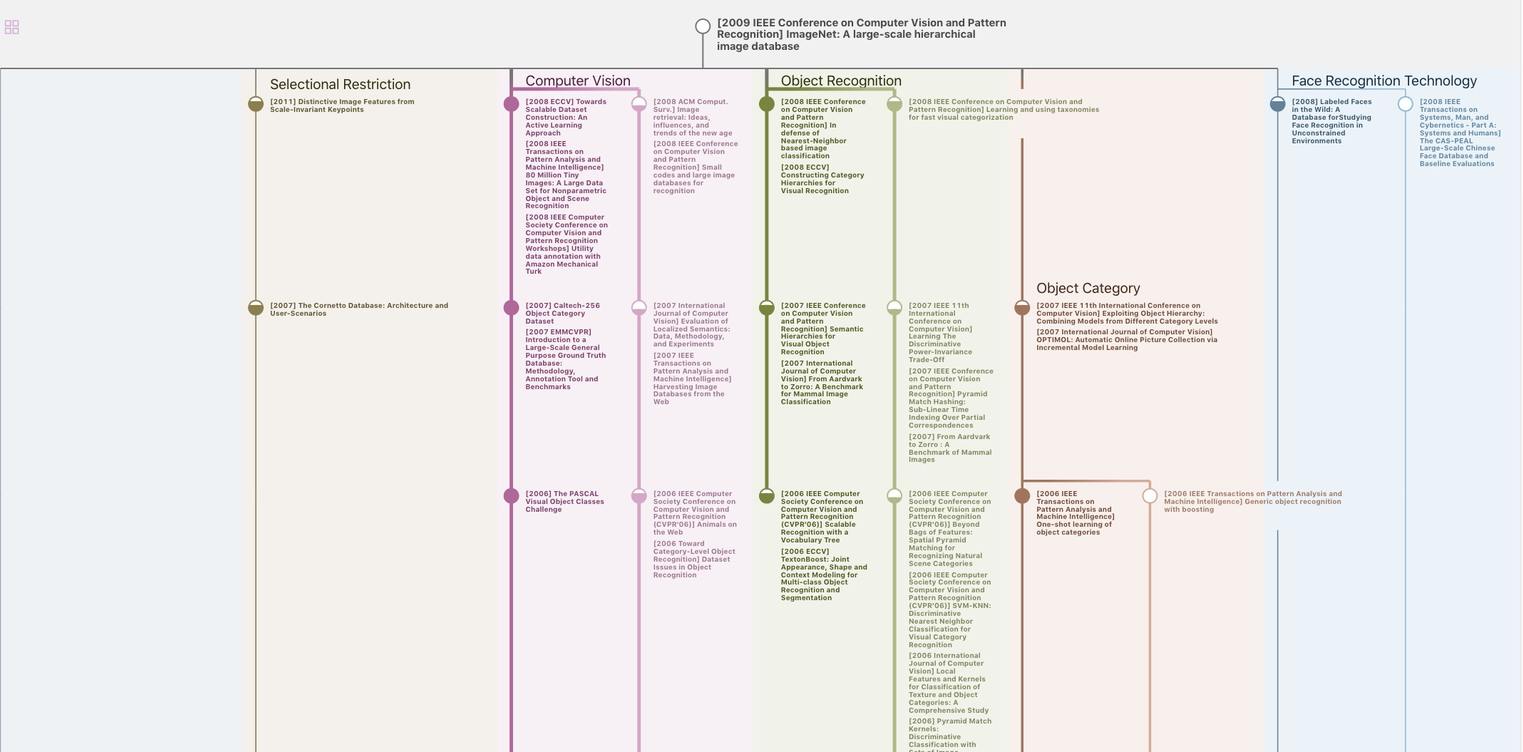
生成溯源树,研究论文发展脉络
Chat Paper
正在生成论文摘要