Levy adaptive b-spline regression via overcomplete systems
arxiv(2023)
摘要
Estimating functions with varying degrees of smoothness is a challenging problem in nonparametric function estimation. In this paper, we propose the Le ' vy adaptive B-Spline regression (LABS) model, an extension of the Le ' vy adaptive regression kernels (LARK) models, for estimating functions with varying degrees of smoothness. The LABS model is a LARK model with B-spline basis functions as generating kernels. The B-spline basis functions consist of piecewise k-degree polynomials with k - 1 continuous derivatives, and can systematically express functions with varying degrees of smoothness. By changing the order of the B-spline basis, the LABS model systematically adapts to the smoothness of the functions, for example, jump discontinuities, sharp peaks, and so on. The results of simulation studies and real-data examples show that the proposed model captures smooth areas, jumps, and sharp peaks of functions. The proposed model also perform best in almost all examples. Finally, we provide theoretical results that the mean function for the LABS model belongs to certain Besov spaces, based on the order of the B-spline basis, and that the prior of the model has full support on the Besov spaces.
更多查看译文
关键词
Key words and phrases: Besov space,Le ' vy random measure,Nonparametric func-,tion estimation,reversible jump Markov chain Monte Carlo.
AI 理解论文
溯源树
样例
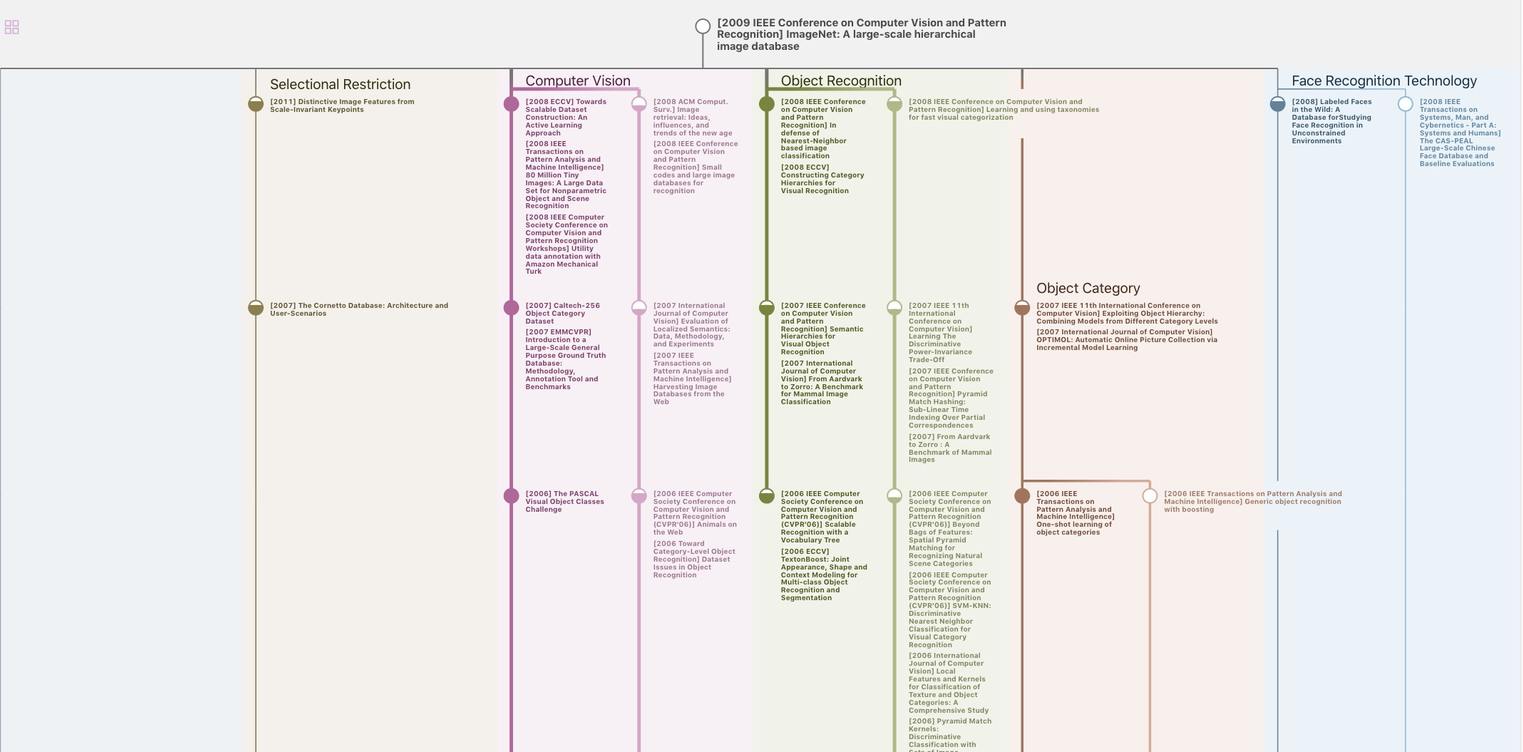
生成溯源树,研究论文发展脉络
Chat Paper
正在生成论文摘要