CORL: Compositional Representation Learning for Few-Shot Classification
arxiv(2023)
摘要
Few-shot image classification consists of two consecutive learning processes: 1) In the meta-learning stage, the model acquires a knowledge base from a set of training classes. 2) During meta-testing, the acquired knowledge is used to recognize unseen classes from very few examples. Inspired by the compositional representation of objects in humans, we train a neural network architecture that explicitly represents objects as a dictionary of shared components and their spatial composition. In particular, during meta-learning, we train a knowledge base that consists of a dictionary of component representations and a dictionary of component activation maps that encode common spatial activation patterns of components. The elements of both dictionaries are shared among the training classes. During meta-testing, the representation of unseen classes is learned using the component representations and the component activation maps from the knowledge base. Finally, an attention mechanism is used to strengthen those components that are most important for each category. We demonstrate the value of our interpretable compositional learning framework for a few-shot classification using miniImageNet, tieredImageNet, CIFAR-FS, and FC100, where we achieve comparable performance.
更多查看译文
关键词
compositional representation learning,classification,few-shot
AI 理解论文
溯源树
样例
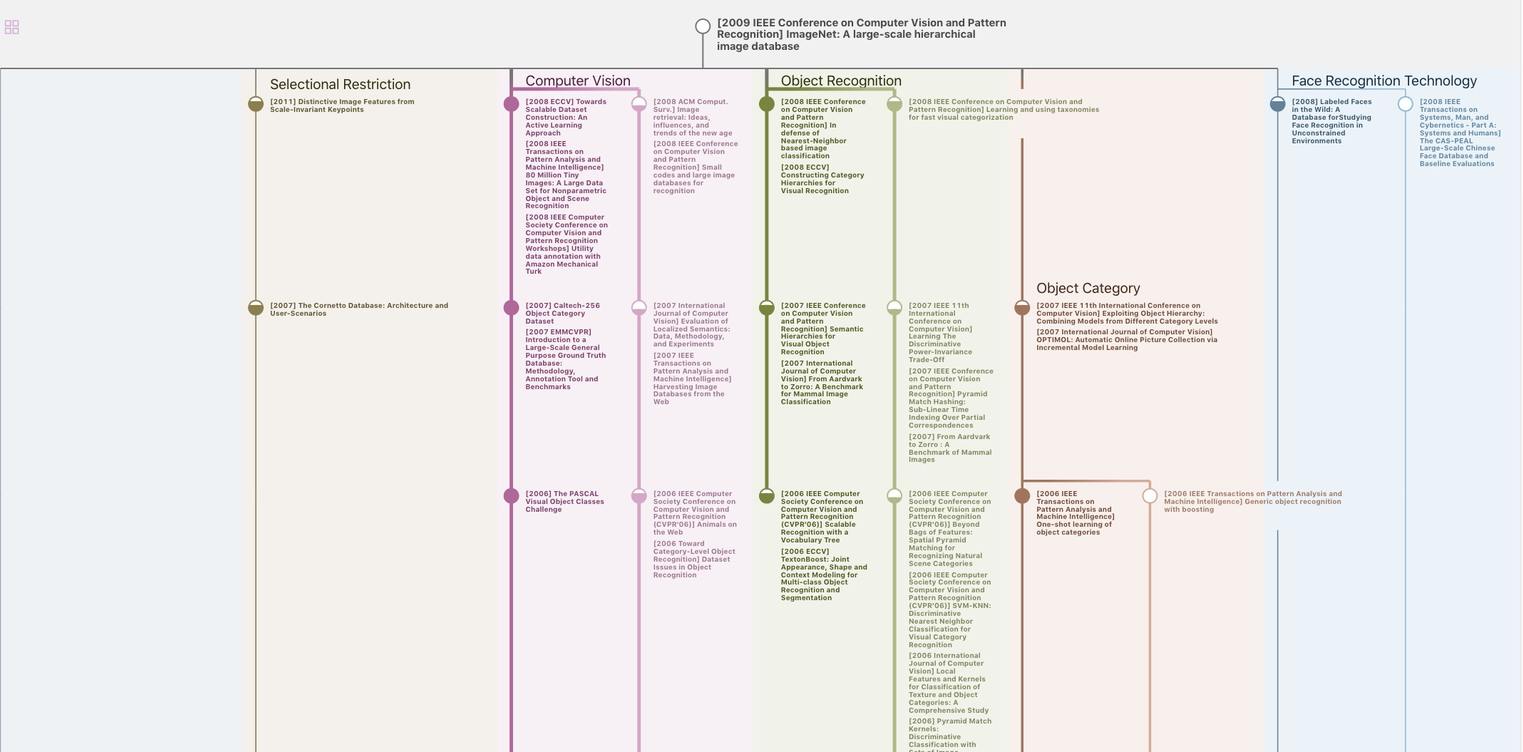
生成溯源树,研究论文发展脉络
Chat Paper
正在生成论文摘要