Structured Pruning of LSTMs via Eigenanalysis and Geometric Median for Mobile Multimedia and Deep Learning Applications
2020 IEEE International Symposium on Multimedia (ISM)(2020)
摘要
In this paper, a novel structured pruning approach for learning efficient long short-term memory (LSTM) network architectures is proposed. More specifically, the eigenvalues of the covariance matrix associated with the responses of each LSTM layer are computed and utilized to quantify the layers' redundancy and automatically obtain an individual pruning rate for each layer. Subsequently, a Geometric Median based (GM-based) criterion is used to identify and prune in a structured way the most redundant LSTM units, realizing the pruning rates derived in the previous step. The experimental evaluation on the Penn Treebank text corpus and the large-scale YouTube-8M audio-video dataset for the tasks of word-level prediction and visual concept detection, respectively, shows the efficacy of the proposed approach
1
.
更多查看译文
关键词
deep learning,mobile multimedia,LSTM,automatic structured pruning,eigenanalysis,geometric median
AI 理解论文
溯源树
样例
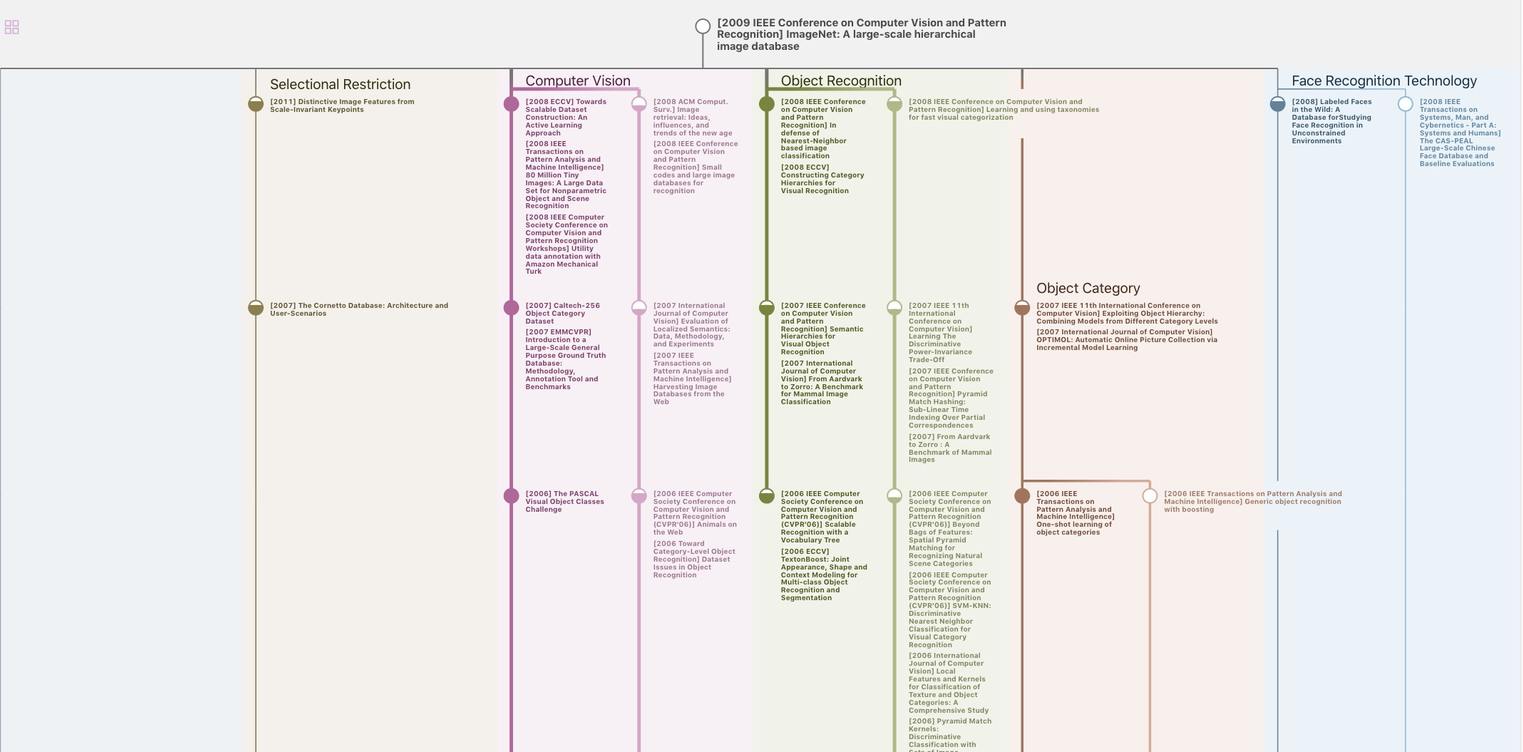
生成溯源树,研究论文发展脉络
Chat Paper
正在生成论文摘要