Recommending Geo-semantically Related Classes for Link Discovery
Journal on Data Semantics(2021)
摘要
The growth of Web of Data led to the development of dataset recommendation methodologies, which automate the discovery of datasets that may contain same or related instances (i.e., objects), in order to be used as input for several tasks including Link Discovery. The recommendation process takes as input one dataset (or any tripleset) and proposes other datasets which are the most likely to contain related instances. Existing recommenders determine the relevance between datasets by comparing their textual and structural similarity or by examining existing links among them. In this paper, we determine relevancy by comparing the geospatial relatedness of triplesets containing instances belonging to spatial classes (that is, classes containing instances whose locations are georeferenced by point geometries) based on the hypothesis that pairs of classes whose instances present similar spatial distribution are likely to contain semantically related instances. The proposed methodology builds summaries that capture the spatial distribution of classes. It utilizes the summaries, first, to rule out irrelevant (to an input class) classes by applying spatial filters and, then, to rank the remaining classes by applying a geospatial relatedness measure, so as the top ranked classes are more probable to contain related instances. The methodology’s evaluation contains an exploration of Web of Data spatial classes characteristics and a discussion of the experiment results that validate our hypothesis. We show that the spatial filtering reduces effectively and efficiently up to 99% the search space for relevant classes in Web of Data and that the proposed geospatial relatedness measures generate ranked lists of recommended classes with 62% mean average precision, approximately 35% higher than simple baselines.
更多查看译文
关键词
Web of Data, Spatial information, Dataset recommendation, Geo-semantic relevance
AI 理解论文
溯源树
样例
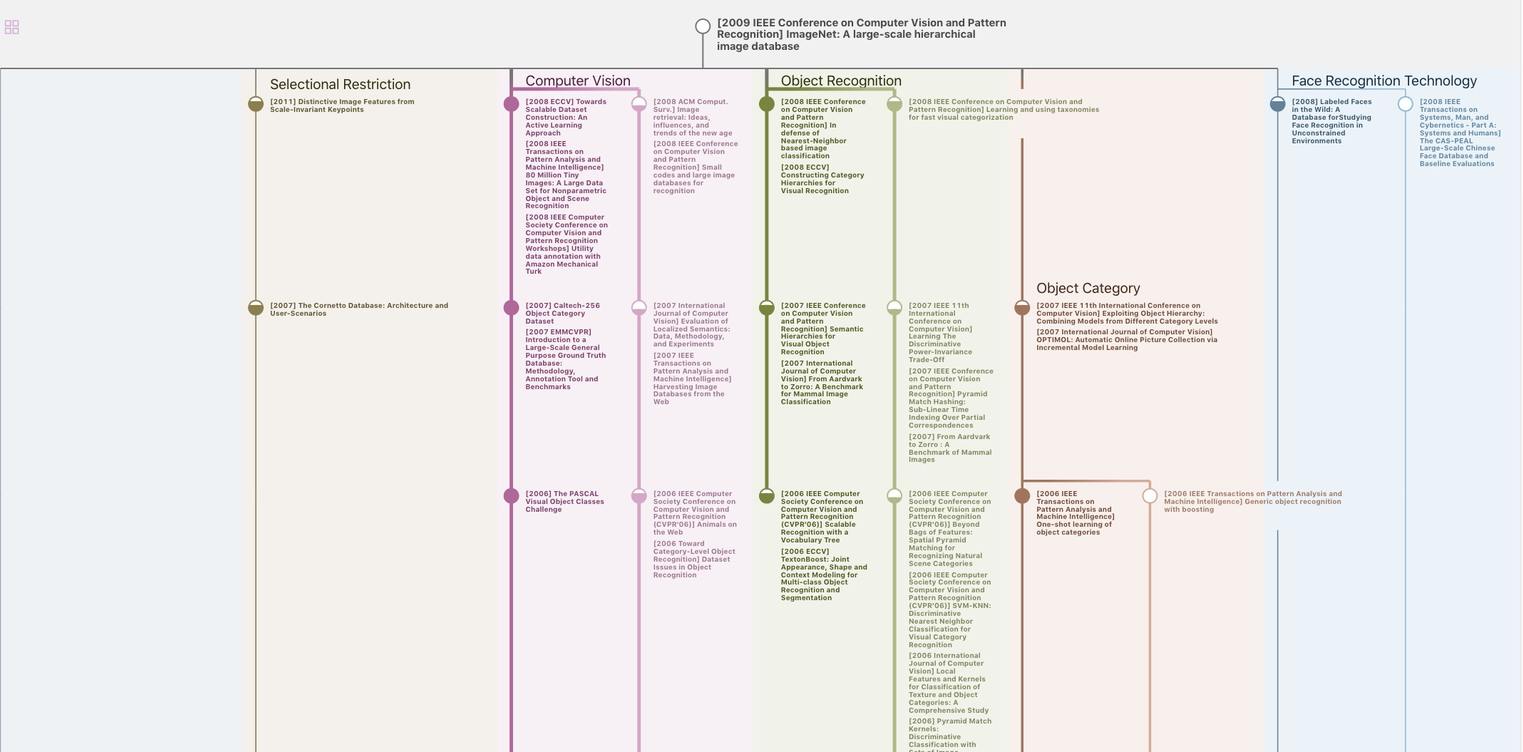
生成溯源树,研究论文发展脉络
Chat Paper
正在生成论文摘要