Deep Learning Based Segmentation Pipeline For Label-Free Phase-Contrast Microscopy Images
2020 28TH SIGNAL PROCESSING AND COMMUNICATIONS APPLICATIONS CONFERENCE (SIU)(2020)
摘要
The segmentation of cells is necessary for biologists in the morphological statistics for quantitative and qualitative analysis in Phase-contrast Microscopy (PCM) images. In this paper, we address the cell segmentation problem in PCM images. Deep Neural Networks (DNNs) commonly is initialized with weights from a network pre-trained on a large annotated data set like ImageNet have superior performance than those trained from scratch on a small dataset. Here, we demonstrate how encoder-decoder type architectures such as U-Net and Feature Pyramid Network (FPN) can be improved by an alternative encoder which pre-trained on the ImageNet dataset. In particular, our experimental results confirm that the image descriptors from ResNet-18 are highly effective in accurate prediction of the cell boundary and have higher Intersection over Union (IoU) in comparison to the classical U-Net and require fewer training epochs.
更多查看译文
关键词
Cell Segmentation, Phase-Contrast microscopy, Deep learning
AI 理解论文
溯源树
样例
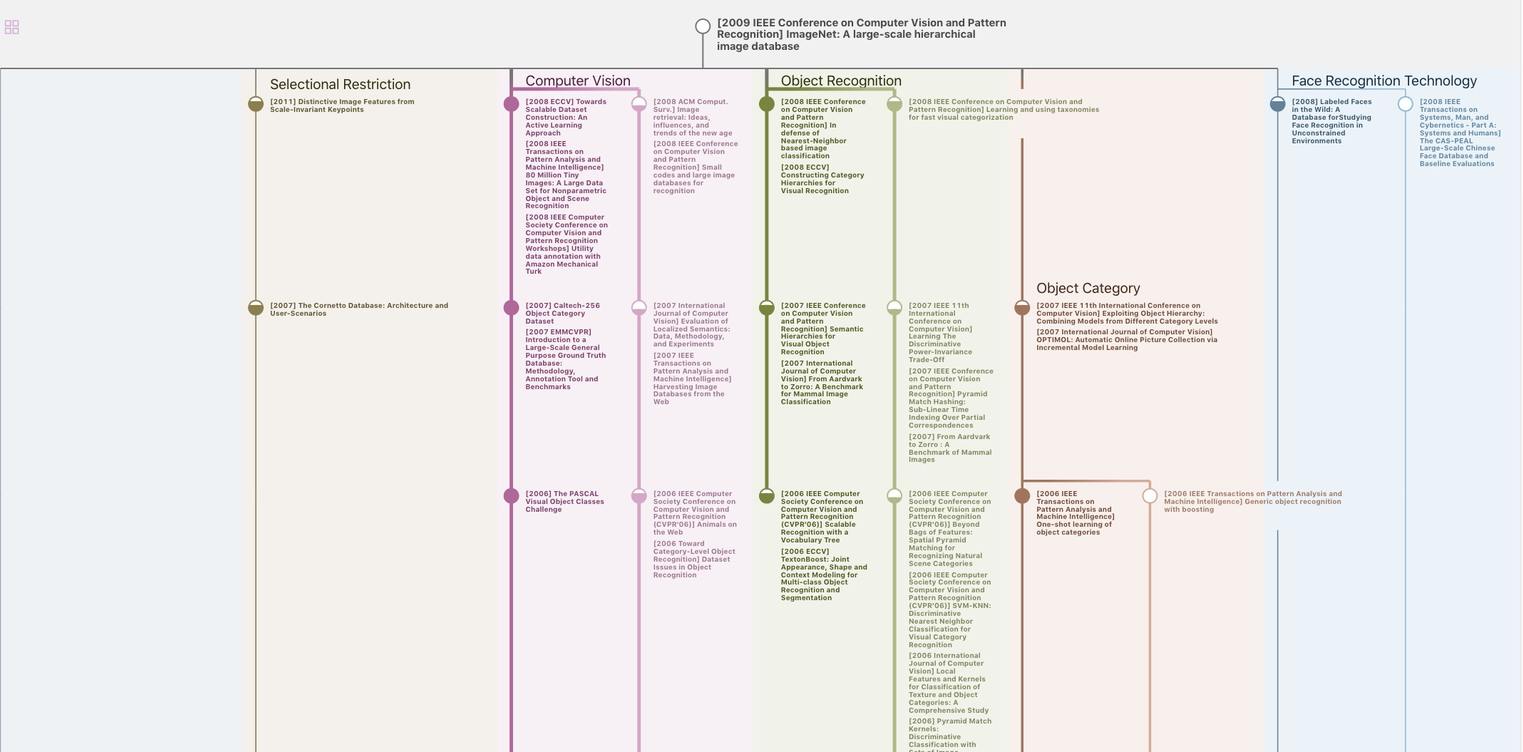
生成溯源树,研究论文发展脉络
Chat Paper
正在生成论文摘要