Denoising Single Voxel Magnetic Resonance Spectroscopy with Deep Learning on Repeatedly Sampled In Vivo Data
arxiv(2021)
摘要
Objective: Magnetic Resonance Spectroscopy (MRS) is a noninvasive tool to reveal metabolic information. One challenge of MRS is the relatively low Signal-Noise Ratio (SNR) due to low concentrations of metabolites. To improve the SNR, the most common approach is to average signals that are acquired in multiple times. The data acquisition time, however, is increased by multiple times accordingly, resulting in the scanned objects uncomfortable or even unbearable. Methods: By exploring the multiple sampled data, a deep learning denoising approach is proposed to learn a mapping from the low SNR signal to the high SNR one. Results: Results on simulated and in vivo data show that the proposed method significantly reduces the data acquisition time with slightly compromised metabolic accuracy. Conclusion: A deep learning denoising method was proposed to significantly shorten the time of data acquisition, while maintaining signal accuracy and reliability. Significance: Provide a solution of the fundamental low SNR problem in MRS with artificial intelligence.
更多查看译文
关键词
Magnetic resonance spectroscopy,deep learning,denoising,in vivo data,metabolites
AI 理解论文
溯源树
样例
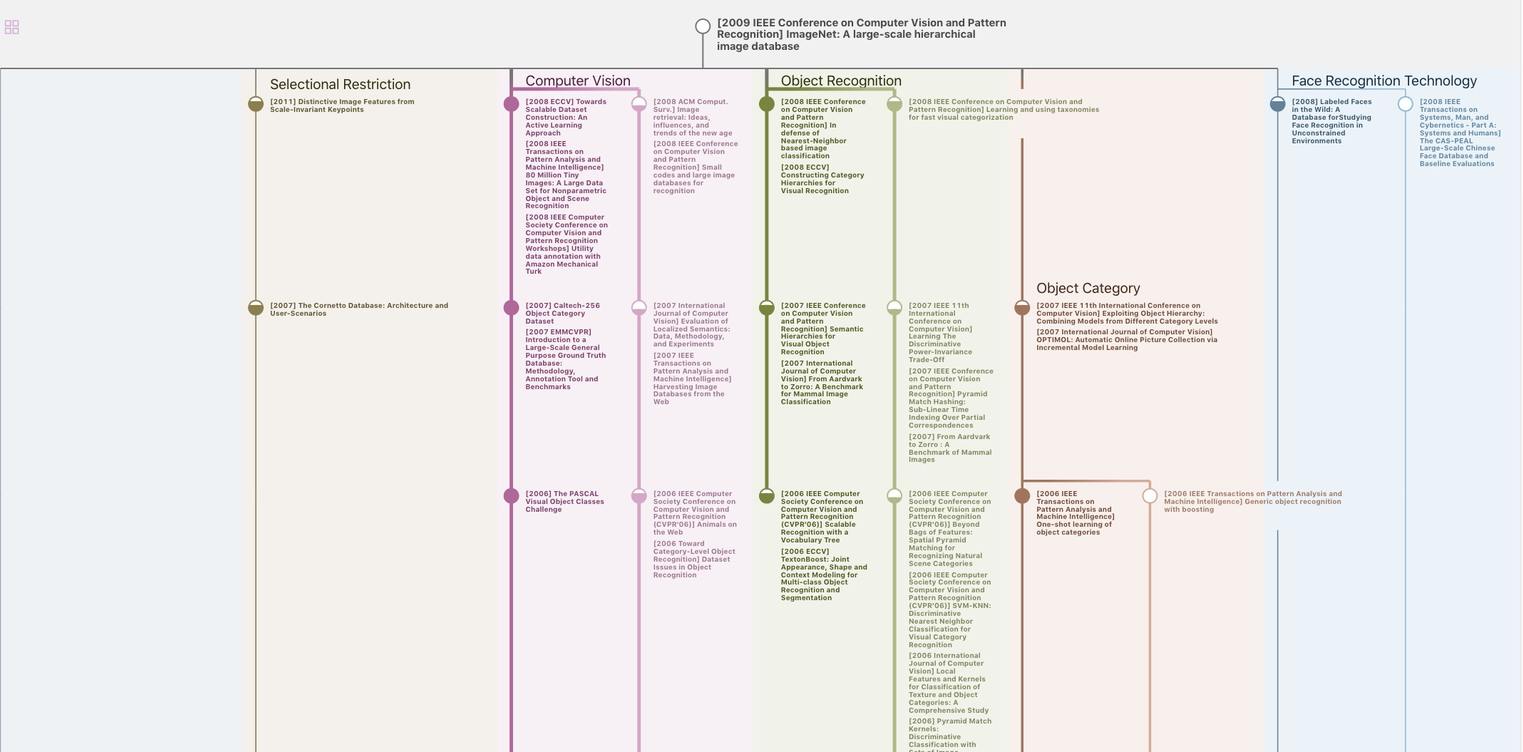
生成溯源树,研究论文发展脉络
Chat Paper
正在生成论文摘要