Towards Robustness to Label Noise in Text Classification via Noise Modeling
Conference on Information and Knowledge Management(2021)
摘要
BSTRACTLarge datasets in NLP tend to suffer from noisy labels due to erroneous automatic and human annotation procedures. We study the problem of text classification with label noise, and aim to capture this noise through an auxiliary noise model over the classifier. We first assign a probability score to each training sample of having a clean or noisy label, using a two-component beta mixture model fitted on the training losses at an early epoch. Using this, we jointly train the classifier and the noise model through a novel de-noising loss having two components: (i) cross-entropy of the noise model prediction with the input label, and (ii) cross-entropy of the classifier prediction with the input label, weighted by the probability of the sample having a clean label. Our empirical evaluation on two text classification tasks and two types of label noise: random and input-conditional, shows that our approach can improve classification accuracy, and prevent over-fitting to the noise.
更多查看译文
关键词
label noise,text classification,robustness
AI 理解论文
溯源树
样例
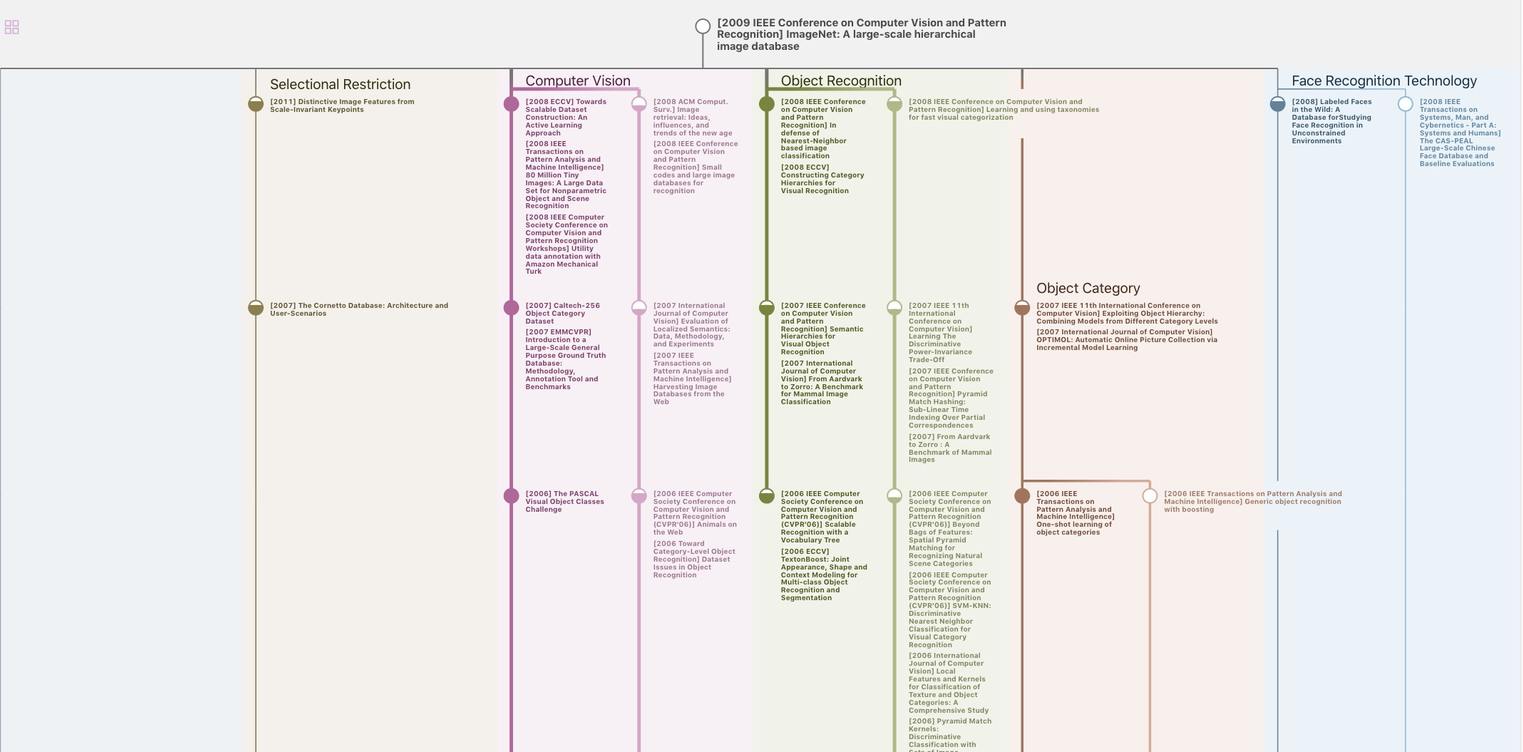
生成溯源树,研究论文发展脉络
Chat Paper
正在生成论文摘要