A new model of decision processing in instrumental learning tasks.
eLife(2021)
摘要
Learning and decision-making are interactive processes, yet cognitive modeling of error-driven learning and decision-making have largely evolved separately. Recently, evidence accumulation models (EAMs) of decision-making and reinforcement learning (RL) models of error-driven learning have been combined into joint RL-EAMs that can in principle address these interactions. However, we show that the most commonly used combination, based on the diffusion decision model (DDM) for binary choice, consistently fails to capture crucial aspects of response times observed during reinforcement learning. We propose a new RL-EAM based on an advantage racing diffusion (ARD) framework for choices among two or more options that not only addresses this problem but captures stimulus difficulty, speed-accuracy trade-off, and stimulus-response-mapping reversal effects. The RL-ARD avoids fundamental limitations imposed by the DDM on addressing effects of absolute values of choices, as well as extensions beyond binary choice, and provides a computationally tractable basis for wider applications.
更多查看译文
关键词
value-based decision making,reinforcement learning,computational modelling,evidence accumulation
AI 理解论文
溯源树
样例
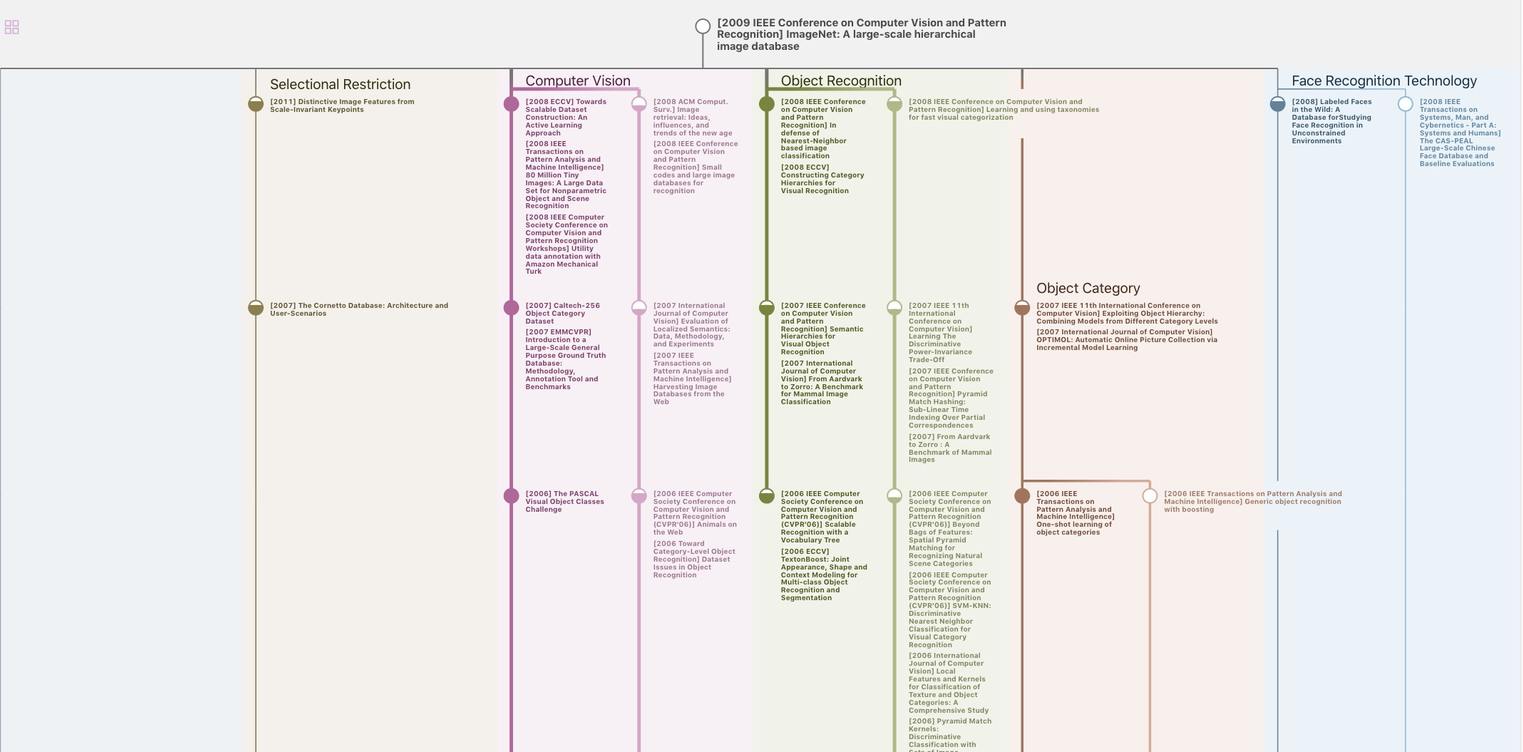
生成溯源树,研究论文发展脉络
Chat Paper
正在生成论文摘要