Risk-Sensitive Model Predictive Control
arxiv(2021)
摘要
We present heuristic policies and performance bounds for risk-sensitive convex stochastic control that generalize linear-exponential-quadratic regulator (LEQR) theory. Our heuristic policy extends standard, risk-neutral model predictive control (MPC): instead of ignoring uncertain noise terms, as in MPC, our heuristic policy assumes these noise terms turns out either favorably or unfavorably, depending on a risk aversion parameter. In the risk-seeking case, this modified planning problem is convex. In the risk-averse case, it requires minimizing a difference of convex functions, which is done (approximately) using the convex--concave procedure. In both cases, we obtain a lower bound on the optimal cost as a by-product of solving the planning problem. We give a numerical example of controlling a battery to power an uncertain load, and show that risk-averse MPC reduces the risk of a very bad outcome (as compared with standard, risk-neutral MPC) with negligible impact on the the average performance.
更多查看译文
关键词
predictive control,model,risk-sensitive
AI 理解论文
溯源树
样例
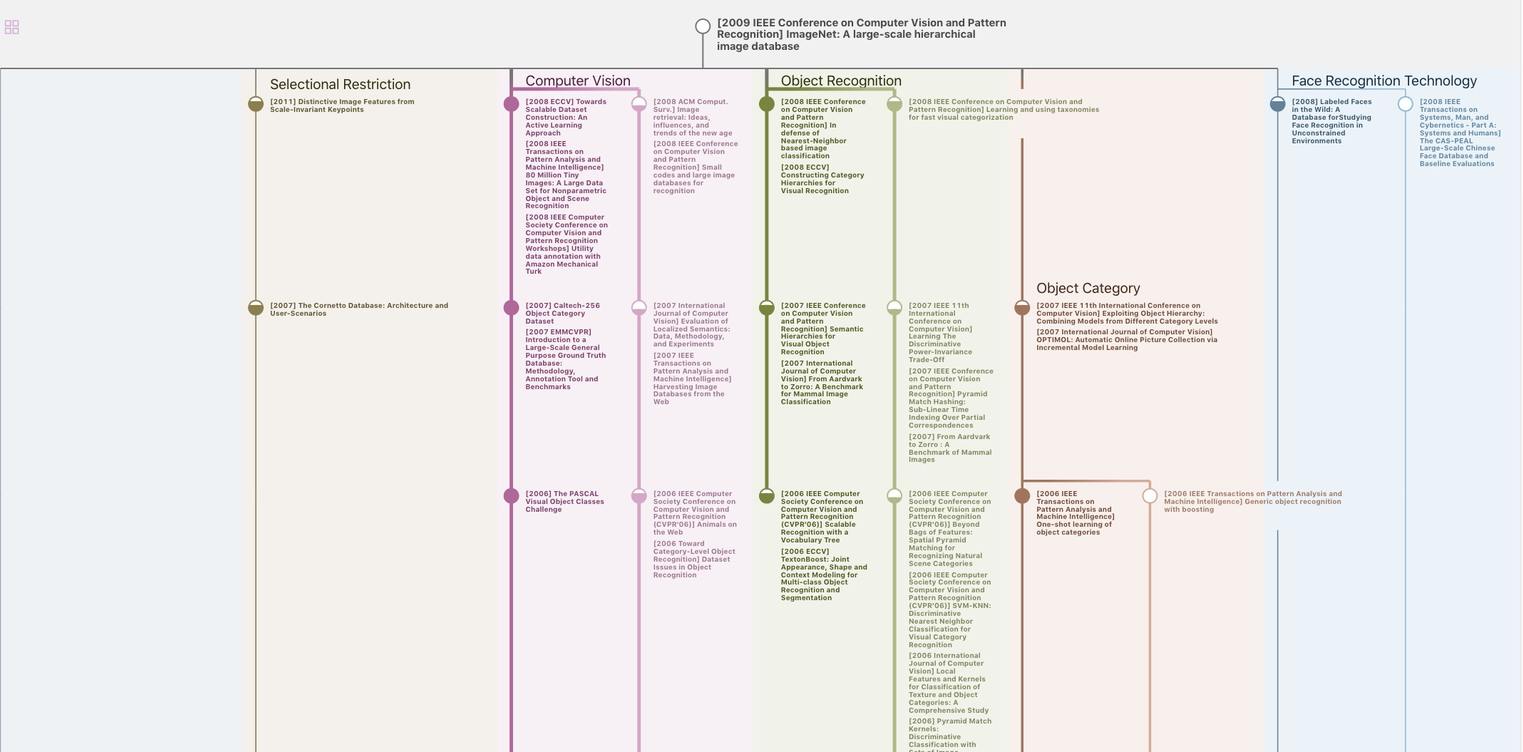
生成溯源树,研究论文发展脉络
Chat Paper
正在生成论文摘要