BSR: A Balanced Framework For Single Image Super Resolution
2020 ITU Kaleidoscope: Industry-Driven Digital Transformation (ITU K)(2020)
摘要
The reconstruction effect of single image super resolution (SISR) has been greatly improved over the traditional statistic and feature-based methods since the wide application of deep convolutional neural networks (DCNNs). Most recent studies mainly focused on the complexity of the neural network models and the stability of the training process without paying much attention to imbalance problems in the fields of super resolution. In this paper, we study three imbalance effects: sample imbalance, feature imbalance, and object function imbalance. A novel framework, which is called Balanced Super Resolution (BSR), is thus proposed to tackle these issues. Specifically, we propose a random filter sampling algorithm to form balanced training sets during batch training. Meanwhile, a feature mapping group, which is a kind of residual structure, is introduced to forward various groups of low-level information to high-level. A light spatial attention mechanism is also proposed to improve the effectiveness of residual features. Furthermore, we study the object functions in traditional SISR networks and deploy a hybrid L1/L2/Lp structure that favors visually-stable SR output. The proposed design achieves persistently better image quality than state-of-the-art DCNN methods in both subjective and objective measurements.
更多查看译文
关键词
Balance,deep convolutional neural network,framework,super-resolution
AI 理解论文
溯源树
样例
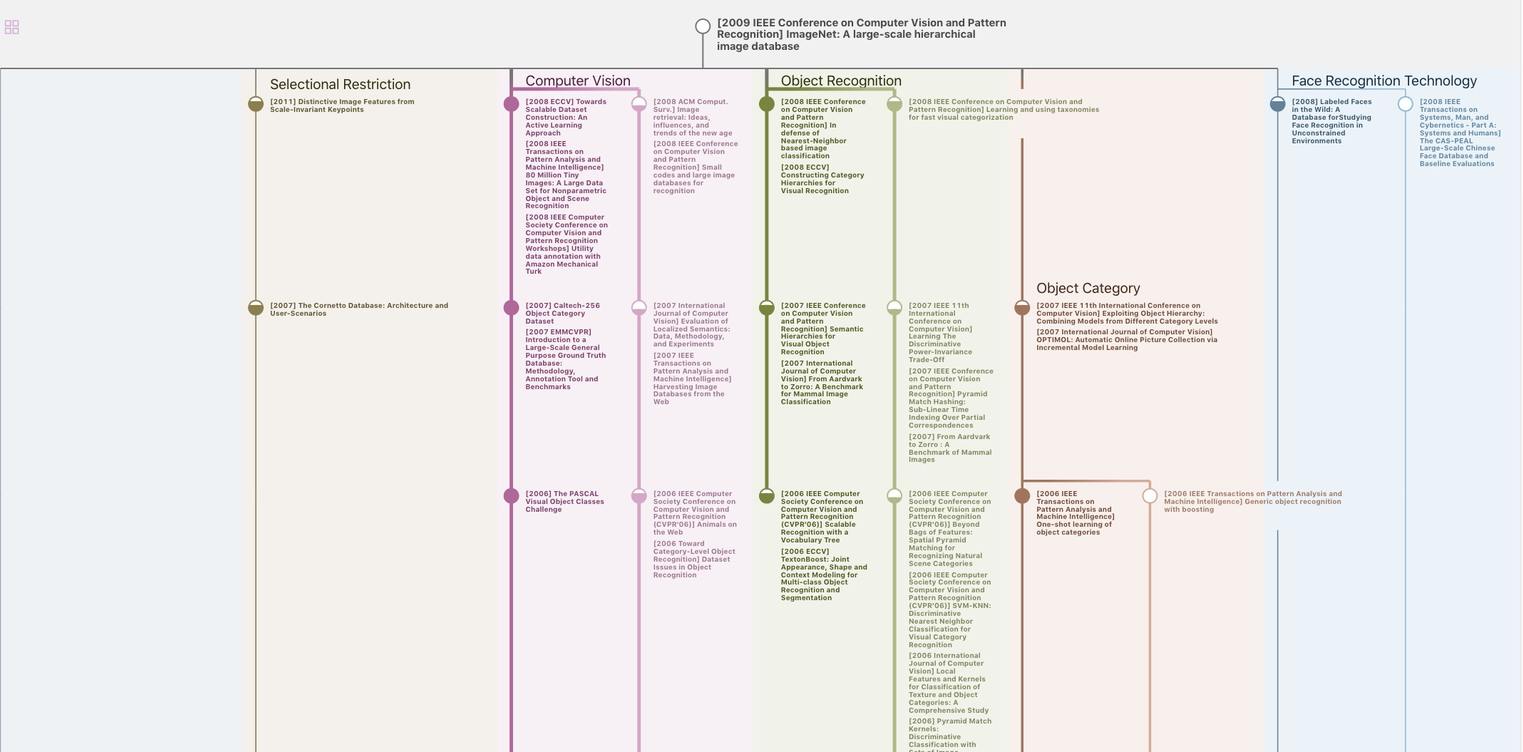
生成溯源树,研究论文发展脉络
Chat Paper
正在生成论文摘要