Measuring Dependence With Matrix-Based Entropy Functional
THIRTY-FIFTH AAAI CONFERENCE ON ARTIFICIAL INTELLIGENCE, THIRTY-THIRD CONFERENCE ON INNOVATIVE APPLICATIONS OF ARTIFICIAL INTELLIGENCE AND THE ELEVENTH SYMPOSIUM ON EDUCATIONAL ADVANCES IN ARTIFICIAL INTELLIGENCE(2021)
摘要
Measuring the dependence of data plays a central role in statistics and machine learning. In this work, we summarize and generalize the main idea of existing information-theoretic dependence measures into a higher-level perspective by the Shearer's inequality. Based on our generalization, we then propose two measures, namely the matrix-based normalized total correlation and the matrix-based normalized dual total correlation, to quantify the dependence of multiple variables in arbitrary dimensional space, without explicit estimation of the underlying data distributions. We show that our measures are differentiable and statistically more powerful than prevalent ones. We also show the impact of our measures in four different machine learning problems, namely the gene regulatory network inference, the robust machine learning under covariate shift and non-Gaussian noises, the subspace outlier detection, and the understanding of the learning dynamics of convolutional neural networks, to demonstrate their utilities, advantages, as well as implications to those problems.
更多查看译文
关键词
entropy functional,dependence,matrix-based
AI 理解论文
溯源树
样例
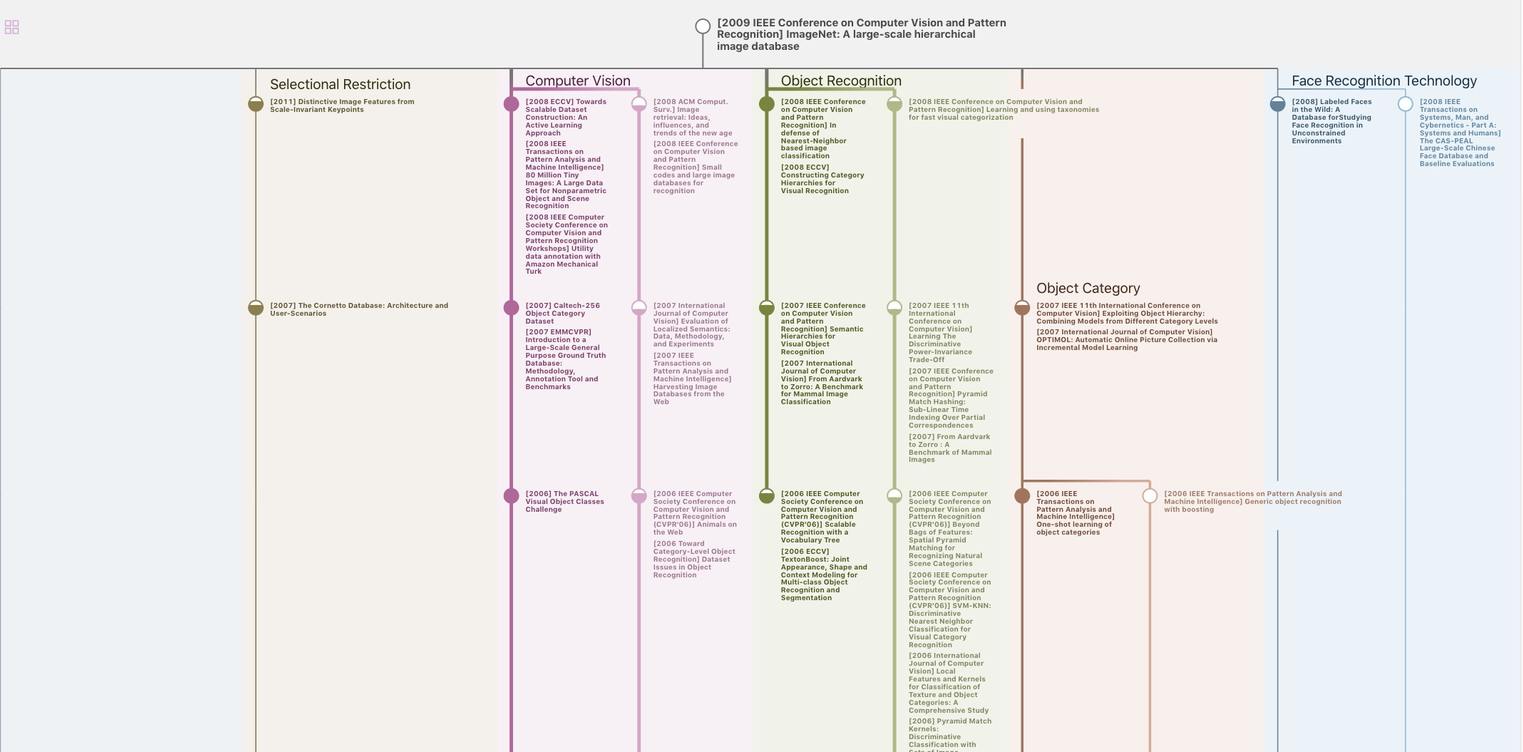
生成溯源树,研究论文发展脉络
Chat Paper
正在生成论文摘要