OpenGF: An Ultra-Large-Scale Ground Filtering Dataset Built Upon Open ALS Point Clouds Around the World
2021 IEEE/CVF CONFERENCE ON COMPUTER VISION AND PATTERN RECOGITION WORKSHOPS (CVPRW 2021)(2021)
摘要
Ground filtering has remained a widely studied but incompletely resolved bottleneck for decades in the automatic generation of high-precision digital elevation model, due to the dramatic changes of topography and the complex structures of objects. The recent breakthrough of supervised deep learning algorithms in 3D scene understanding brings new solutions for better solving such problems. However, there are few large-scale and scene-rich public datasets dedicated to ground extraction, which considerably limits the development of effective deep-learning-based ground filtering methods. To this end, we present OpenGF, first Ultra-Large-Scale Ground Filtering dataset covering over 47 km(2) of 9 different typical terrain scenes built upon open ALS point clouds of 4 different countries around the world. OpenGF contains more than half a billion finely labeled ground and non-ground points, thousands of times the number of labeled points than the de facto standard ISPRS filtertest dataset. We extensively evaluate the performance of state-of-the-art rule-based algorithms and 3D semantic segmentation networks on our dataset and provide a comprehensive analysis. The results have confirmed the capability of OpenGF to train deep learning models effectively. This dataset is released at https://github.com/Nathan-UW/OpenGF to promote more advancing research for ground filtering and large-scale 3D geographic environment understanding.
更多查看译文
关键词
als point clouds,filtering,ground,ultra-large-scale
AI 理解论文
溯源树
样例
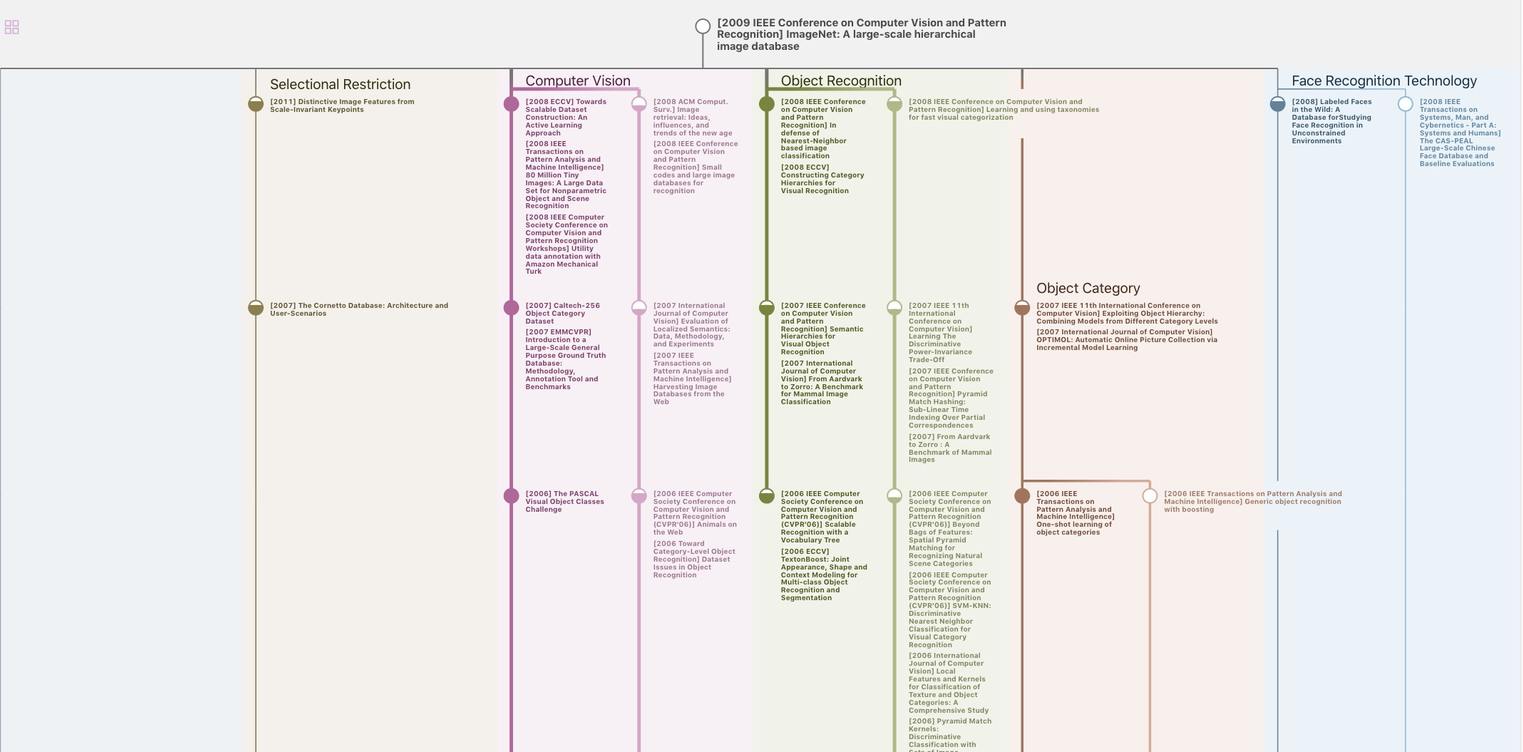
生成溯源树,研究论文发展脉络
Chat Paper
正在生成论文摘要