Automatic detection and quantification of floating marine macro-litter in aerial images: Introducing a novel deep learning approach connected to a web application in R
Environmental Pollution(2021)
摘要
The threats posed by floating marine macro-litter (FMML) of anthropogenic origin to the marine fauna, and marine ecosystems in general, are universally recognized. Dedicated monitoring programmes and mitigation measures are in place to address this issue worldwide, with the increasing support of new technologies and the automation of analytical processes. In the current study, we developed algorithms capable of detecting and quantifying FMML in aerial images, and a web-oriented application that allows users to identify FMML within images of the sea surface. The proposed algorithm is based on a deep learning approach that uses convolutional neural networks (CNNs) capable of learning from unstructured or unlabelled data. The CNN-based deep learning model was trained and tested using 3723 aerial images (50% containing FMML, 50% without FMML) taken by drones and aircraft over the waters of the NW Mediterranean Sea. The accuracies of image classification (performed using all the images for training and testing the model) and cross-validation (performed using 90% of images for training and 10% for testing) were 0.85 and 0.81, respectively. The Shiny package of R was then used to develop a user-friendly application to identify and quantify FMML within the aerial images. The implementation of this, and similar algorithms, allows streamlining substantially the detection and quantification of FMML, providing support to the monitoring and assessment of this environmental threat. However, the automated monitoring of FMML in the open sea still represents a technological challenge, and further research is needed to improve the accuracy of current algorithms.
更多查看译文
关键词
Remote sensing,Machine learning,Unmanned aerial vehicles,Convolutional neural network,Marine litter
AI 理解论文
溯源树
样例
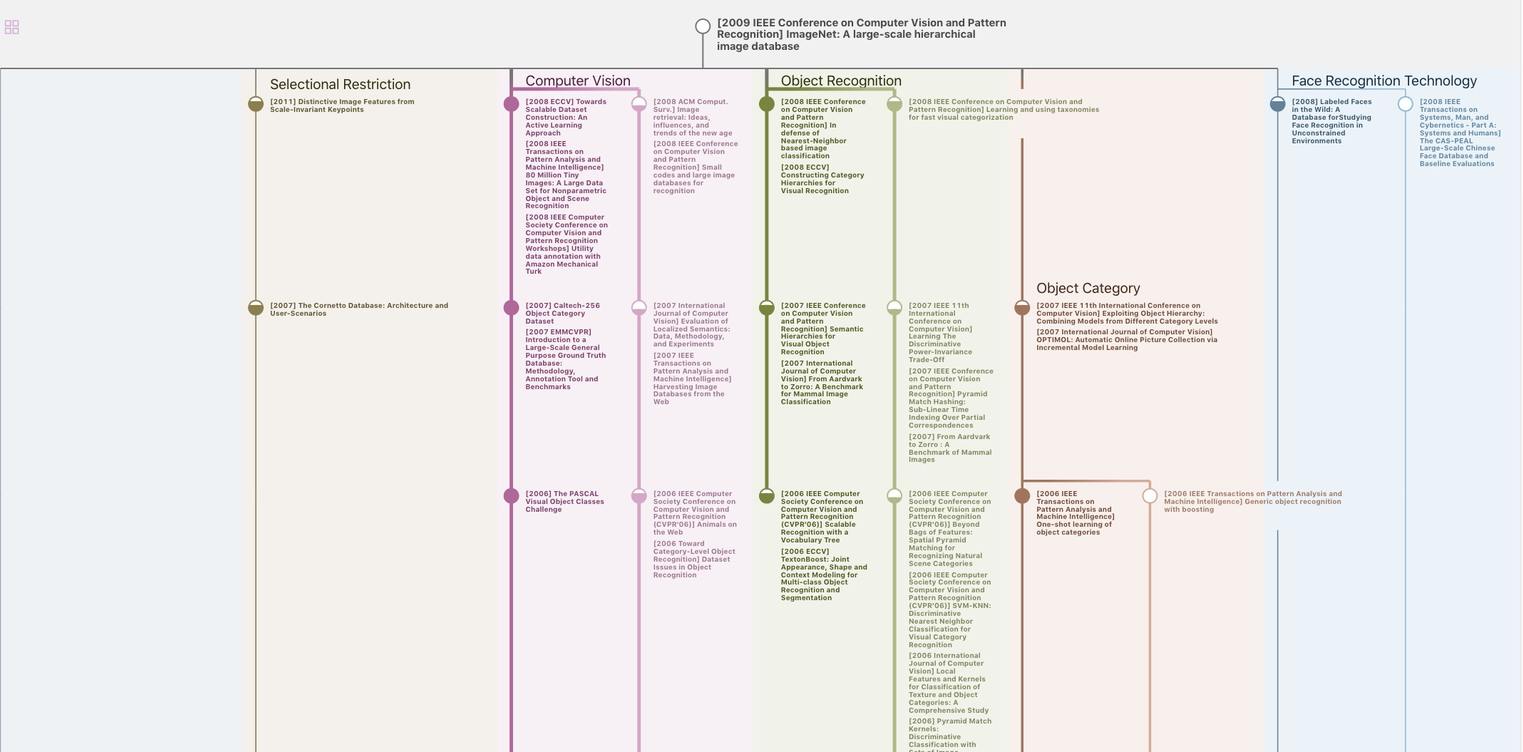
生成溯源树,研究论文发展脉络
Chat Paper
正在生成论文摘要