On Using Kernel Integration By Graphical Lasso To Study Partial Correlations Between Heterogeneous Data Sets
JOURNAL OF CHEMOMETRICS(2021)
摘要
Integration of unstructured and very diverse data is often required for a deeper understanding of the complex biological systems. In order to uncover communalities between heterogeneous data, the data are often harmonized by constructing a kernel and perform numerical integration. In this study, we propose a method for data integration in the framework of an undirected graphical model, where the nodes represent individual data sources of varying nature in terms of complexity and underlying distribution and where the edges represent the partial correlations between two blocks of data. We propose a modified GLASSO for estimation of the graph, with a combination of cross-validation and extended Bayes Information Criterion for sparsity tuning. Furthermore, hierarchical clustering on the weighted consensus kernels from a fixed network is used to partitioning the samples into different classes. Simulations show increasing ability to uncover true edges with increasing sample size and signal to noise. Likewise, identification of nonexisting edges towards disconnected nodes is feasible. The framework is demonstrated for integration of longitudinal symptom burden data, from the second and third year of life, combined with 21 diseases precursors and information of the development of asthma and eczema at the age of 6 years, from 403 children from the COPSAC2010 mother-child cohort. This suggests that maternal predisposition as well as being born preterm indirectly lead to a higher risk of asthma via an increased respiratory symptom burden.
更多查看译文
关键词
dual‐, primal optimization, GLASSO, heterogeneous data integration, kernelization, undirected graphical models
AI 理解论文
溯源树
样例
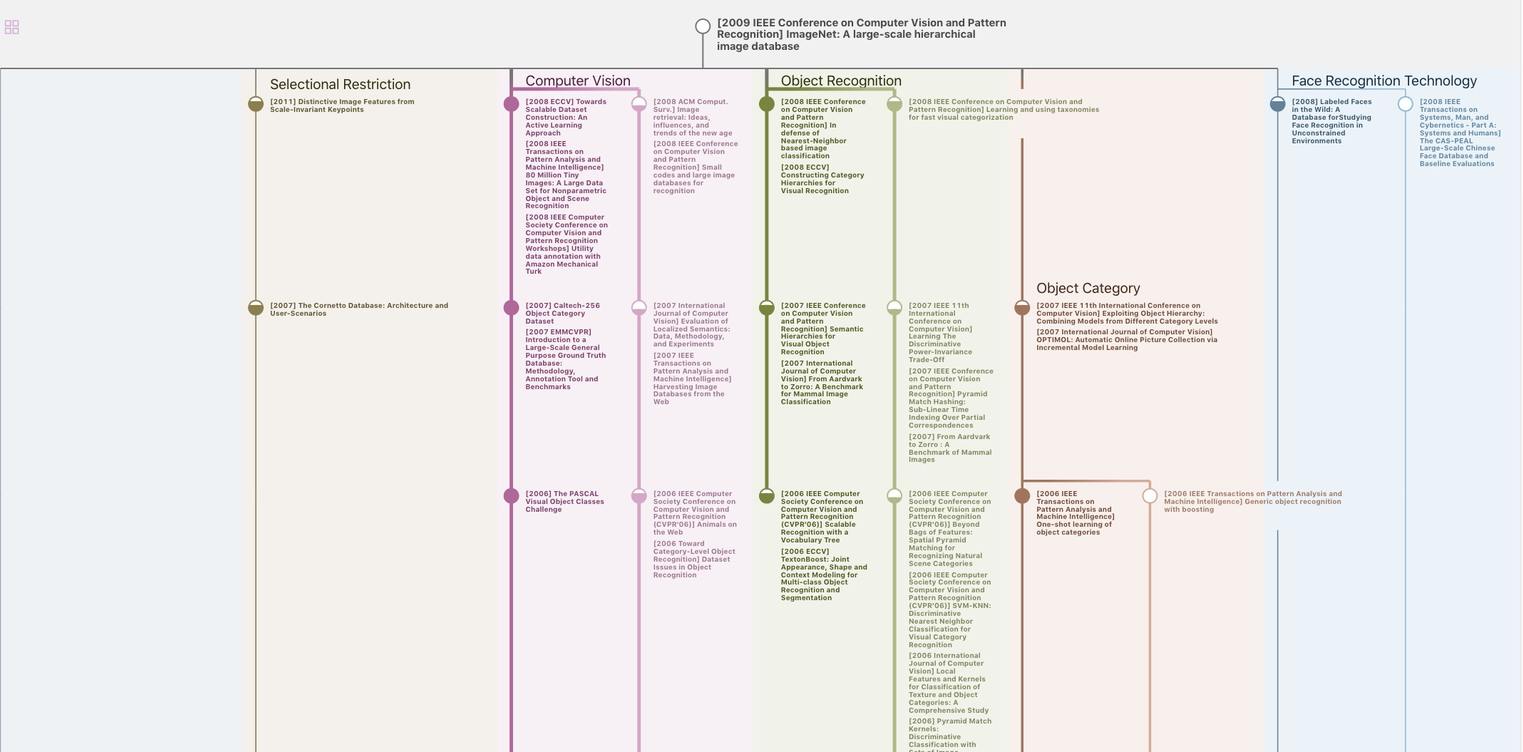
生成溯源树,研究论文发展脉络
Chat Paper
正在生成论文摘要