Multi-Resolution Representation For Streaming Time Series Retrieval
INTERNATIONAL JOURNAL OF PATTERN RECOGNITION AND ARTIFICIAL INTELLIGENCE(2021)
摘要
Streaming time series retrieval (TSR) has been widely concerned in academia and industry. Considering the large volume, high dimensionality and continuous accumulation features of time series, there is limited capability to perform in-depth similarity searching directly on the raw time series data. Therefore, time series representation, which can provide the dimension reduction-based approximate results for the raw data, should be utilized in the first step for streaming TSR. However, the existing representation-based TSR methods mainly have two limitations: on the one hand, the representation efficiency of the current methods is too slow to adapt for real-time streaming time series representation; on the other hand, the retrieval efficiency of them is also not ideal, and thus fails to recognize the specific given sequence patterns on the streaming data effectively. In this paper, we present an efficient retrieval method on streaming time series. Concretely, our method can incrementally represent the features of streaming data to automatically prune the corresponding dissimilar sequences and retain the most similar candidates for efficient one-pass searching. Extensive experiments on real world datasets have been conducted to demonstrate the superiority of our method.
更多查看译文
关键词
Streaming time series, pattern recognition, similarity retrieval, time series representation
AI 理解论文
溯源树
样例
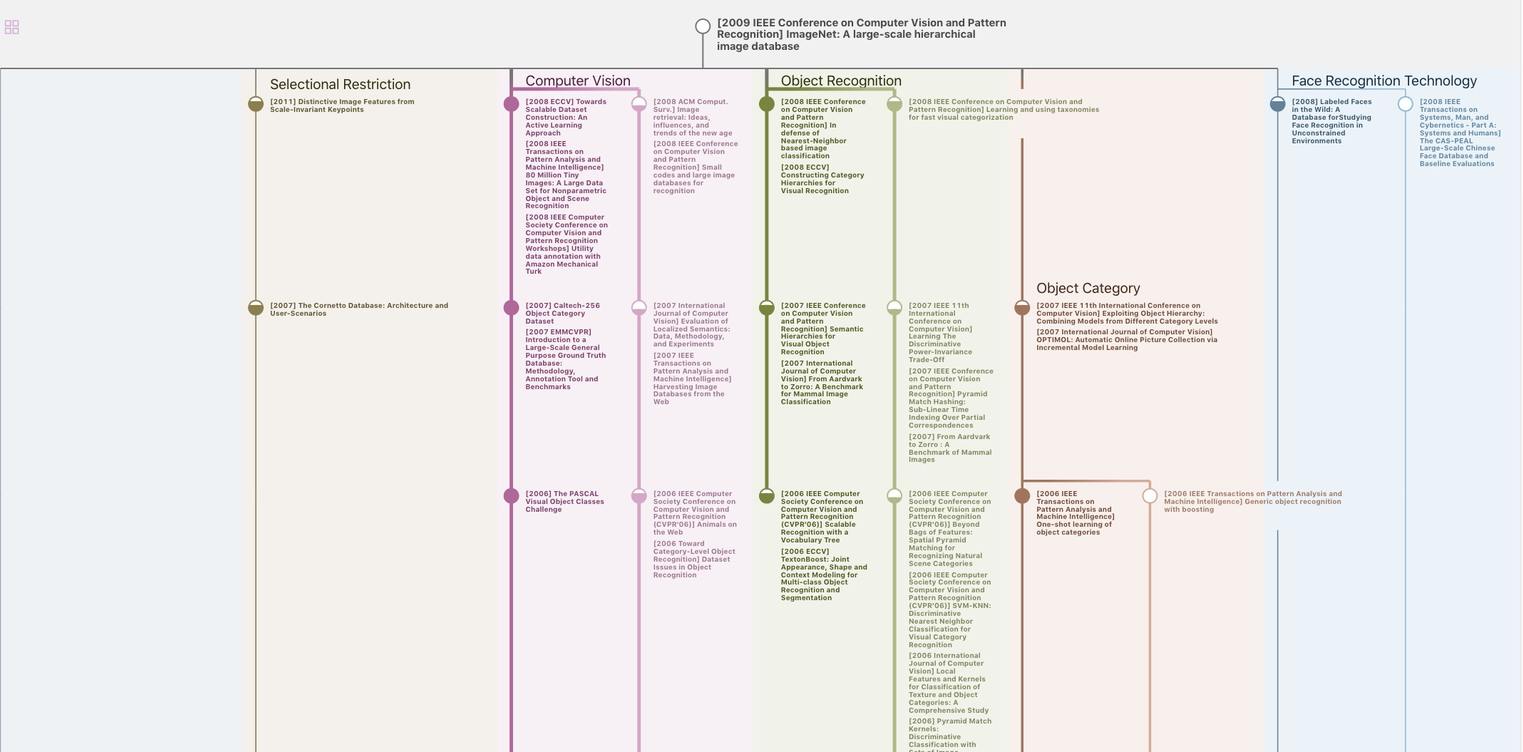
生成溯源树,研究论文发展脉络
Chat Paper
正在生成论文摘要