Self-Attentive Normalization for Automated Gleason Grading System
TENCON(2020)
摘要
Recently, convolutional neural networks (CNNs)- based automated Gleason grading system for prostate cancer has been widely researched. However, these systems still need further improvement to achieve pathologist-level performance. To this end, this paper introduces a novel self-attentive normalization (SAN) which is the first work to employ the attention mechanism for the automated Gleason grading system. Unlike conventional normalization techniques, e.g. batch normalization and instance normalization, which learn a single affine transformation, the proposed method can learn the elementwise affine transformation to focus on more informative regions of the feature map. Since SAN requires a small number of extra learning parameters, it can be integrated into existing automated Gleason grading systems seamlessly with negligible overheads. Extensive quantitative evaluations show that, by applying SAN to various CNN architectures, the diagnostic accuracy can be significantly improved. For instance, we raise VGG-16's diagnostic accuracy from 73.99% to 79.16% on the Harvard Dataverse.
更多查看译文
关键词
Automated Gleason grading system,Convolutional neural networks,Self-attentive normalization
AI 理解论文
溯源树
样例
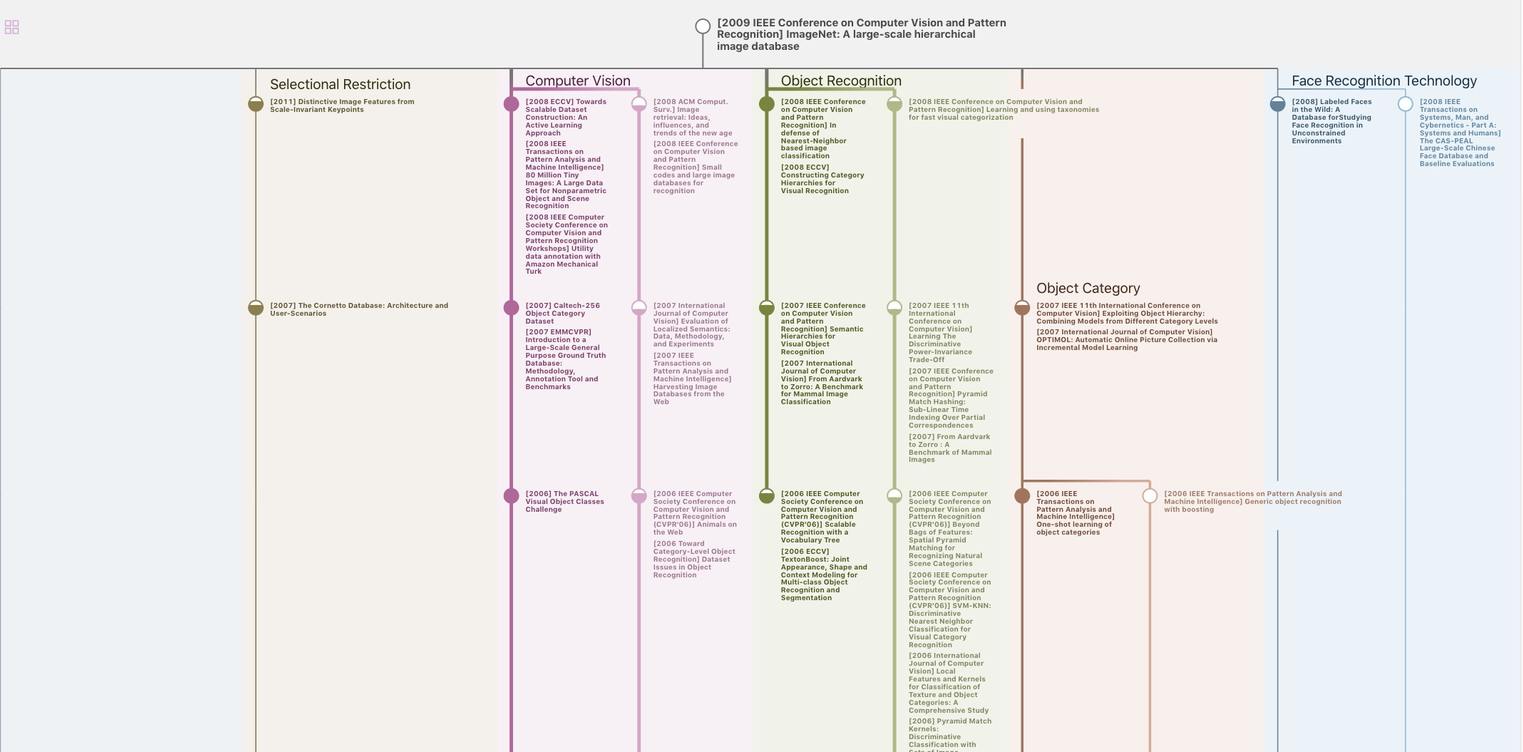
生成溯源树,研究论文发展脉络
Chat Paper
正在生成论文摘要