Dimensionality Reduction For Human Activity Recognition Using Google Colab
INFORMATION(2021)
摘要
Human activity recognition (HAR) is a classification task that involves predicting the movement of a person based on sensor data. As we can see, there has been a huge growth and development of smartphones over the last 10-15 years-they could be used as a medium of mobile sensing to recognize human activity. Nowadays, deep learning methods are in a great demand and we could use those methods to recognize human activity. A great way is to build a convolutional neural network (CNN). HAR using Smartphone dataset has been widely used by researchers to develop machine learning models to recognize human activity. The dataset has two parts: training and testing. In this paper, we propose a hybrid approach to analyze and recognize human activity on the same dataset using deep learning method on cloud-based platform. We have applied principal component analysis on the dataset to get the most important features. Next, we have executed the experiment for all the features as well as the top 48, 92, 138, and 164 features. We have run all the experiments on Google Colab. In the experiment, for the evaluation of our proposed methodology, datasets are split into two different ratios such as 70-10-20% and 80-10-10% for training, validation, and testing, respectively. We have set the performance of CNN (70% training-10% validation-20% testing) with 48 features as a benchmark for our work. In this work, we have achieved maximum accuracy of 98.70% with CNN. On the other hand, we have obtained 96.36% accuracy with the top 92 features of the dataset. We can see from the experimental results that if we could select the features properly then not only could the accuracy be improved but also the training and testing time of the model.
更多查看译文
关键词
cloud platform, convolutional neural network (CNN), deep learning, dimensionality reduction, feature extraction, google colab, human activity recognition (HAR), principal component analysis (PCA), smartphone
AI 理解论文
溯源树
样例
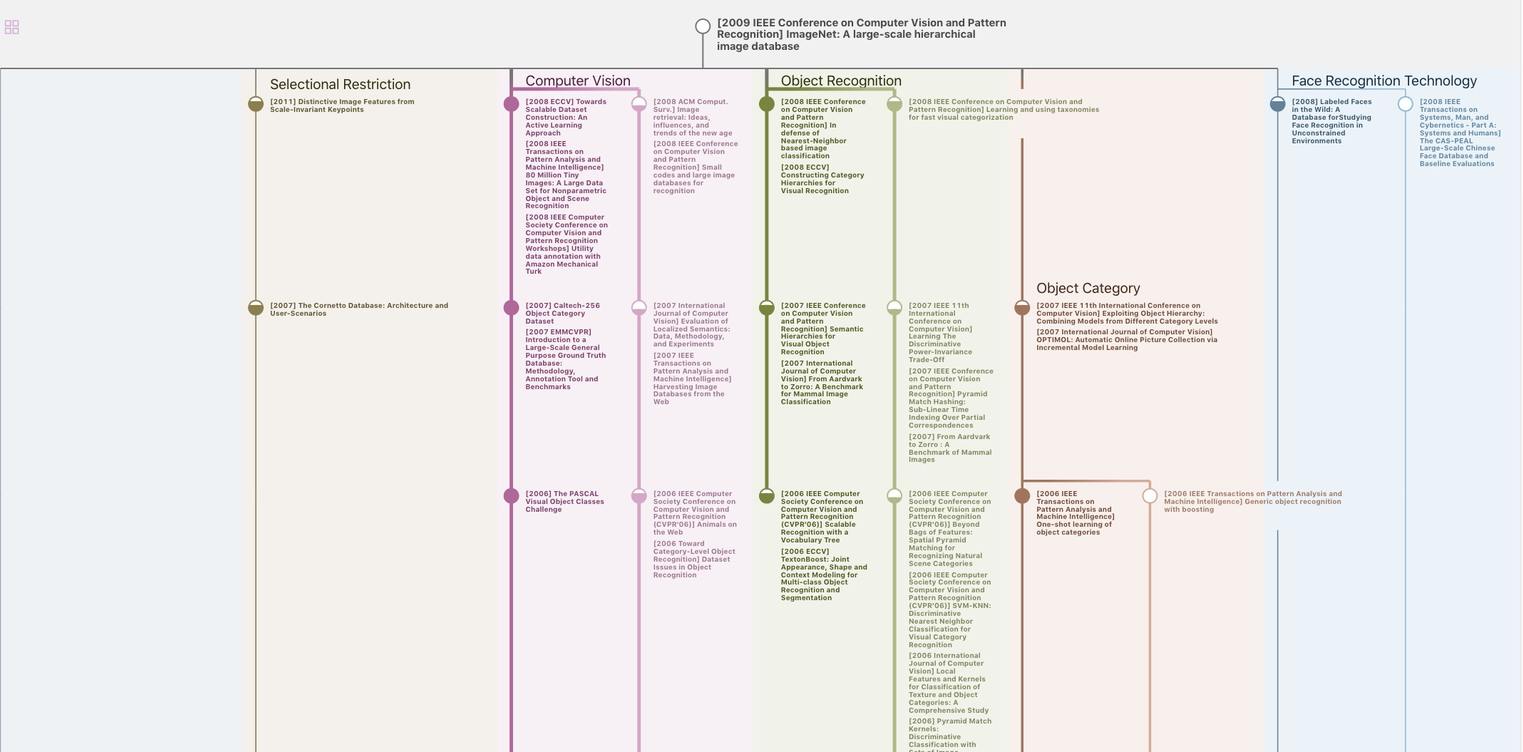
生成溯源树,研究论文发展脉络
Chat Paper
正在生成论文摘要