Towards Scalable Spectral Embedding and Data Visualization via Spectral Coarsening
WSDM(2021)
摘要
ABSTRACTThis paper proposes a scalable multilevel framework for the spectral embedding of large undirected graphs. The proposed method first computes much smaller yet sparse graphs while preserving the key spectral (structural) properties of the original graph, by exploiting a nearly-linear time spectral graph coarsening approach. Then, the resultant spectrally-coarsened graphs are leveraged for the development of much faster algorithms for multilevel spectral graph embedding (clustering) as well as visualization of large data sets. We conducted extensive experiments using a variety of large graphs and datasets and obtained very promising results. For instance, we are able to coarsen the "coPapersCiteseer" graph with 0.43 million nodes and 16 million edges into a much smaller graph with only 13K (32X fewer) nodes and 17K (950X fewer) edges in about 16 seconds; the spectrally-coarsened graphs allow us to achieve up to 1,100X speedup for multilevel spectral graph embedding (clustering) and up to 60X speedup for t-SNE visualization of large data sets.
更多查看译文
关键词
Spectral graph theory,spectral embedding,graph clustering,data visualization
AI 理解论文
溯源树
样例
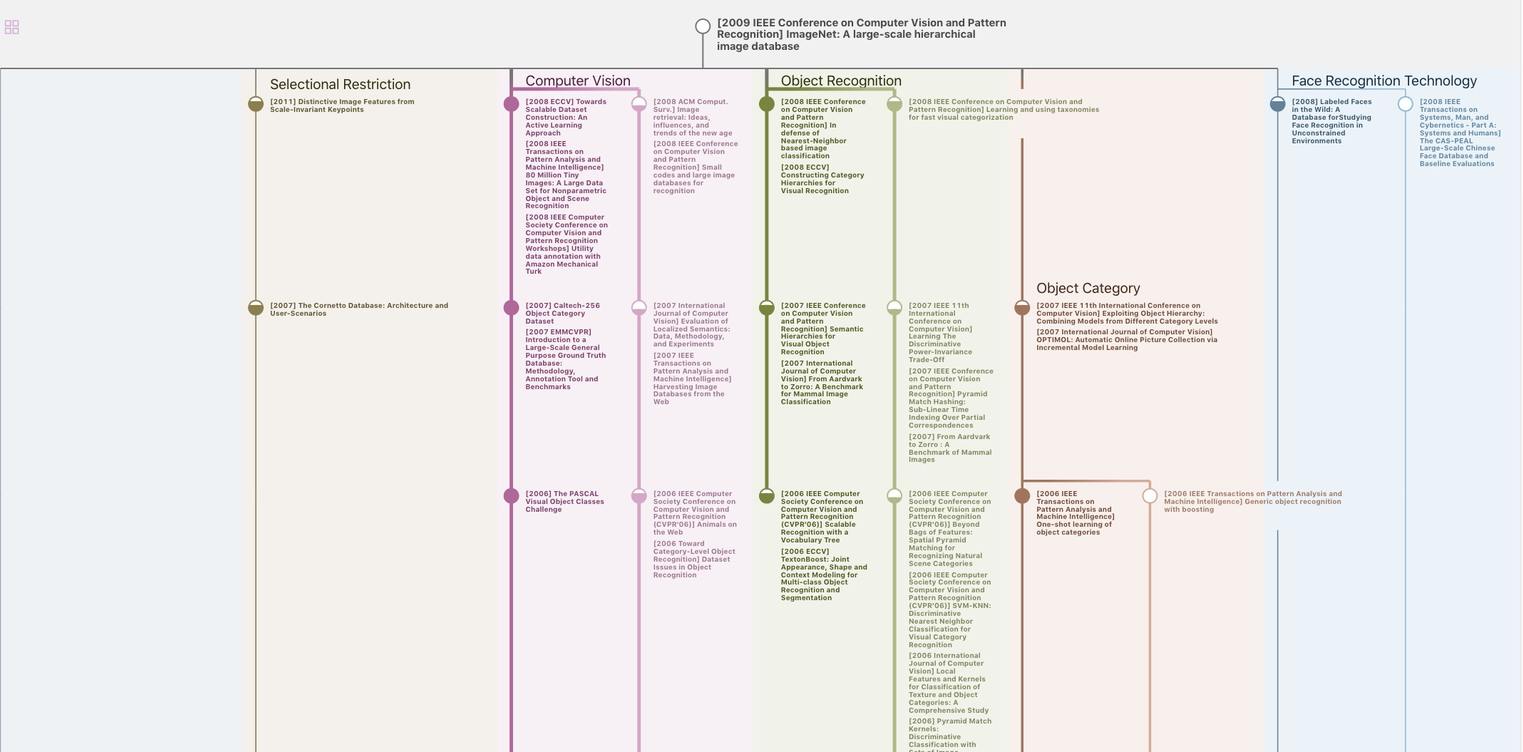
生成溯源树,研究论文发展脉络
Chat Paper
正在生成论文摘要