Deep Reinforcement Learning Supervised Autonomous Exploration In Office Environments
2018 IEEE INTERNATIONAL CONFERENCE ON ROBOTICS AND AUTOMATION (ICRA)(2018)
摘要
Exploration region selection is an essential decision making process in autonomous robot exploration task. While a majority of greedy methods are proposed to deal with this problem, few efforts are made to investigate the importance of predicting long-term planning. In this paper, we present an algorithm that utilizes deep reinforcement learning (DRL) to learn exploration knowledge over office blueprints, which enables the agent to predict a long-term visiting order for unexplored subregions. On the basis of this algorithm, we propose an exploration architecture that integrates a DRL model, a nextbest- view (NBV) selection approach and a structural integrity measurement to further improve the exploration performance. At the end of this paper, we evaluate the proposed architecture against other methods on several new office maps, showing that the agent can efficiently explore uncertain regions with a shorter path and smarter behaviors.
更多查看译文
关键词
supervised autonomous exploration,office environments,exploration region selection,autonomous robot exploration task,greedy methods,long-term planning,deep reinforcement learning,exploration knowledge,office blueprints,DRL model,next-best-view selection approach,structural integrity measurement,office maps,decision making process
AI 理解论文
溯源树
样例
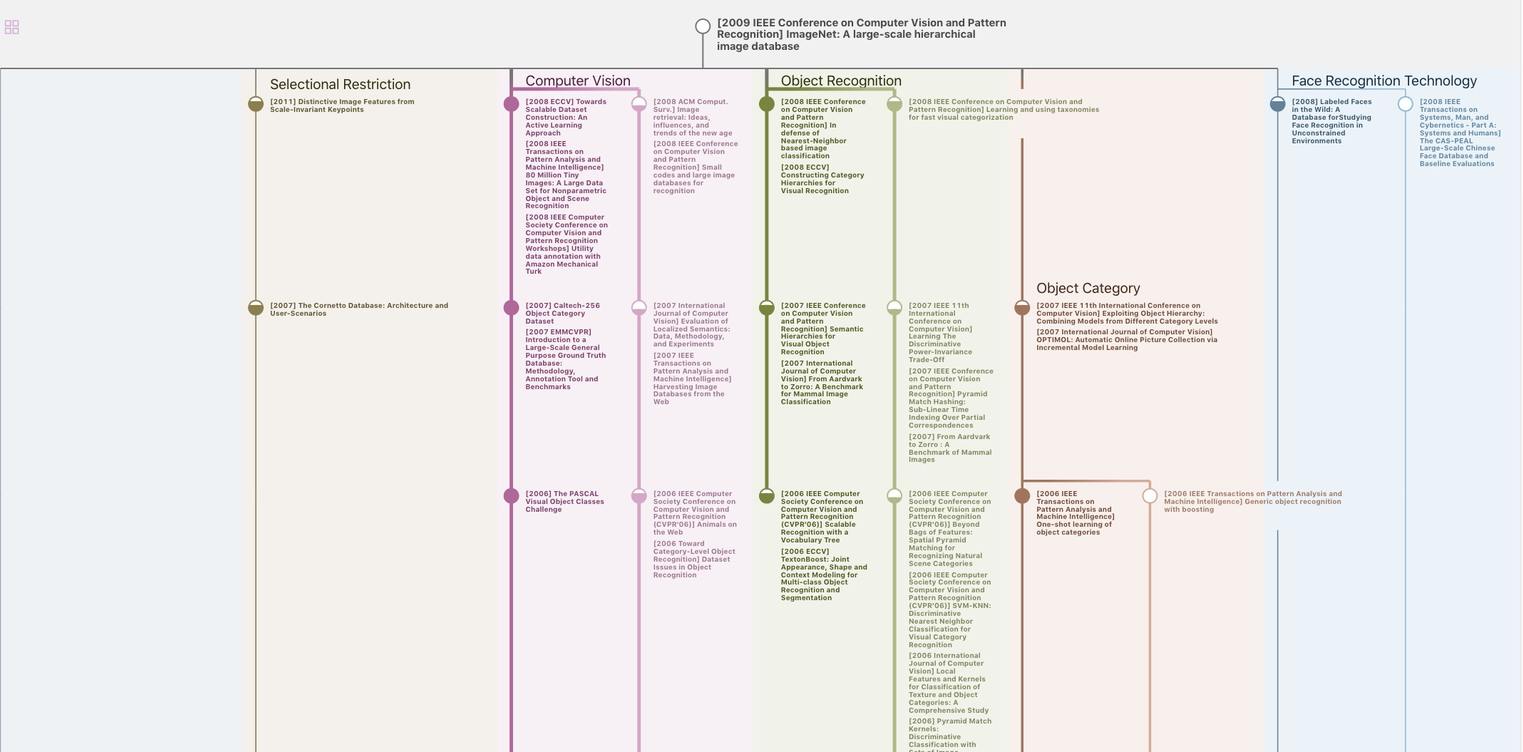
生成溯源树,研究论文发展脉络
Chat Paper
正在生成论文摘要