Unsupervised Change Detection Using Multiscale And Multiresolution Gaussian-Mixture-Model Guided By Saliency Enhancement
IEEE JOURNAL OF SELECTED TOPICS IN APPLIED EARTH OBSERVATIONS AND REMOTE SENSING(2021)
摘要
Popular unsupervised change detection algorithms suffer from two problems: first, the difference image generated by bitemporal images usually includes a large number of falsely changed regions due to noise corruption and illumination change; second, fuzzy clustering algorithms are sensitive to noise and they miss the relationship among feature components. To address these issues, we propose a multiscale and multiresolution Gaussian-mixture-model guided by saliency-enhancement (SE-MGMM) for change detection in bitemporal remote sensing images. The proposed SE-MGMM makes two contributions. The first is a novel salient strategy that can enhance saliency objects while suppressing the image background. The strategy uses the saliency weight information to enhance changed regions leading to the improvement of grayscale contrast between changed regions and unchanged regions. The second is that we present a Gaussian-mixture-model based on spatial multiscale and frequency multiresolution information fusion, which can effectively utilize features of difference images and improve detection results of changed regions. Experiments show that the proposed SE-MGMM is robust for both very high-resolution remote sensing images and synthetic aperture radar images. Moreover, the SE-MGMM achieves better change detection and provides better performance metrics than state-of-the-art approaches.
更多查看译文
关键词
Task analysis, Feature extraction, Lighting, Level set, Gray-scale, Change detection algorithms, Satellites, Change detection, feature fusion, Gaussian-mixture-model (GMM), saliency enhancement
AI 理解论文
溯源树
样例
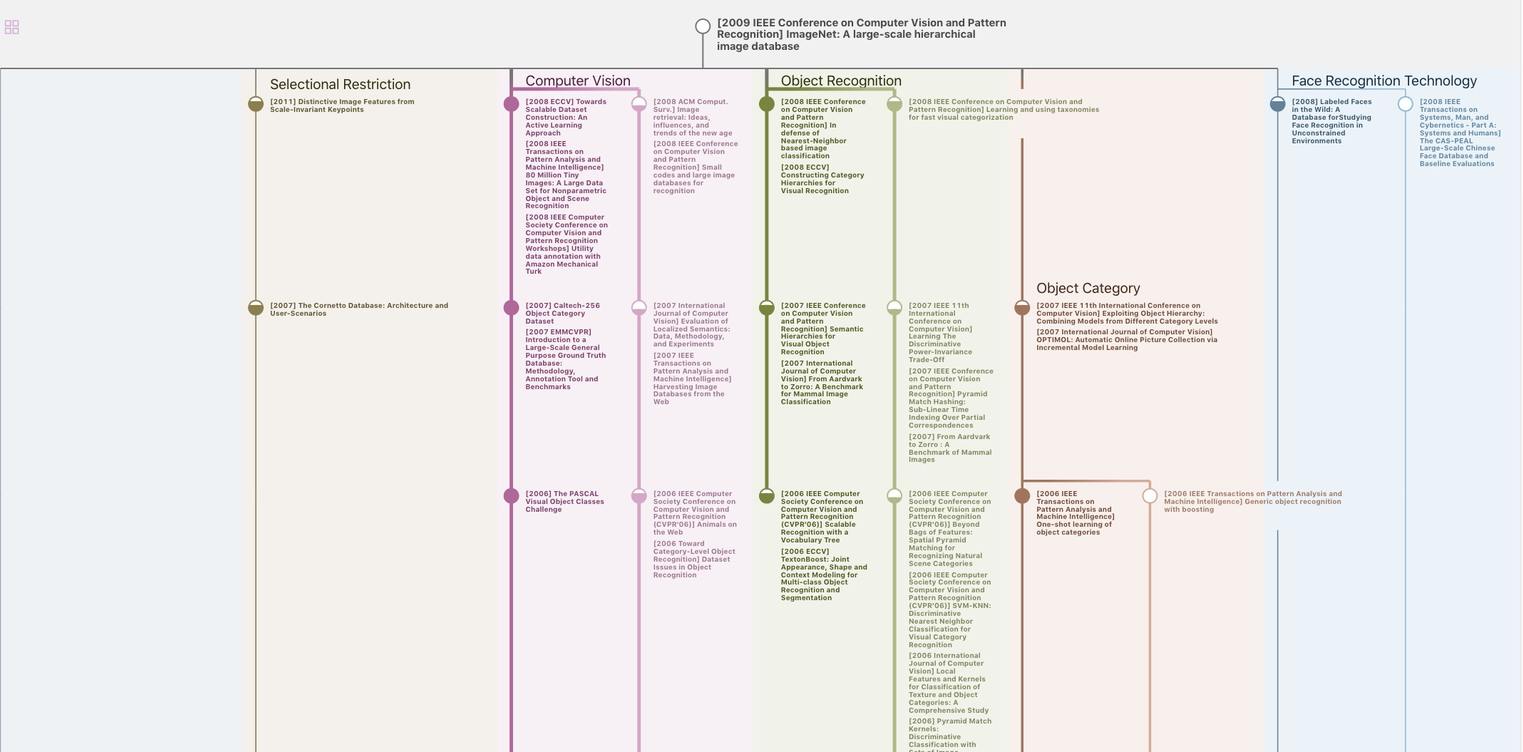
生成溯源树,研究论文发展脉络
Chat Paper
正在生成论文摘要