Knowledge-based Deep Reinforcement Learning for Train Automatic Stop Control of High-Speed Railway
international conference on machine learning(2020)
摘要
Train automatic stop control (TASC) is one of the key techniques of Automatic train operation (ATO) to achieve high stopping precision. Aiming to improve accurate stopping performance, this paper proposes a novel TASC method based on double deep Q-network (DDQN) using knowledge from experienced driver to address time allocation of braking command. The knowledge is used for estimating a braking command to improve the learning efficiency, and DDQN determines the execution time of the command to avoid frequent switching of commands and ultimately reach better stopping decisions. The proposed method can achieve a probability of 100% and significantly outperforms 3 existing methods on the stopping errors within ± 0.30 m under high disturbances in the simulation platform, which is based on actual field data from the Beijing-Shenyang high-speed railway provided by cooperative enterprise.
更多查看译文
AI 理解论文
溯源树
样例
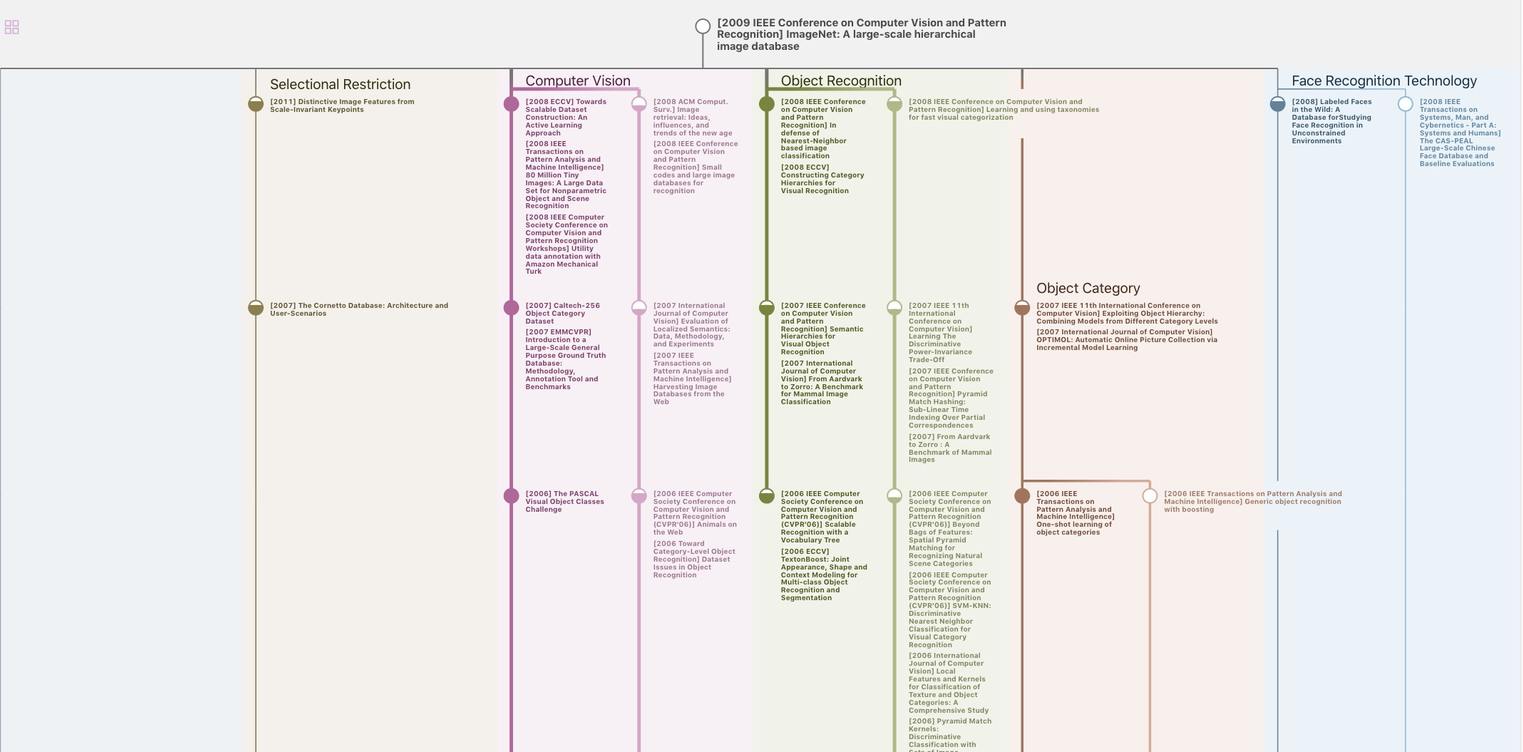
生成溯源树,研究论文发展脉络
Chat Paper
正在生成论文摘要