Seismic Geometric Nonparallelism Attributes
IEEE GEOSCIENCE AND REMOTE SENSING LETTERS(2022)
摘要
Pattern recognition based on computer-assisted seismic classification accelerates traditional seismic interpretation and can automatically extract seismic patterns of interest on large data volumes that would take considerable effort by a human interpreter. Seismic facies such as salt domes, mass transport complexes, and karst collapse features, can often be differentiated from surrounding conformal sediments by lateral and vertical changes in dip, energy, and continuity. To better exploit the difference between conformal and more chaotic seismic features, we introduce 3-D geometric nonparallelism attributes to statistically quantify lateral and vertical changes in both dip and amplitude gradients. When examining the attribute response of salt and karst features, coherent noise or small internal reflector blocks in an otherwise chaotic background give rise to a x201C;salt and pepperx201D; pattern on coherence, reflector parallelism, and texture attributes. Whereas human interpreters see the larger patterns and can put them in the proper geological context, simple attribute classification techniques cannot and see such embedded coherent anomalies as separate facies. To address this issue, we apply a 3-D structure-oriented adaptive Kuwahara filtering technique to precondition the seismic attribute volumes to smooth the internal attribute response while sharpening the facies edges prior to subsequent machine learning classification. We validate our workflow by applying a semisupervised generative topographic mapping machine learning-based multiattribute classification algorithm of five seismic attribute volumes to mapping karst collapse facies.
更多查看译文
关键词
Seismic measurements, Geologic measurements, Covariance matrices, Standards, Eigenvalues and eigenfunctions, Weight measurement, Wavelength measurement, Pattern recognition, seismic attribute, seismic facies analysis
AI 理解论文
溯源树
样例
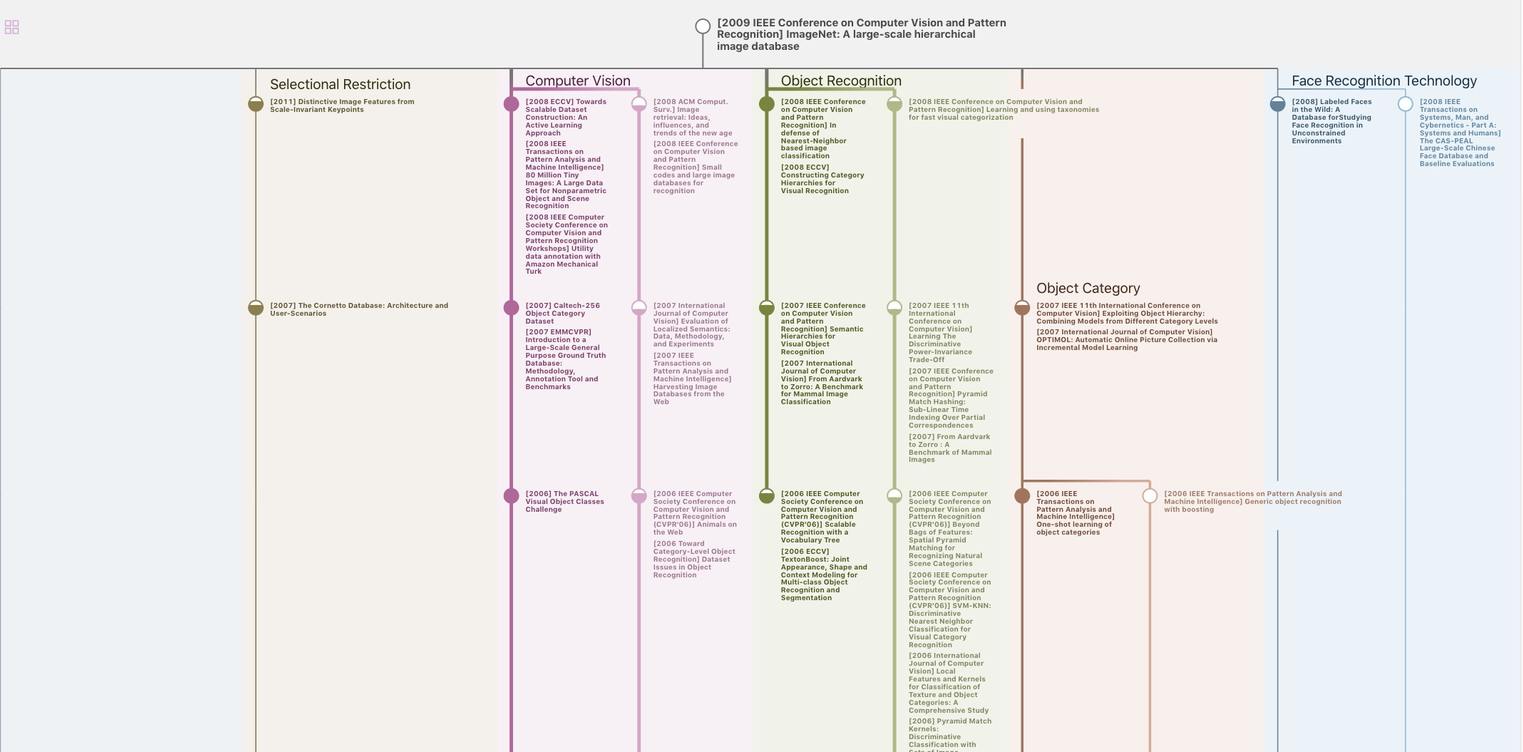
生成溯源树,研究论文发展脉络
Chat Paper
正在生成论文摘要