Comparison of multispectral modeling of physiochemical attributes of greengage: Brix and pH values
FOOD SCIENCE AND TECHNOLOGY(2021)
摘要
Chemometric modeling concerns both accuracy and computational expense for the prediction of quality-indicating attributes of food materials. Modeling approaches were explored with the hyperspectral images with pH and Brix values of greengages. A two-phase architecture was applied for modeling. Firstly, waveband selection was performed using two approaches, i.e., succession projection algorithm (SPA) and its combination with genetic algorithm (SPA+GA). Secondly, multispectral models based on the two feature sets of wavebands were built via a total of six different modeling methods, i.e., partial least squares regression (PLSR) and extreme learning machine (ELM) in their respective stand-alone versions, their applications combined with genetic algorithm (GA), and their ensemble enhancements with modified Adaboost.RT (MAdaboost.RT). Analysis of accuracy and computational expense showed that supervised feature selection with SPA+GA was superior to unsupervised SPA for better modeling accuracy. MAdaboost.RT-ELM showed high accuracy at low computational expense. ELM models were the better base models than the PLSR ones, for being more randomized and diverse. It indicates that MAdaboost.RT-ELM on SPA is the best choice for a quick test on a newly available dataset, while switching the dimensionality reduction from SPA to SPA+GA may yield more accurate models with added, but well worthy, computational expense.
更多查看译文
关键词
multispectral modeling, supervised feature wavelength selection, modified Adaboost, RT, extreme learning machine, greengage, Brix, pH
AI 理解论文
溯源树
样例
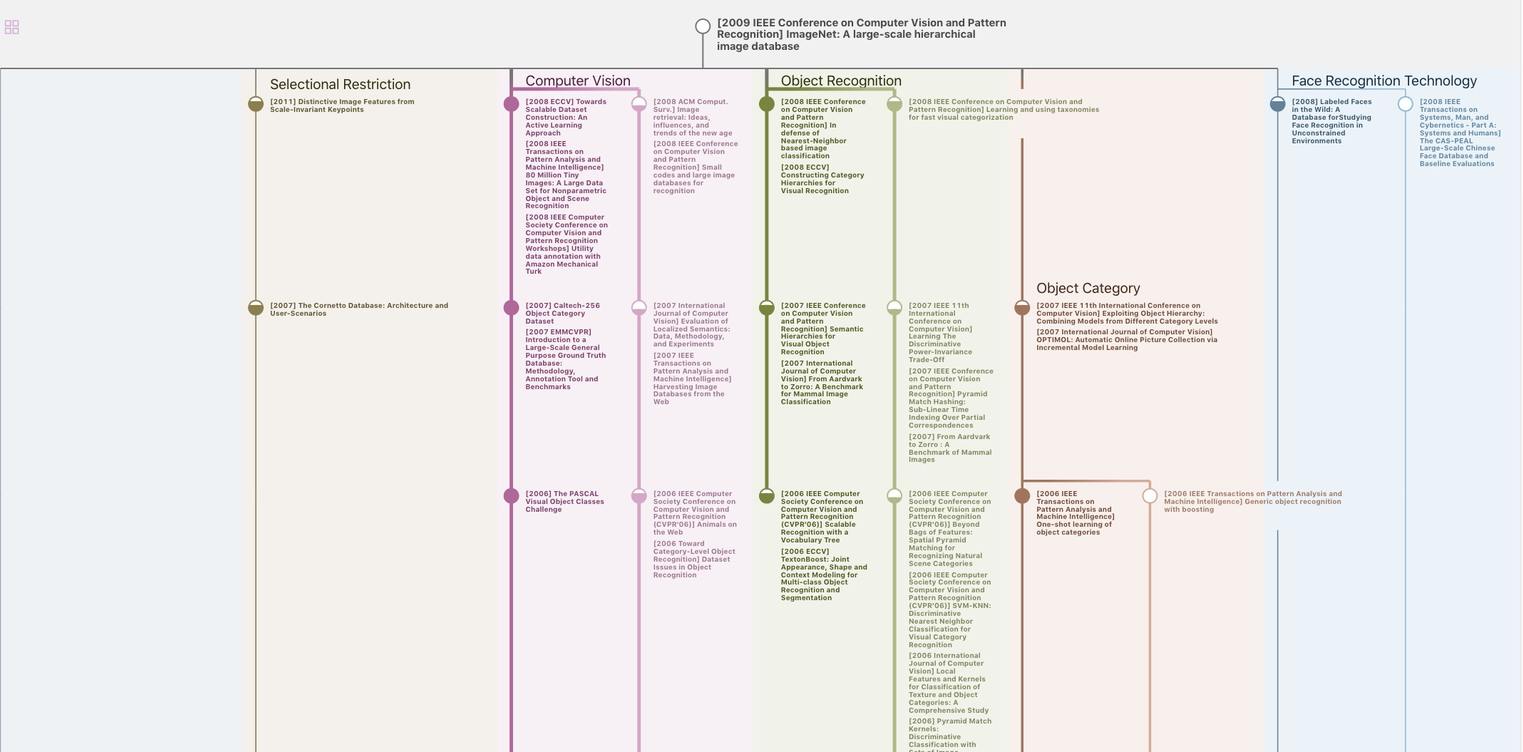
生成溯源树,研究论文发展脉络
Chat Paper
正在生成论文摘要