Deep Reinforcement Learning (DRL) for Portfolio Allocation
MACHINE LEARNING AND KNOWLEDGE DISCOVERY IN DATABASES: APPLIED DATA SCIENCE AND DEMO TRACK, ECML PKDD 2020, PT V(2021)
摘要
Deep reinforcement learning (DRL) has reached an unprecedent level on complex tasks like game solving (Go [6], StarCraft II [7]), and autonomous driving. However, applications to real financial assets are still largely unexplored and it remains an open question whether DRL can reach super human level. In this demo, we showcase state-of-the-art DRL methods for selecting portfolios according to financial environment, with a final network concatenating three individual networks using layers of convolutions to reduce network's complexity. The multi entries of our network enables capturing dependencies from common financial indicators features like risk aversion, citigroup index surprise, portfolio specific features and previous portfolio allocations. Results on test set show this approach can overperform traditional portfolio optimization methods with results available at our demo website.
更多查看译文
关键词
Deep reinforcement learning, Portfolio selection, Convolutional networks, Index surprise, Risk aversion
AI 理解论文
溯源树
样例
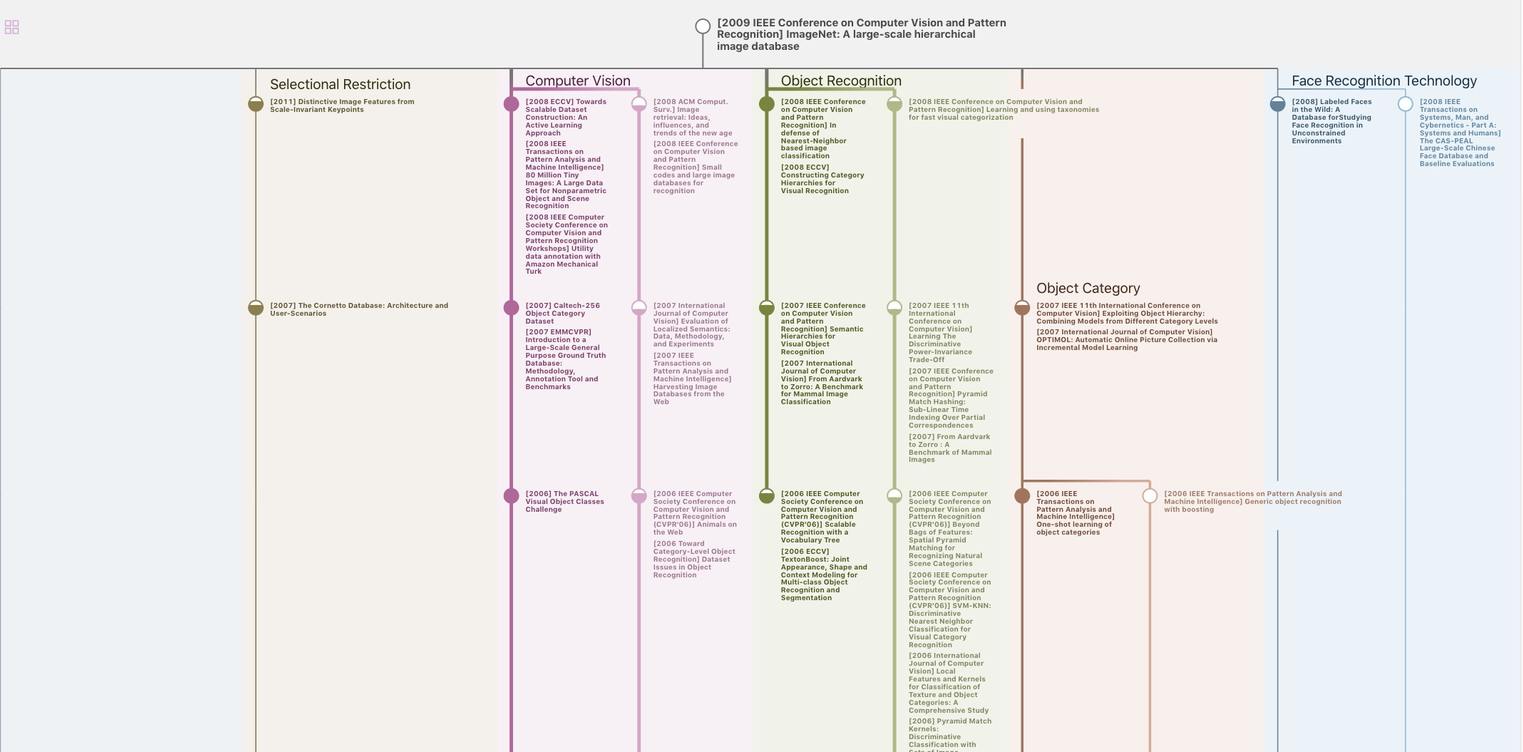
生成溯源树,研究论文发展脉络
Chat Paper
正在生成论文摘要